Scattering-based hybrid network for facial attribute classification
Frontiers of Computer Science: Selected Publications from Chinese Universities(2023)
摘要
Face attribute classification (FAC) is a high-profile problem in biometric verification and face retrieval. Although recent research has been devoted to extracting more delicate image attribute features and exploiting the inter-attribute correlations, significant challenges still remain. Wavelet scattering transform (WST) is a promising non-learned feature extractor. It has been shown to yield more discriminative representations and outperforms the learned representations in certain tasks. Applied to the image classification task, WST can enhance subtle image texture information and create local deformation stability. This paper designs a scattering-based hybrid block, to incorporate frequency-domain (WST) and image-domain features in a channel attention manner (Squeeze-and-Excitation, SE), termed WS-SE block. Compared with CNN, WS-SE achieves a more efficient FAC performance and compensates for the model sensitivity of the small-scale affine transform. In addition, to further exploit the relationships among the attribute labels, we propose a learning strategy from a causal view. The cause attributes defined using the causality-related information can be utilized to infer the effect attributes with a high confidence level. Ablative analysis experiments demonstrate the effectiveness of our model, and our hybrid model obtains state-of-the-art results in two public datasets.
更多查看译文
关键词
wavelet scattering transform,causality-related learning,facial attribute classification
AI 理解论文
溯源树
样例
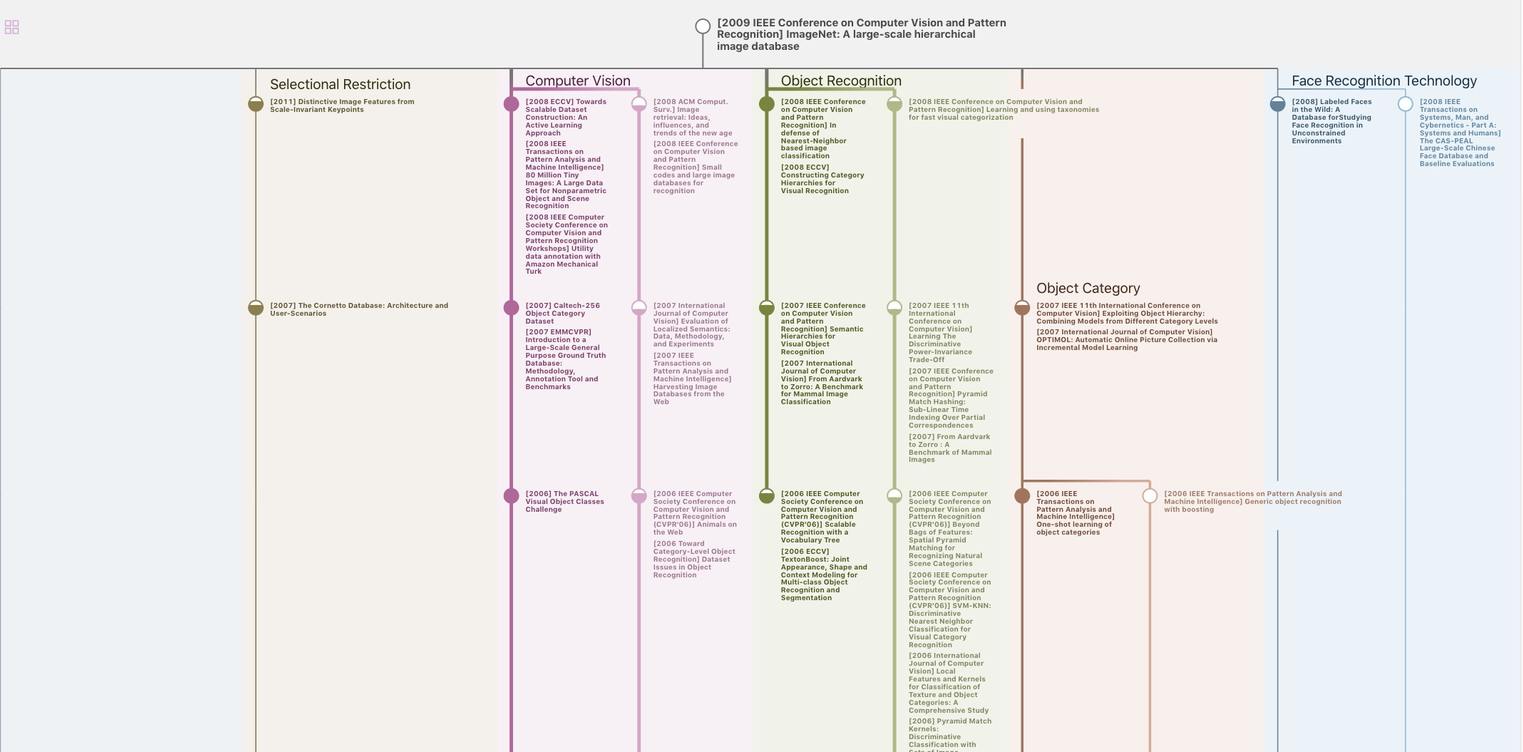
生成溯源树,研究论文发展脉络
Chat Paper
正在生成论文摘要