Hybrid machine learning model for malware analysis in android apps
PERVASIVE AND MOBILE COMPUTING(2024)
摘要
Android smartphones have been widely adopted across the globe. They have the capability to access private and confidential information resulting in these devices being targeted by malware devisers. The dramatic escalation of assaults build an awareness to create a robust system that detects the occurrence of malicious actions in Android applications. The malware exposure study consists of static and dynamic analysis. This research work proposed a hybrid machine learning model based on static and dynamic analysis which offers efficient classification and detection of Android malware. The proposed novel malware classification technique can process any android application, then extracts its features, and predicts whether the applications under process is malware or benign. The proposed malware detection model can characterizes diverse malware types from Android platform with high positive rate. The proposed approach detects malicious applications in reduced execution time while also improving the security of Android as compared to existing approaches. State-of-the-art machine learning algorithms such as Support Vector Machine, k-Nearest Neighbor, Naive Bayes, and different ensembles are employed on benign and malign applications to assess the execution of all classifiers on permissions, API calls and intents to identify malware. The proposed technique is evaluated on Drebin, MalGenome and Kaggle dataset, and outcomes indicate that this robust system improved runtime detection of malware with high speed and accuracy. Best accuracy of 100% is achieved on benchmark dataset when compared with state of the art techniques. Furthermore, the proposed approach outperforms state of the art techniques in terms of computational time, true positive rate, false positive rate, accuracy, precision, recall, and f-measure.
更多查看译文
关键词
Android,Malware detection,Machine learning,Ensemble learning,Classification
AI 理解论文
溯源树
样例
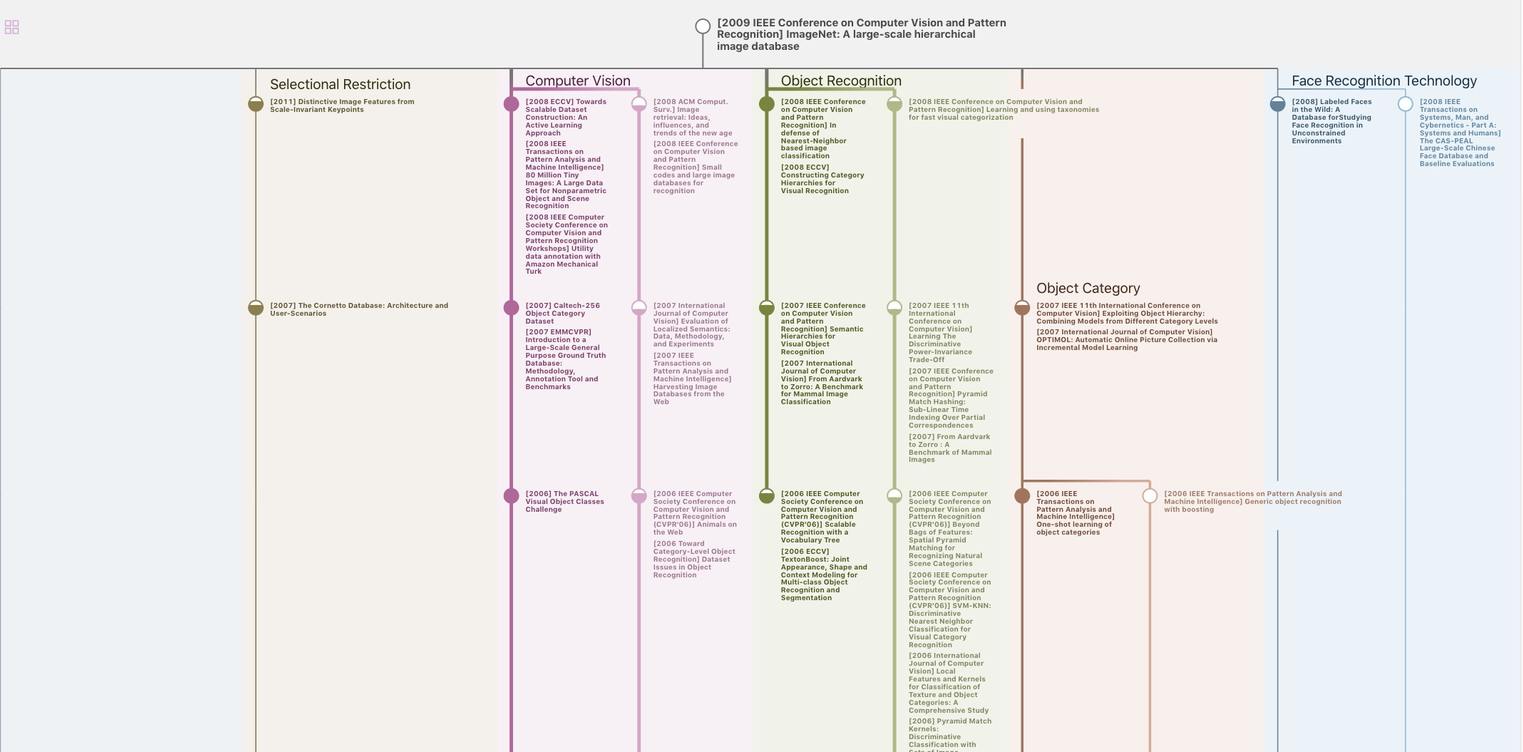
生成溯源树,研究论文发展脉络
Chat Paper
正在生成论文摘要