RNN-based CO2 minimum miscibility pressure (MMP) estimation for EOR and CCUS applications
FUEL(2024)
摘要
Accurate estimation of minimum miscibility pressure (MMP) is crucial for assessing the efficiency of most miscible and immiscible processes, specifically CO2-based enhanced oil recovery (EOR) methods and Carbon capture utilization and sequestration (CCUS). The experimental procedure for MMP prediction is often timeconsuming and costly. On the other hand, the empirical models that have been historically used could work based on limited input parameters, ignore the importance of others and are not necessarily accurate. The novelty of the current study is using an explainable deep-learning approach, Recurrent neural network (RNN), to train a model using a multi-dimensional (22 features) dataset with 544 rows of data. The Dataset comprises mole fractions of injected gas (pure and impure CO2). Out of those features, eight subsets of parameters (labelled X_1 to X_8) were used to develop models. The multi-dimensionality of the dataset makes it suitable to study the effects of various parameters on MMP, specifically in conditions of interest to CCUS-EOR applications. Among the multiple inputs tested, the model trained with X_1 and X_8 input parameters (including mole fraction of different hydrocarbon and nonhydrocarbon components and reservoir temperature) resulted in the most accurate estimations of MMP (R2 = 0.99). To further enhance the explainability of the model, feature importance and shapely values analysis were conducted on the developed models, and the impact of each input feature on MMP was elaborated. Temperature, volatile/intermediate, and nonhydrocarbon components are the most influential parameters depending on the subset of parameters chosen. Moreover, the developed model using X_8 inputs performed significantly better (37 % more accurately) than three well-known empirical models from the literature.
更多查看译文
关键词
Minimum miscibility pressure (MMP),Deep learning,Recurrent neural networks (RNN),CCUS,EOR
AI 理解论文
溯源树
样例
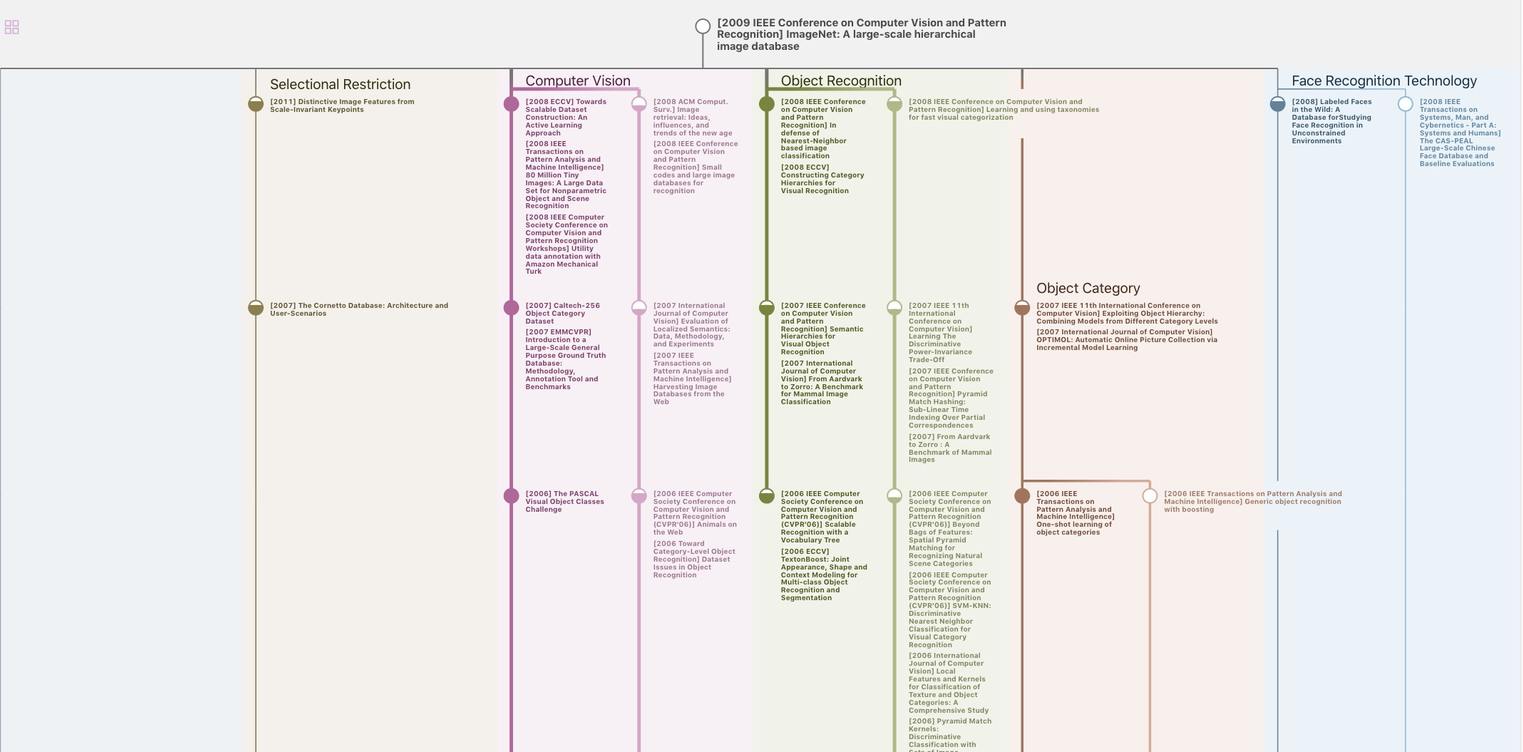
生成溯源树,研究论文发展脉络
Chat Paper
正在生成论文摘要