Improvements in Language Modeling, Voice Activity Detection, and Lexicon in OpenASR21 Low Resource Languages
SPEECH AND COMPUTER, SPECOM 2023, PT II(2023)
摘要
OpenASR21 evaluation was on 15 low resource languages and 3 case sensitive languages. During the evaluation, participants got significant reduction in word error rates (WER) with text downloaded from the internet for only the case sensitive languages, since the development and evaluation audio contained broadcast news. For the 15 low resource languages, participants showed only small gains for some of the languages. The reason is that the development and test set contain dialog between two people, which is very different from the primarily news texts and web pages available over the internet. Here, we show that training text translated from other OpenASR21 languages reduces the WER for many languages. During the evaluation, one team added words to the lexicon using a 3-gram phone language model, but they do not show what WER reduction they achieve. We show that adding new words in the lexicon from public text is beneficial for languages where the out-of-vocabulary rate is high, and outline conditions for reducing the WER. Adding an attention layer to the TDNN (time delay neural net) based voice activity detector reduced the WER for 17 out of the 18 languages. With all the improvements combined, we are getting lower word error rate for the development set for three languages (Farsi, Kazakh and Tamil) than the site that achieved the best error rate for all the languages during the evaluation period.
更多查看译文
关键词
OpenASR21,Low-resource,Speech recognition,Language modeling
AI 理解论文
溯源树
样例
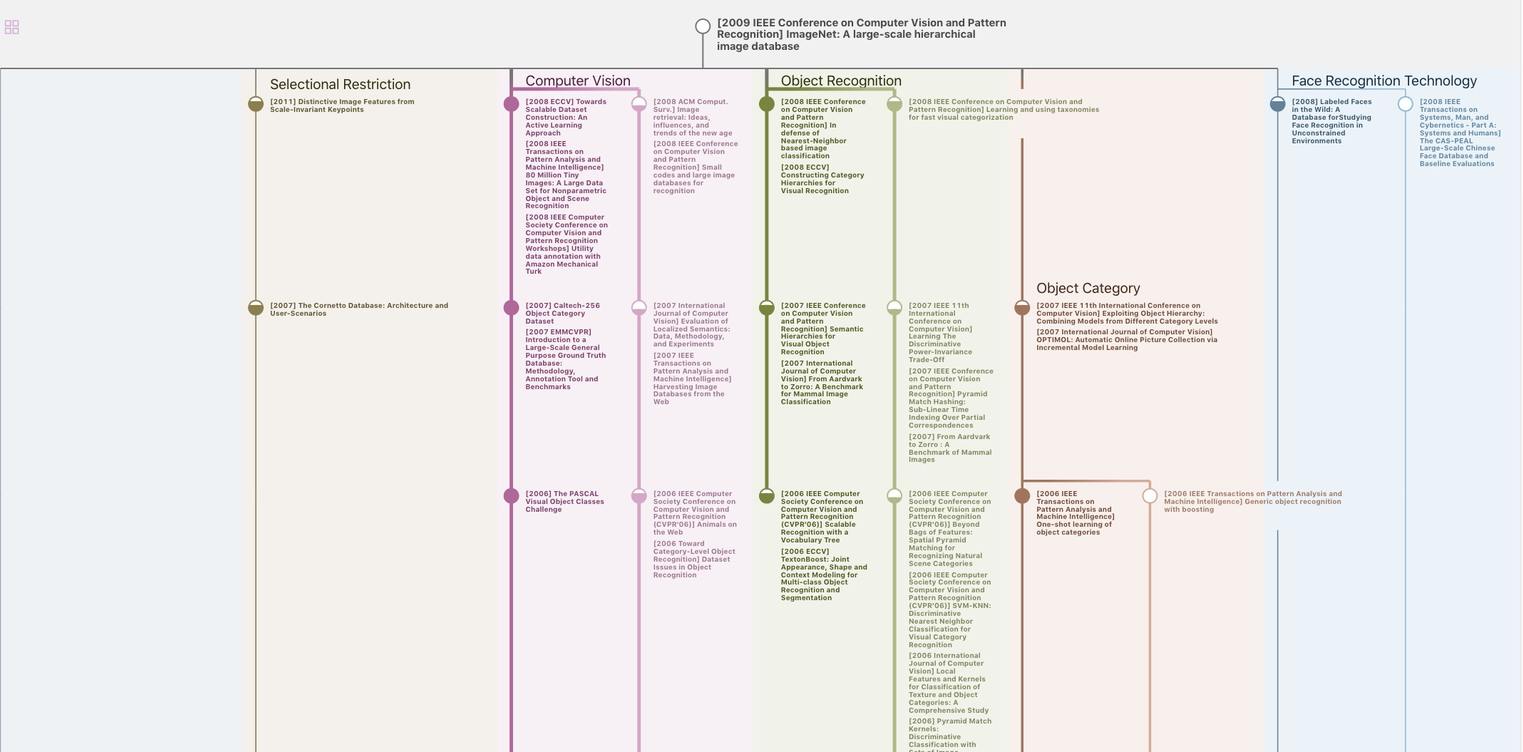
生成溯源树,研究论文发展脉络
Chat Paper
正在生成论文摘要