Analysing Breathing Patterns in Reading and Spontaneous Speech
SPEECH AND COMPUTER, SPECOM 2023, PT II(2023)
摘要
This paper focuses on the time and phase-domain analysis of speech signals to extract breathing patterns. The speech signals under investigation fall into two categories: reading and spontaneous speaking. We introduce SBreathNet, a deep Long Short-Term Memory (LSTM) based regressive model, to extract breathing patterns from speech signals. SBreathNet is trained with speech collected from 100 individuals reading a phonetically balanced text and extracts the breathing patterns with an average Pearson correlation coefficient (r-value) of 0.61 with the true breathing signal captured using a respiratory belt. The average breaths-per-minute error (BPME) across 100 speakers is 2.50. The analysis is done using leave-one-speaker-out approach. Similarly, when SBreathNet is trained with spontaneous speech signals, it extracts the breathing patterns with an r-value of 0.41 and an average BPME of 3.9. By comparing the performance across speakers, speech categories, and speech-breathing categories, we aim to uncover the factors influencing SBreathNet's effectiveness when applied to these two types of speech signals.
更多查看译文
关键词
Speech analysis,Computational paralinguistics,Speech-breathing patterns,Health informatics
AI 理解论文
溯源树
样例
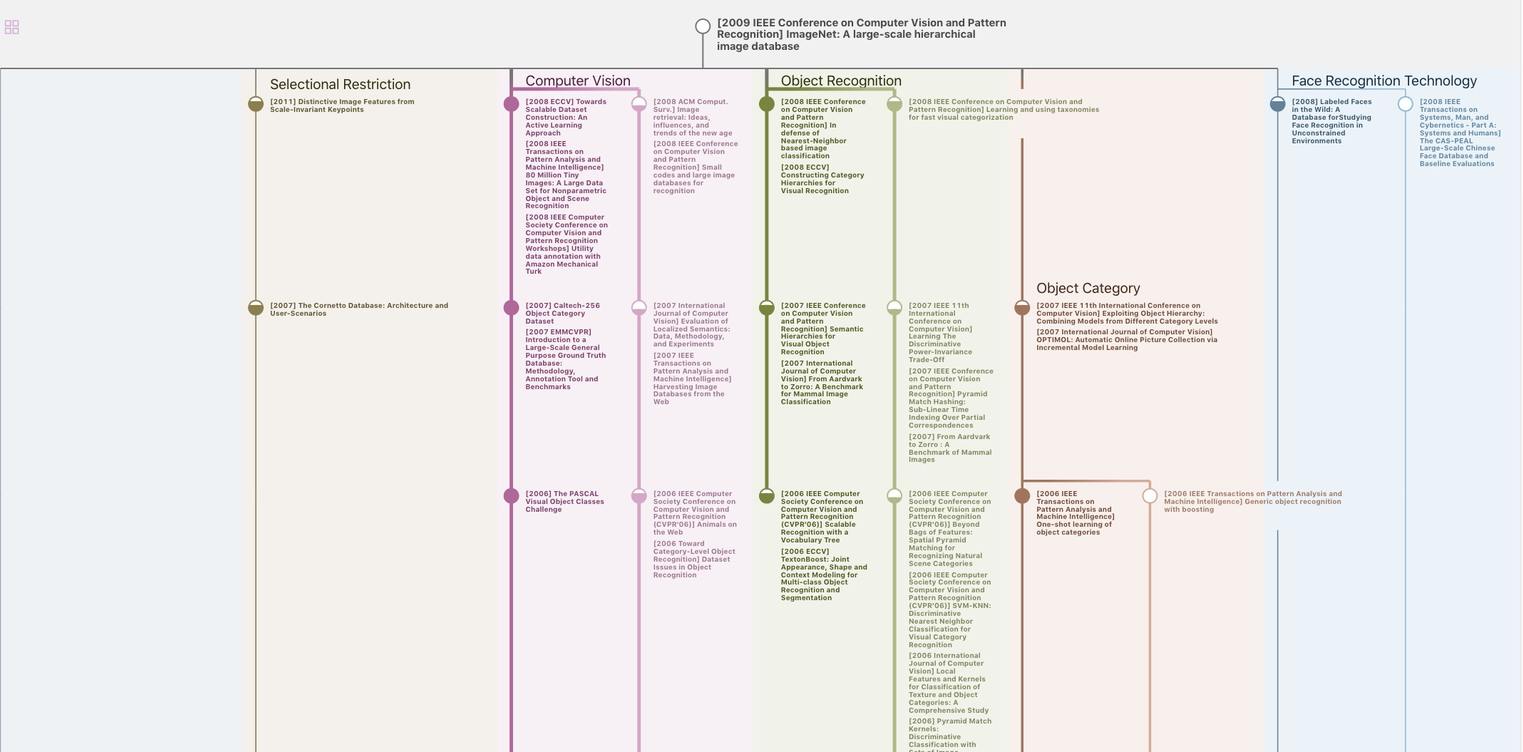
生成溯源树,研究论文发展脉络
Chat Paper
正在生成论文摘要