Exploiting Generative Adversarial Networks in Joint Sensitivity Encoding for Enhanced MRI Reconstruction
ADVANCES IN VISUAL COMPUTING, ISVC 2023, PT II(2023)
摘要
In this paper, we propose a novel approach for improving the quality of Parallel Magnetic Resonance Imaging (pMRI) reconstructions by incorporating the power of Generative Adversarial Networks (GANs). We integrate the Joint Sensitivity Encoding (JSENSE) technique with a GAN for parallel magnetic resonance imaging (MRI). The innovation lies in refining the JSENSE iterative reconstruction process using a GAN which effectively addresses the persistent challenge of low signal-to-noise ratio (SNR) and artifact degradation. While JSENSE offers improved reconstruction in rapid scanning or under-sampled acquisitions, images often exhibit noise and aliasing artifacts when the reduction factor is high. To resolve this problem, we deployed a GAN within the JSENSE framework for image-to-image translation and transforming noisy and artifact-ridden images into high-quality ones. Our GAN model, trained on paired sets of clean and noisy MRI images, performs noise and artifact removal after each JSENSE reconstruction iteration. Comparative evaluations with standard JSENSE and other contemporary techniques, such as CG-SENSE indicate significant improvement in the quality of the proposed method. Our approach achieved superior Structural Similarity Index Measure (SSIM) and lower Normalized Mean Squared Error (NMSE) with increased reduction factors and demonstrated its effectiveness in high-quality MRI reconstruction.
更多查看译文
关键词
Magnetic Resonance Imaging,MRI reconstruction,Generative Adversarial Network,Parallel MRI
AI 理解论文
溯源树
样例
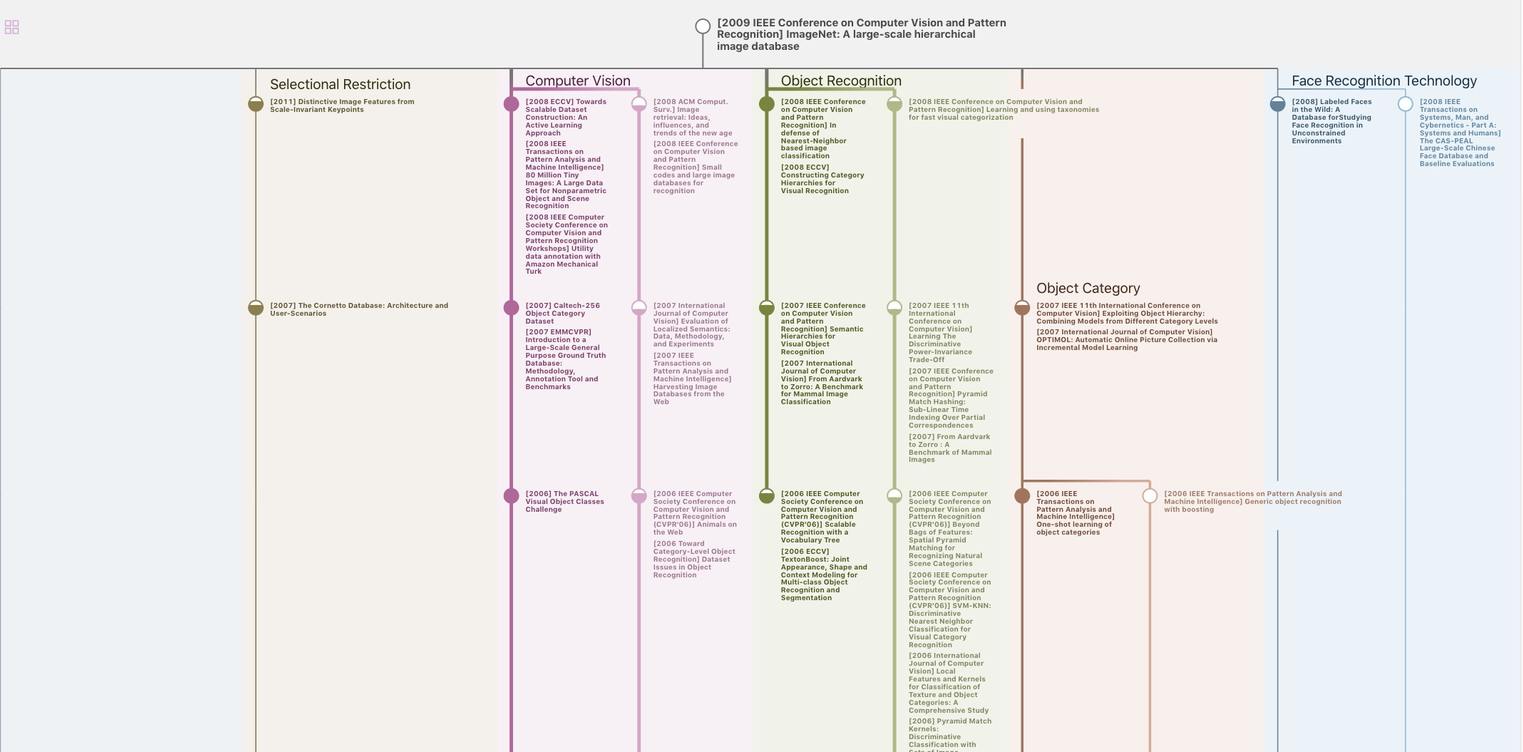
生成溯源树,研究论文发展脉络
Chat Paper
正在生成论文摘要