Stabilized activation scale estimation for precise Post-Training Quantization
NEUROCOMPUTING(2024)
摘要
In recent years, there have been more and more studies on Post-Training Quantization (PTQ). Many outstanding works have emerged, which has greatly promoted the availability of PTQ methods. However, in terms of low-bit quantization, there is still a wide gap between PTQ and the current state-of-the-art Quantization Aware Training (QAT) methods. In this work, we find that the current way of obtaining the activation scale is not completely reasonable in PTQ, which leads to the fact that the weight cannot be well adapted to the biased quantized activation during inference. Based on experiments and analysis, we propose a method called StablePTQ. It obtains a stable activation scale and mixes rich input into the block reconstruction to achieve an improving quantization accuracy. StablePTQ achieves remarkable improvements in several different bit quantization especially W2A4, and can be applied to other quantization algorithms as a plug-and-play approach. The source code of StablePTQ is avaiable at https://github.com/hustvl/StablePTQ.
更多查看译文
关键词
Post-training quantization,Exponential moving average,Mixed activation
AI 理解论文
溯源树
样例
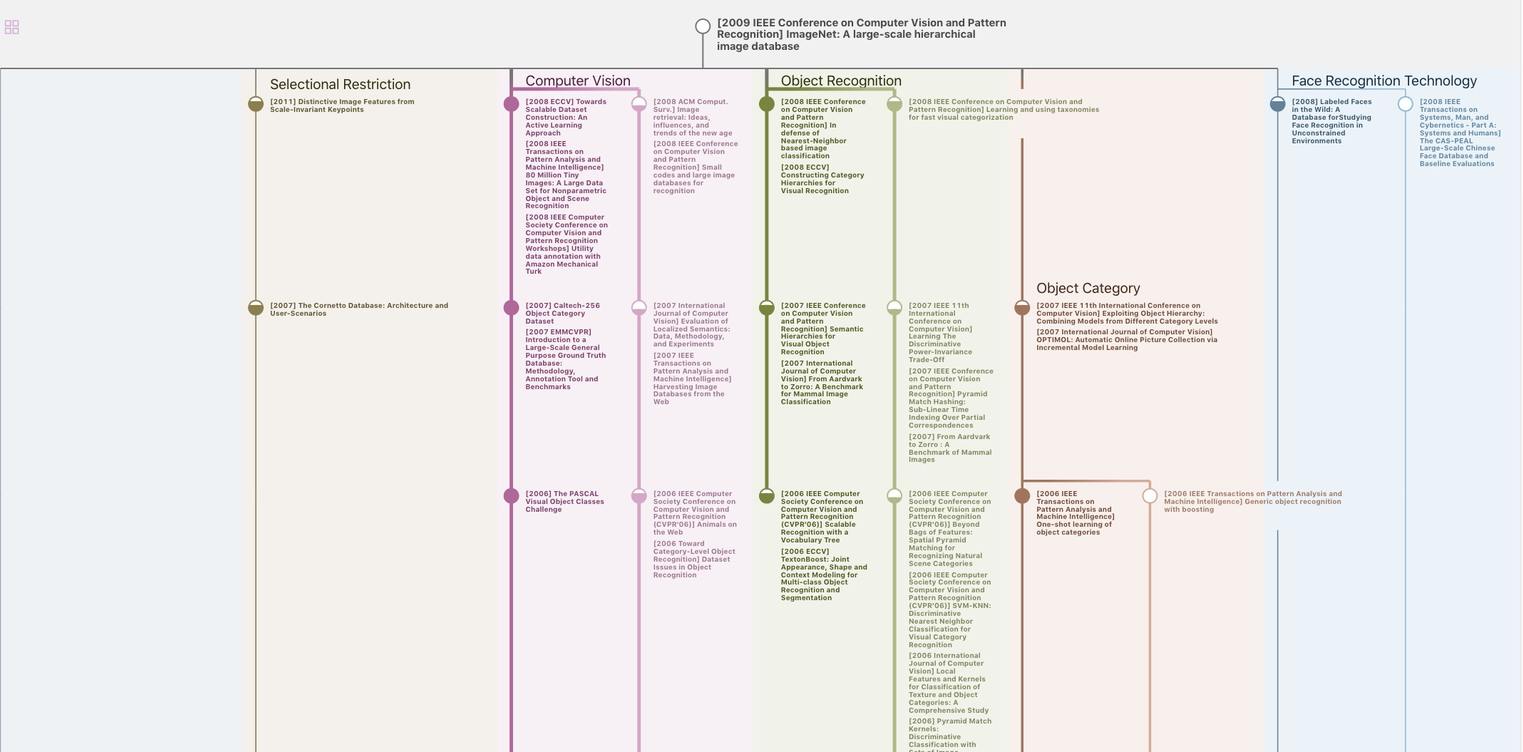
生成溯源树,研究论文发展脉络
Chat Paper
正在生成论文摘要