Design of graphene-based terahertz absorber and machine learning prediction model
OPTICS COMMUNICATIONS(2024)
摘要
In today's revolution of artificial intelligence, machine learning (ML) has come up with providing most rapid, accurate solution towards many complicated design problems. In this paper, a regression-based machine learning model has been developed, trained, and tested to forecast the performance of the Terahertz absorber. Different parameter variations of the dual circular ring Graphene FSS absorber viz., periodicity, thicknesses and radius of circular rings have been performed to attain maximum absorption of 99.9 % at center frequency 10.5 THz and bandwidth 1 THz (10.0 THz - 11.0 THz). The efficiency of nine different regression models (Keras regressor, Histogram gradient regressor, adaboost regressor, gradient boosting regressor, random forest regressor, decision tree regressor, k neighbours regressor, ridge regression, and linear regression) were tested against accurately predicting the absorption values and their performances were compared using R2 score and RSME. The study yielded very good R2 scores (near to 1.0) in case of random forest regression, thereby, demonstrating its effectiveness for future absorptivity prediction. The developed model considerably reduces time and effort in accurate prediction of the design parameters for THz absorber for any given absorptivity.
更多查看译文
关键词
Graphene,Terahertz absorber,Machine learning,Random forest,Regression model
AI 理解论文
溯源树
样例
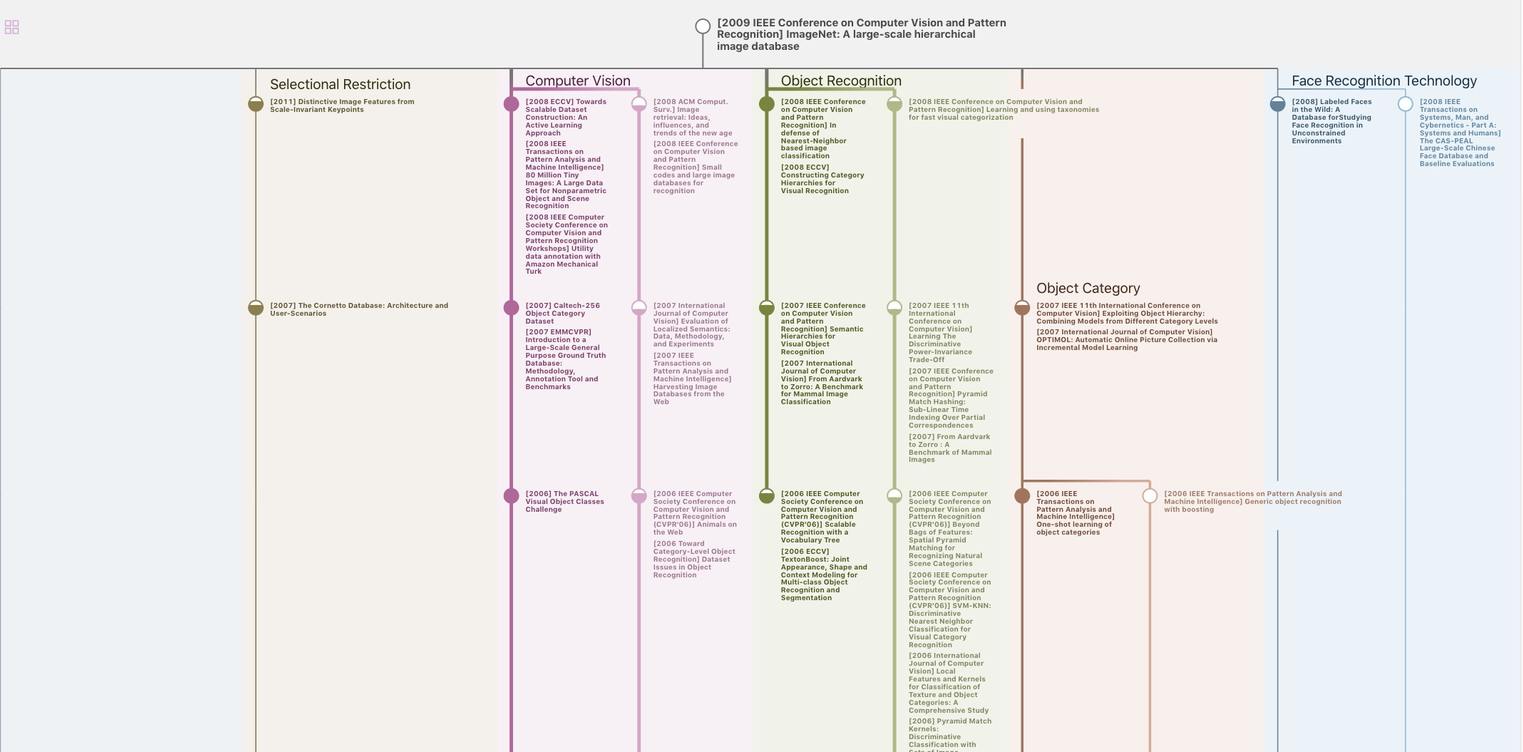
生成溯源树,研究论文发展脉络
Chat Paper
正在生成论文摘要