DFS-WR: A novel dual feature selection and weighting representation framework for classification
INFORMATION FUSION(2024)
摘要
Classification methods relying on multidimensional features have been widely applied in commerce, healthcare, and transportation. Nevertheless, haphazardly selecting inappropriate features may not only hinder the improvement of classification performance but also disrupt the convergence of classifier parameters, leading to prolonged classifier runtime and an increased storage burden. Therefore, this paper proposed a novel framework (dubbed as DFS-WR) to perform dual selection and weighted representation of multidimensional features. Firstly, DFS-WR employs Laplacian scores to conduct a de-redundant operation as the first feature selection. Subsequently, DFS-WR utilizes a correlation coefficient matrix and redundant sorting to eliminate duplicate deployment of similar features in classification through the second selection. Finally, for hierarchical expression, DFS-WR assigns weights by virtue of information gain (IG), thereby emphasizing the significance of dominant features. The performance of DFS-WR was further evaluated across four aspects: classification ability, runtime performance, storage occupancy, and portability and universality, using five public datasets and eleven widely adopted classifiers. Additionally, this paper considered the proper configuration of crucial parameters within DFS-WR and compared it with other related works. The results indicated that the dual selection strategy for eliminating redundancy and similarities among features could improve the performance of classifiers, and the weighted process based on IG outperformed unweighted and ReliefF-based weighted methods. By employing DFS-WR to multidimensional features, the classifier's runtime and data storage capacity were effectively reduced. In addition, it was proved that the deployment of DFS-WR generally had no limitations on datasets and classifiers, underscoring its good portability and universality in practical applications.
更多查看译文
关键词
Dual feature selection,Multidimensional feature classification,Redundant feature elimination,Similarity feature elimination,Feature weighting,Information gain
AI 理解论文
溯源树
样例
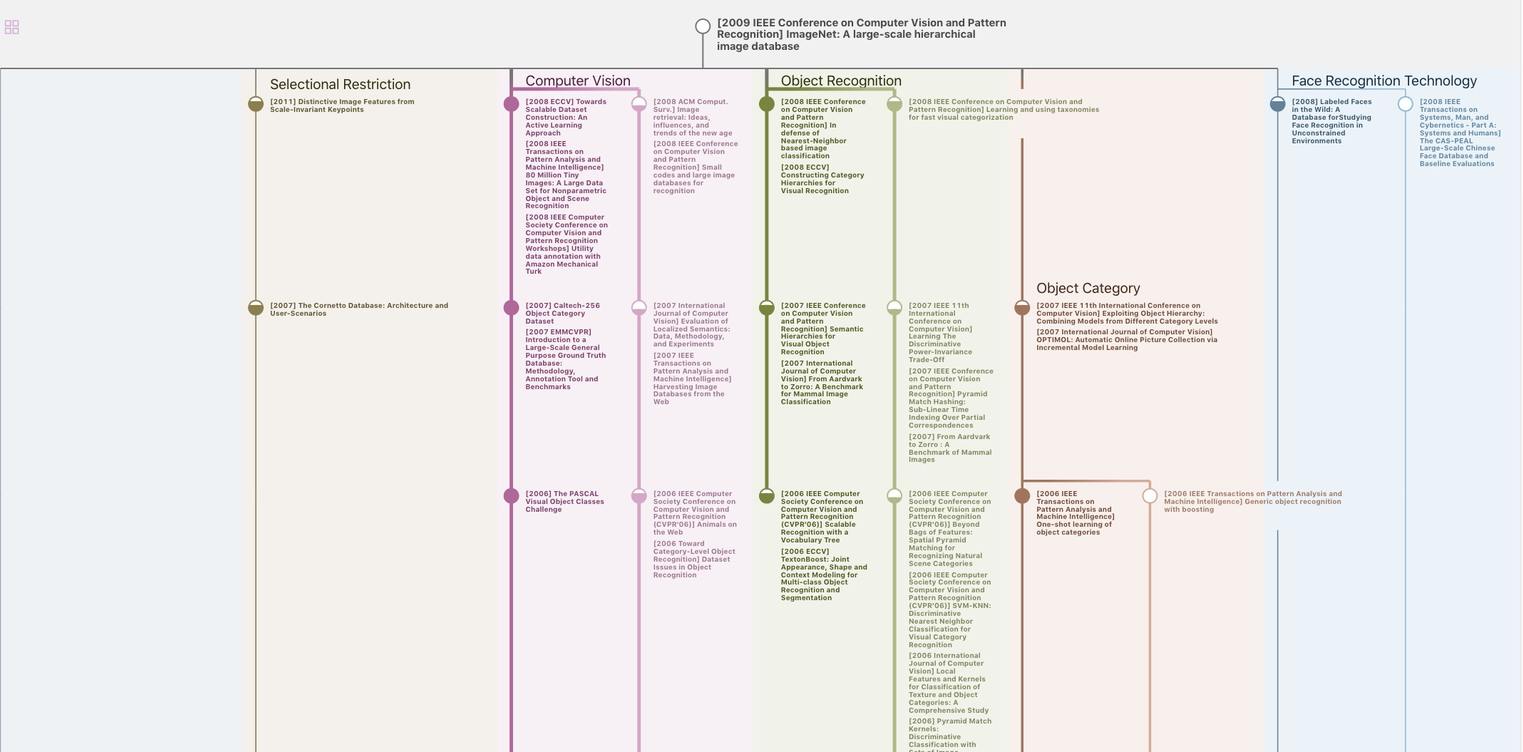
生成溯源树,研究论文发展脉络
Chat Paper
正在生成论文摘要