On tuning parameters guiding similarity computations in a data deduplication pipeline for customers records Experience from a R&D project
INFORMATION SYSTEMS(2024)
摘要
Data stored in information systems are often erroneous. Duplicate data are one of the typical error type. To discover and handle duplicates, the so-called deduplication methods are applied. They are complex and time costly algorithms. In data deduplication, pairs of records are compared and their similarities are computed. For a given deduplication problem, challenging tasks are: (1) to decide which similarity measures are the most adequate to given attributes being compared and (2) defining the importance of attributes being compared, and (3) defining adequate similarity thresholds between similar and not similar pairs of records. In this paper, we summarize our experience gained from a real R&D project run for a large financial institution. In particular, we answer the following three research questions: (1) what are the adequate similarity measures for comparing attributes of text data types, (2) what are the adequate weights of attributes in the procedure of comparing pairs of records, and (3) what are the similarity thresholds between classes: duplicates, probably duplicates, non-duplicates? The answers to the questions are based on the experimental evaluation of 54 similarity measures for text values. The measures were compared on five different real data sets of different data characteristic. The similarity measures were assessed based on: (1) similarity values they produced for given values being compared and (2) their execution time. Furthermore, we present our method, based on mathematical programming, for computing weights of attributes and similarity thresholds for records being compared. The experimental evaluation of the method and its assessment by experts from the financial institution proved that it is adequate to the deduplication problem at hand. The whole data deduplication pipeline that we have developed has been deployed in the financial institution and is run in their production system, processing batches of over 20 million of customer records.
更多查看译文
关键词
Data quality,Entity resolution,Entity matching,Data deduplication,Data deduplication pipeline,Customers records deduplication,Text similarity measures,Customer data,Python packages,Mathematical programming,Attribute weights,Similarity thresholds
AI 理解论文
溯源树
样例
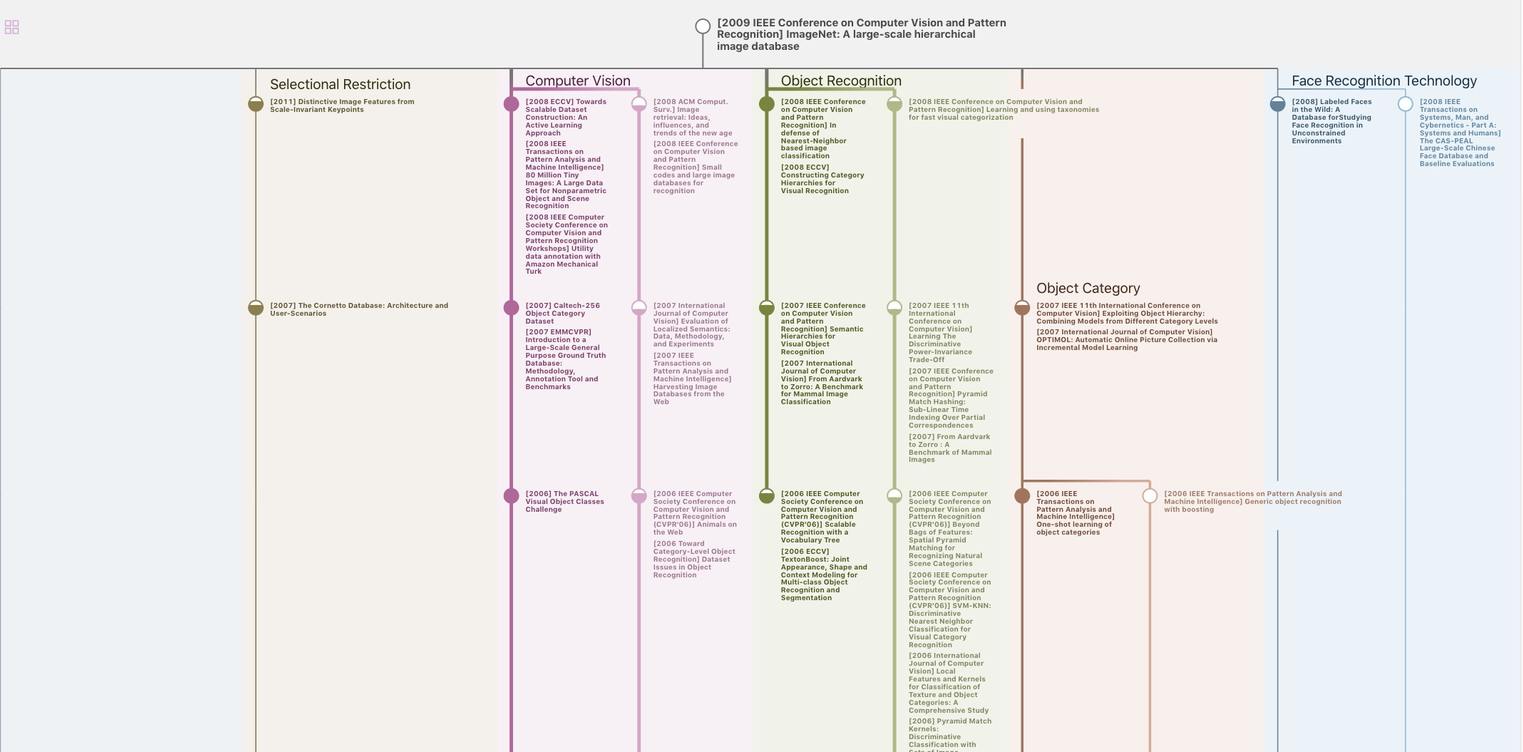
生成溯源树,研究论文发展脉络
Chat Paper
正在生成论文摘要