A fast ADMM algorithm for sparse precision matrix estimation using lasso penalized D-trace loss
EGYPTIAN INFORMATICS JOURNAL(2024)
摘要
Sparse precision matrix estimation, also known as the estimation of the inverse covariance matrix in statistical contexts, represents a critical challenge in numerous multivariate analysis applications. This challenge becomes notably complex when the dimension of the data is far greater than the capacity of samples. To address this issue, we introduce a convex relaxation model that employs the first-order optimality conditions associated with the lasso-penalized D-trace loss for the purpose of estimating sparse precision matrix. The proposed model is effectively solved through the widely recognized alternating direction method of multipliers. Additionally, we provide closed-form solutions to subproblems in each iteration with a computational complexity of 0(np2), and establish the convergence of our proposed algorithm. Numerical investigations demonstrate that our algorithm exhibits the capability to handle large-scale datasets and significantly outperforms the existing methods, particularly when dealing with high-dimensional scenarios characterized by a large dimension p.
更多查看译文
关键词
Precision matrix,Penalized quadratic loss,Convex relaxation,Gaussian graphical model,ADMM
AI 理解论文
溯源树
样例
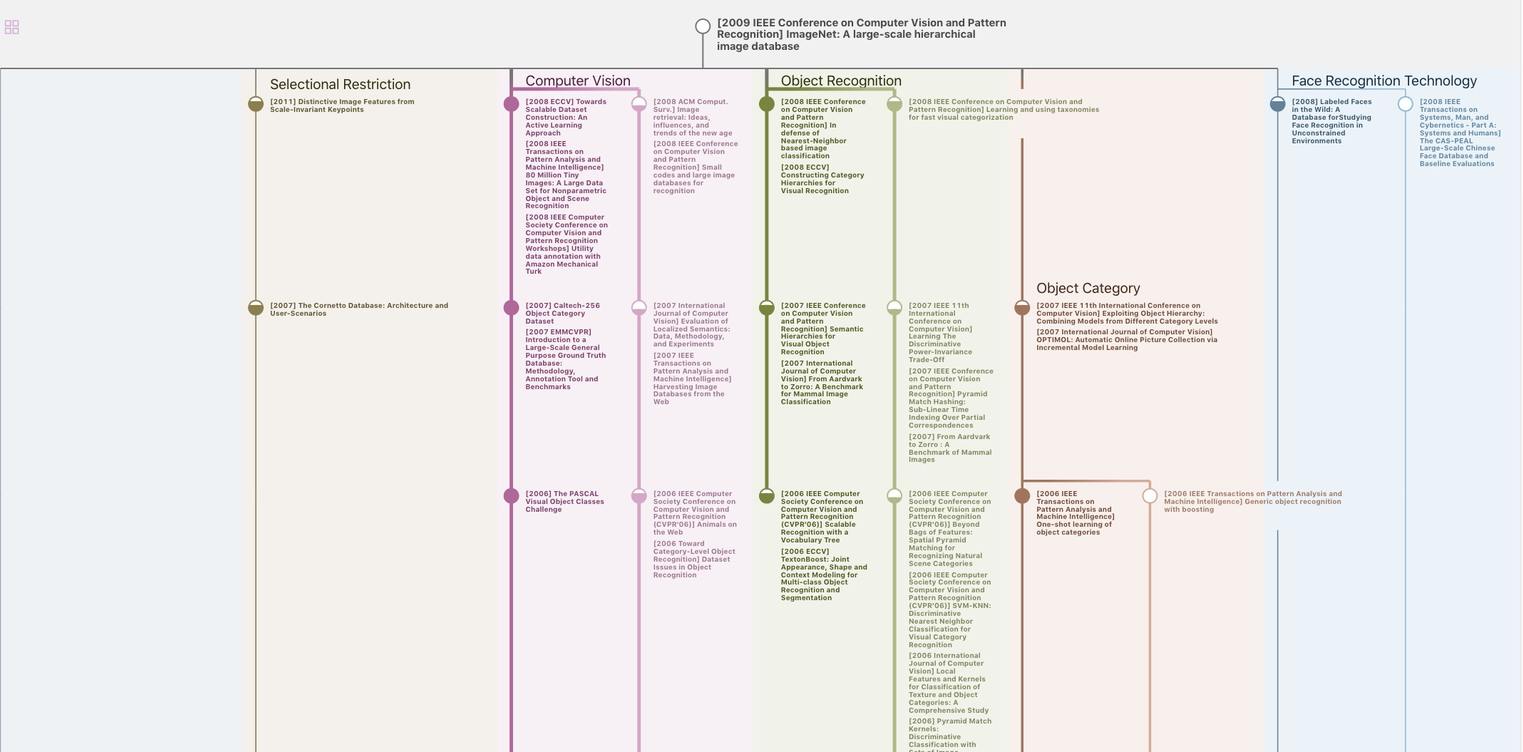
生成溯源树,研究论文发展脉络
Chat Paper
正在生成论文摘要