Energy-conserving hyper-reduction and temporal localization for reduced order models of the incompressible Navier-Stokes equations
JOURNAL OF COMPUTATIONAL PHYSICS(2024)
摘要
A novel hyper-reduction method is proposed that conserves kinetic energy and momentum for reduced order models of the incompressible Navier-Stokes equations. The main advantage of conservation of kinetic energy is that it endows the hyper-reduced order model (hROM) with a nonlinear stability property. The new method poses the discrete empirical interpolation method (DEIM) as a minimization problem and subsequently imposes constraints to conserve kinetic energy. Two methods are proposed to improve the robustness of the new method against error accumulation: oversampling and Mahalanobis regularization. Mahalanobis regularization has the benefit of not requiring additional measurement points. Furthermore, a novel method is proposed to perform energy- and momentum-conserving temporal localization with the principle interval decomposition: new interface conditions are derived such that energy and momentum are conserved for a full time-integration instead of only during separate intervals. The performance of the new energy- and momentum-conserving hyper-reduction methods and the energy- and momentum-conserving temporal localization method is analysed using three convection-dominated test cases; a shear-layer roll-up, two-dimensional homogeneous isotropic turbulence and a time-periodic inviscid flow consisting of a vortex in a uniform background flow. Our main finding is that energy conservation in combination with oversampling or regularization leads to a robust method with excellent long time stability properties. When any of these two ingredients is missing, accuracy and/or stability is significantly impaired.
更多查看译文
关键词
Discrete empirical interpolation method,Energy conservation,Incompressible Navier-Stokes equations,Reduced order models,Mahalanobis regularization,Temporal localization
AI 理解论文
溯源树
样例
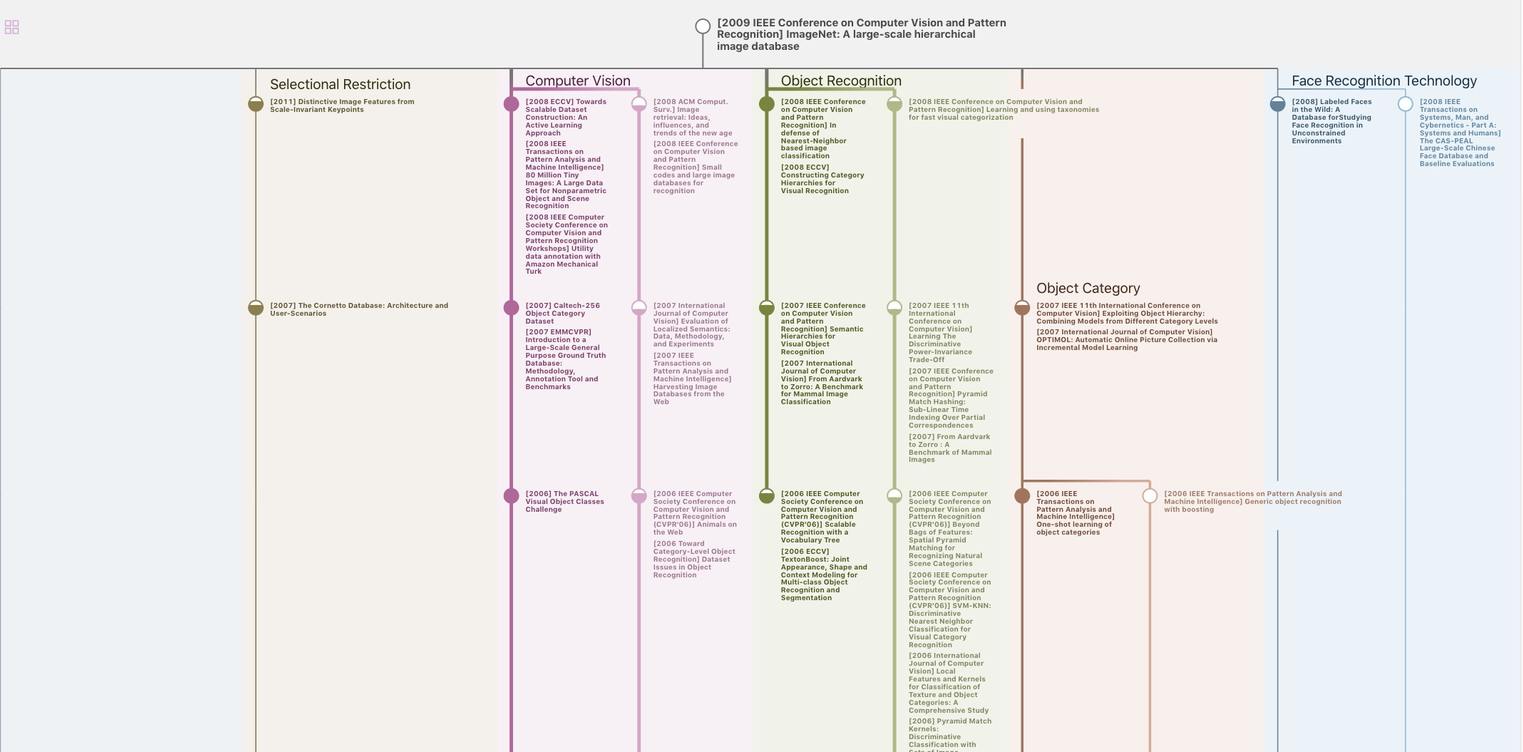
生成溯源树,研究论文发展脉络
Chat Paper
正在生成论文摘要