Identification of Fast Progressors Among Patients With Nonalcoholic Steatohepatitis Using Machine Learning
Gastro Hep Advances(2024)
摘要
Background and Aims: There is a high unmet need to develop noninvasive tools to identify nonalcoholic fatty liver disease/nonalcoholic steatohepatitis (NAFLD/NASH) patients at risk of fast progression to end-stage liver disease (ESLD). This study describes the development of a machine learning (ML) model using data around the first clinical evidence of NAFLD/NASH to identify patients at risk of future fast progression. Methods: Adult patients with ESLD (cirrhosis or hepatocellular carcinoma) due to NAFLD/NASH were identified in Optum electronic health records (2007–2018 period). Patients were stratified into fast (0.5 and 3 years) and standard progressor (6–10 years) cohorts based on retrospectively established progression time between ESLD and the earliest observable disease, and characteristics were reported using descriptive statistics. Two ML models predicting fast progression were created, performance was compared, and top predictive features from the final model were compared between cohorts. Results: Among a total of 4013 NAFLD patients with cirrhosis or hepatocellular carcinoma (mean age 58.6 ± 12.5; 65% female), 24% were fast (n = 951) and 25% standard (n = 992) progressors that were used for modeling. The cohorts were comparable for gender, body mass index, type 2 diabetes, and arterial hypertension, but differed significantly for obesity, hyperlipidemia, and age at index. The final model (NASH FASTmap) is a 44 feature light gradient boosting model which performed better (area under the curve [0.77], F1-score [0.74], accuracy [0.71], and precision [0.71]) than eXtreme gradient boosting model to predict fast progression. Conclusion: Future fast progression to ESLD in NAFLD/NASH patients can be predicted from clinical data using ML. Electronic health record implementation of NASH FASTmap could support clinical assessment for risk stratification and potentially improve disease management.
更多查看译文
关键词
Artificial Intelligence,Cirrhosis,Disease Progression,Risk Stratification
AI 理解论文
溯源树
样例
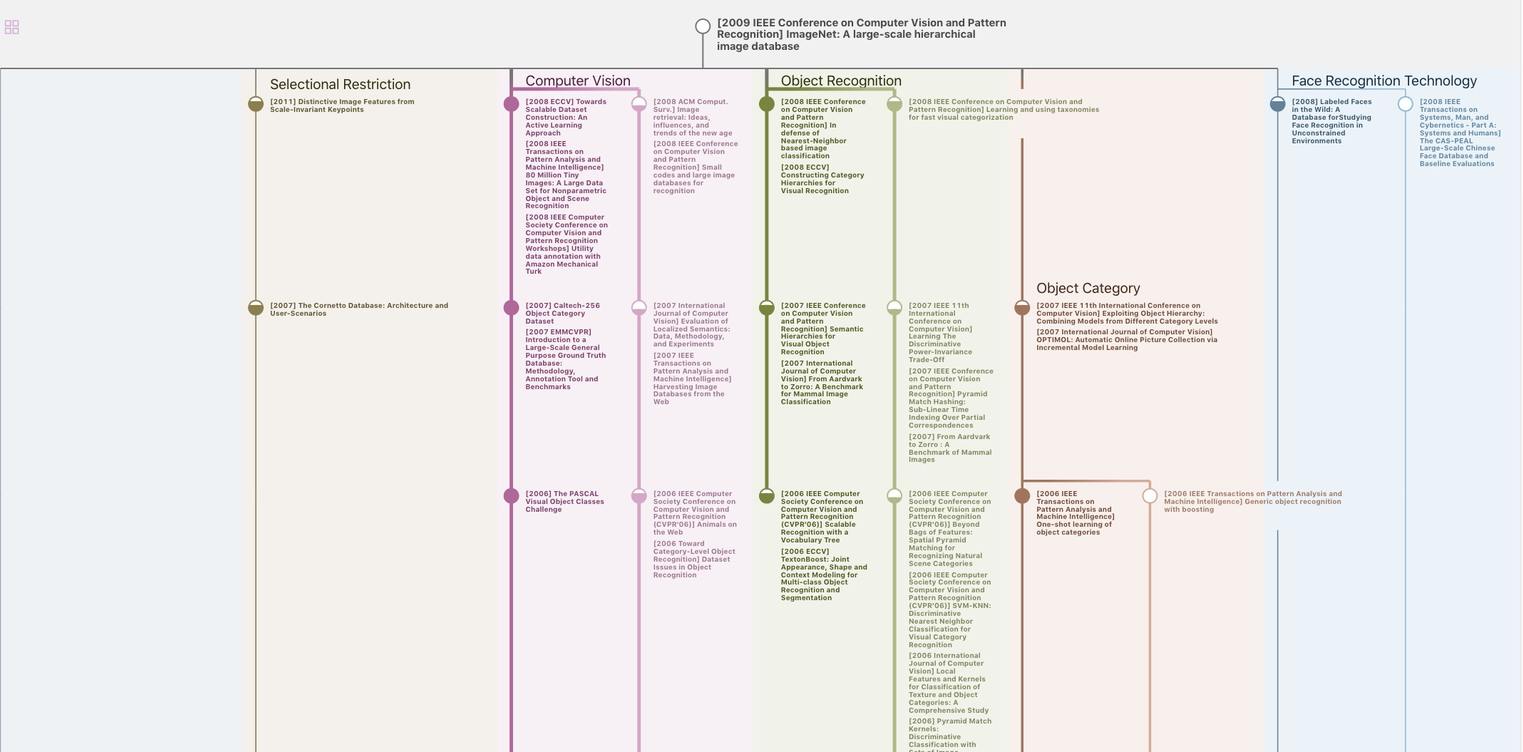
生成溯源树,研究论文发展脉络
Chat Paper
正在生成论文摘要