SilentTrig: An imperceptible backdoor attack against speaker identification with hidden triggers
PATTERN RECOGNITION LETTERS(2024)
摘要
Speaker identification based on deep learning is known to be susceptible to backdoor attacks. However, the current research on audio backdoor attacks is limited, and these attacks often use obvious noises as triggers, which can raise suspicion among users. In this paper, we introduce SilentTrig, a novel and imperceptible backdoor attack method targeted at speaker identification. Our approach involves utilizing an optimized steganographic network to embed triggers into benign audio samples and implementing a two-stage adversarial optimization process. This ensures that the poisoned samples are acoustically indistinguishable from their benign counterparts, resulting in a substantially improved attack imperceptibility. We evaluate SilentTrig on two datasets and four state-of-the-art models. The results demonstrate a high Attack Success Rate (ASR) of up to 99.2%, a Just Noticeable Difference (JND) of only 0.3, and resistance to typical defense methods such as Neural Cleanse and Fine-Pruning.
更多查看译文
关键词
Speaker identification,Backdoor attack,Trigger,Deep learning,Imperceptibility
AI 理解论文
溯源树
样例
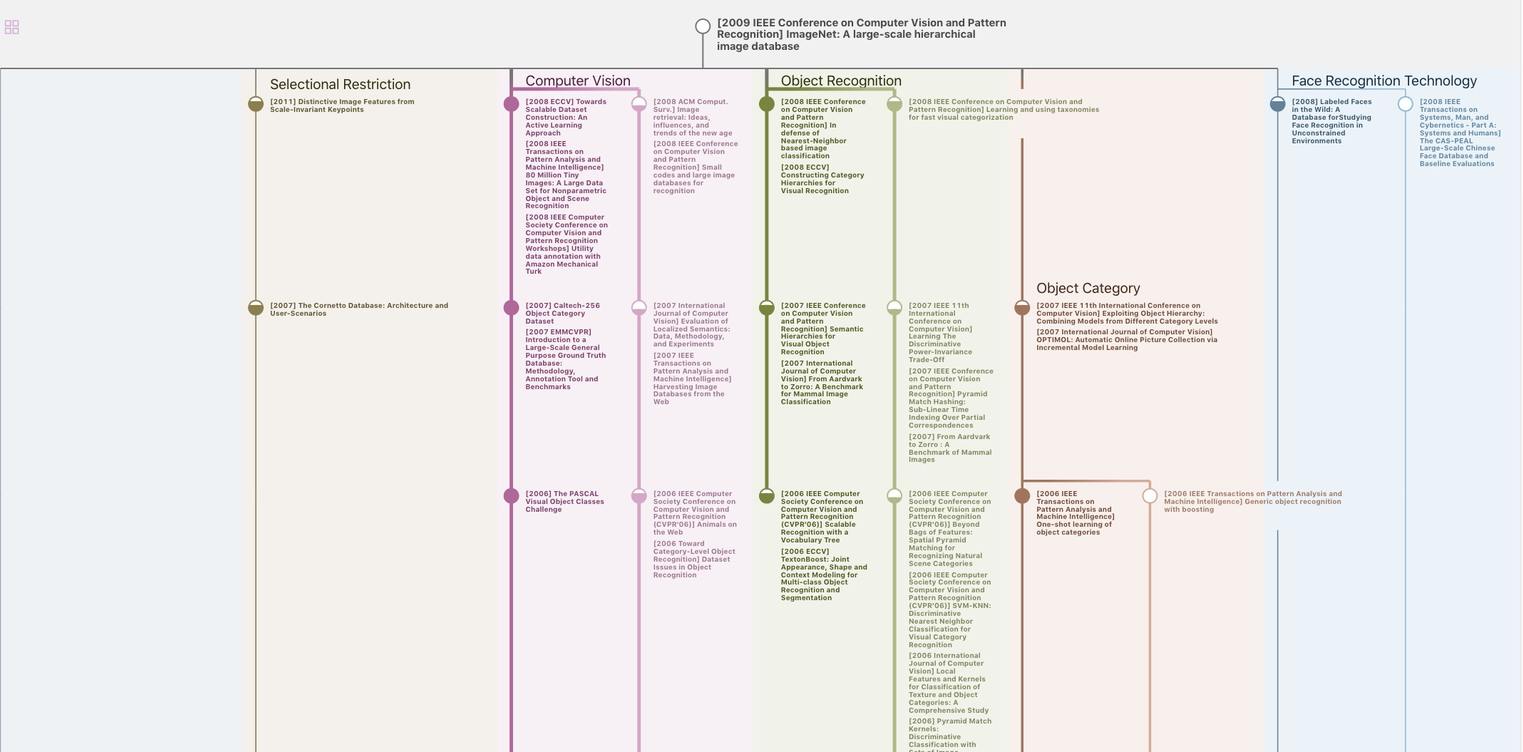
生成溯源树,研究论文发展脉络
Chat Paper
正在生成论文摘要