A semi-supervised deep learning approach for cropped image detection
EXPERT SYSTEMS WITH APPLICATIONS(2024)
摘要
Deep learning algorithms have shown great performance in multimedia forensics applications using supervised learning on large-scale labeled datasets. However, constructing such extensive labeled datasets can be challenging and costly in several multimedia forensics scenarios. Additionally, heavyweight deep learning models with complex architectures and a large number of parameters require significant hardware resources for training. To address these challenges in the context of image cropping detection, a common multimedia forensics application, we propose a semi-supervised deep learning framework capable of training on a large amount of unlabeled image samples. In this framework, we leverage a teacher model, trained on a small set of labeled image samples, to rank the confidence scores of image samples in a large-scale unlabeled dataset. By utilizing the ranked image samples, we train a student network successfully. To validate the effectiveness of our collaborative training framework across various image cropping detection scenarios, we conduct extensive experiments on a large-scale dataset. The experimental results clearly demonstrate that our semi-supervised learning approach achieved a state-of-the-art performance compared to existing supervised detection frameworks, achieving an accuracy of 91.79% on the BOSSbase dataset and 89.23% on the Alaska dataset. Furthermore, we conducted in-depth research on various factors that influence detection performance in the context of semi-supervised learning. These factors include pairings of teacher-student models, the top K selection approach, the number of unlabeled samples, the number of iterations in self-training, and the proportion of high-confidence samples using in semi-supervised learning.
更多查看译文
关键词
Image cropping,Semi-supervised learning,Multimedia forensics,Deep learning algorithms,Unlabeled data
AI 理解论文
溯源树
样例
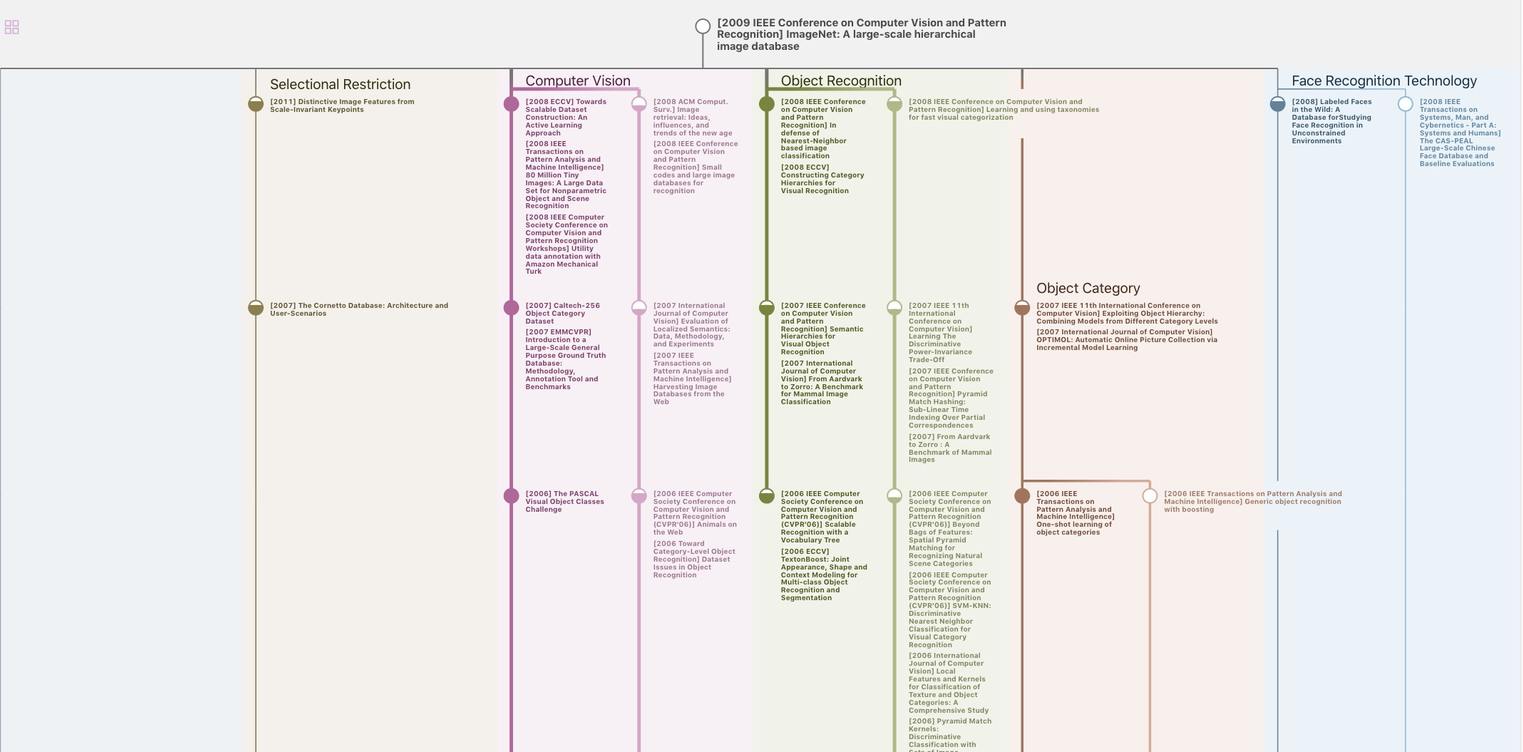
生成溯源树,研究论文发展脉络
Chat Paper
正在生成论文摘要