Domain Generalization for Foreground Segmentation Using Federated Learning
ADVANCES IN VISUAL COMPUTING, ISVC 2023, PT I(2023)
摘要
Foreground segmentation is one of the main applications in computer vision, as it separates an object in an image from its background. State-of-the-art models are achieving great metrics in terms of object segmentation tasks. However, when these models are subject to domain shift, their performance massively degrades. This is due to poor generalization. Domain generalization can be achieved by training the model using multiple datasets from different domains. However, most of the existing foreground segmentation models are unable to learn effectively from multiple datasets. If the training of multiple datasets is done simultaneously, the model cannot learn the different distributions, as each distribution requires its own set of parameters. Moreover, if the training is done sequentially, the models will struggle to perform well on all datasets due to a problem called catastrophic forgetting. This paper proposes a deep learning model and a novel training procedure to learn from multiple domains while overcoming the mentioned problems effectively. The proposed model is an encoder with a multi-head decoder that combines shared and isolated parameters for different domains. The proposed training procedure is similar to federated learning, where client models are created such that each client learns from a specific domain. After that, the main model learns the knowledge gained by each client using knowledge distillation with regularization to prevent the main network from forgetting the previously gained knowledge. Experiments demonstrate the effectiveness of our proposed work in comparison with state-of-the-art models.
更多查看译文
关键词
Foreground segmentation,Domain generalization,Knowledge distillation,Federated learning,Deep learning
AI 理解论文
溯源树
样例
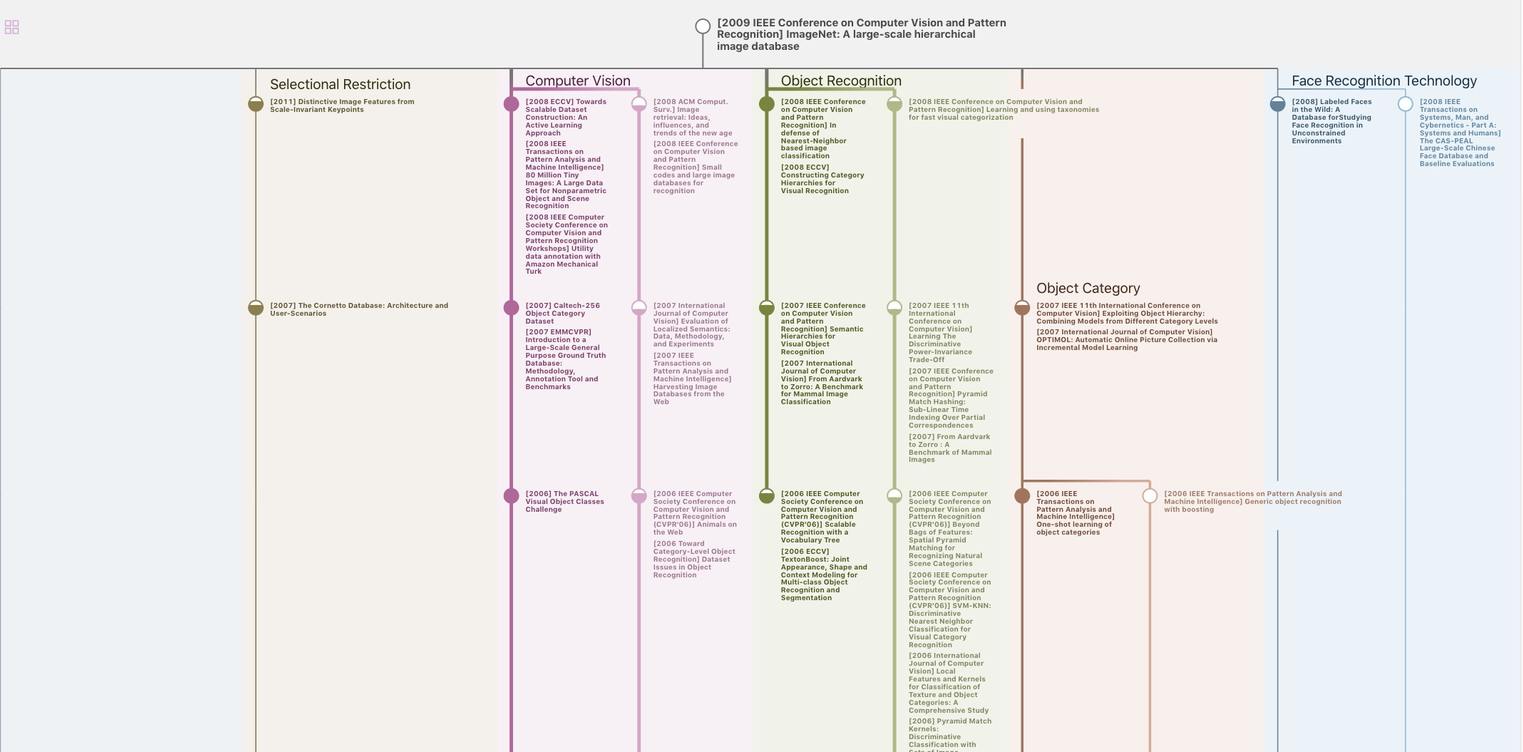
生成溯源树,研究论文发展脉络
Chat Paper
正在生成论文摘要