Study on remote sensing inversion and temporal-spatial variation of Hulun lake water quality based on machine learning
JOURNAL OF CONTAMINANT HYDROLOGY(2024)
摘要
Hulun Lake is facing significant water quality degradation, necessitating effective monitoring for safety. Traditional methods lack the necessary spatial and temporal coverage, underscoring the need for a remote sensing model. In this study, we utilized the Landsat 8 OLI dataset, incorporating cross-section monitoring and field sampling data comprehensively. Employing the random forest algorithm, we constructed a remote sensing inversion model for six water quality parameters in Hulun Lake: chlorophyll-a (Chl-a), total nitrogen (TN), total phosphorus (TP), ammonia nitrogen (NH3- N), chemical oxygen demand (COD), and dissolved oxygen (DO). The model was applied to the non-freezing period of Hulun Lake from 2016 to 2021, exhibiting commendable performance and generating high-resolution maps. Time series analysis revealed that during the study period, the pollution levels of TN, TP, and COD in Hulun Lake were extremely serious, exceeding the Class V water standard of China's surface water environmental quality standard. Regional analysis indicated lower pollutant concentrations in the central lake area compared to the lake inlet. The inflowing rivers with high pollution adversely impacted Hulun Lake's water quality. To ensure the continued health of Hulun Lake's water quality, it is imperative to monitor lake water quality attentively and implement necessary measures to prevent further deterioration. This study holds crucial importance for shaping and executing ecological protection and restoration strategies for Hulun Lake.
更多查看译文
关键词
Random Forest,Landsat 8 OLI,Water quality retrieval,Hulun Lake,Temporal-spatial dynamics
AI 理解论文
溯源树
样例
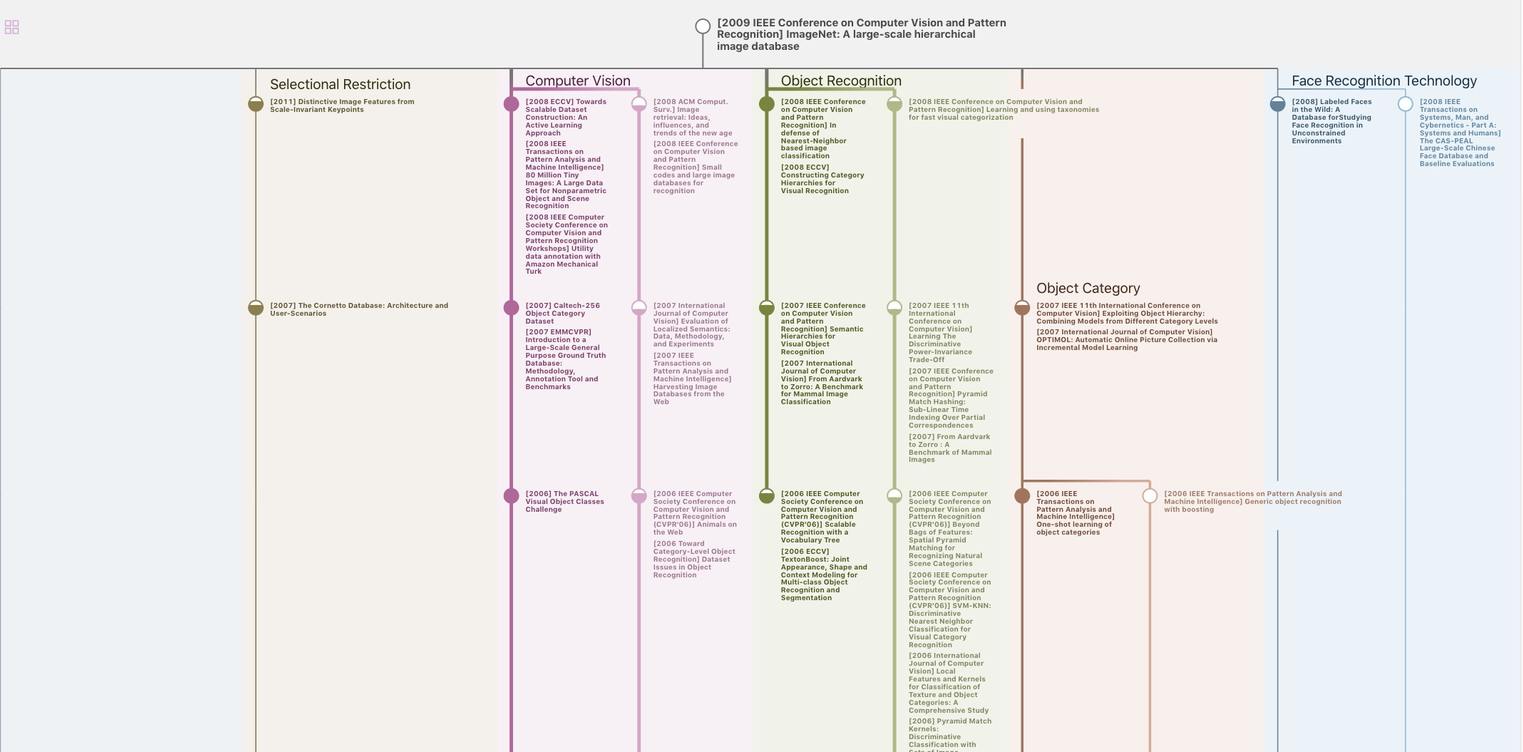
生成溯源树,研究论文发展脉络
Chat Paper
正在生成论文摘要