Co-attention-Based Pairwise Learning for Author Name Disambiguation
LEVERAGING GENERATIVE INTELLIGENCE IN DIGITAL LIBRARIES: TOWARDS HUMAN-MACHINE COLLABORATION, ICADL 2023, PT II(2023)
摘要
Digital libraries face a pressing issue of author name ambiguity. This paper proposes a novel pairwise learning model for author name disambiguation, utilizing self-attention and co-attention mechanisms. The model integrates textual, discrete, and co-author attributes, amongst others, to capture comprehensive information from bibliographic records. It incorporates an optional random projection-based dimension reduction technique for efficiency to handle large datasets. The attention weight visualizations provide explanations for the model's predictions. Our experiments on a substantial bibliographic catalogue repository validate the model's effectiveness using accuracy, F1, and ROC AUC scores.
更多查看译文
关键词
Author name disambiguation,Attention mechanisms,dExplainable machine learning,Feature Integration
AI 理解论文
溯源树
样例
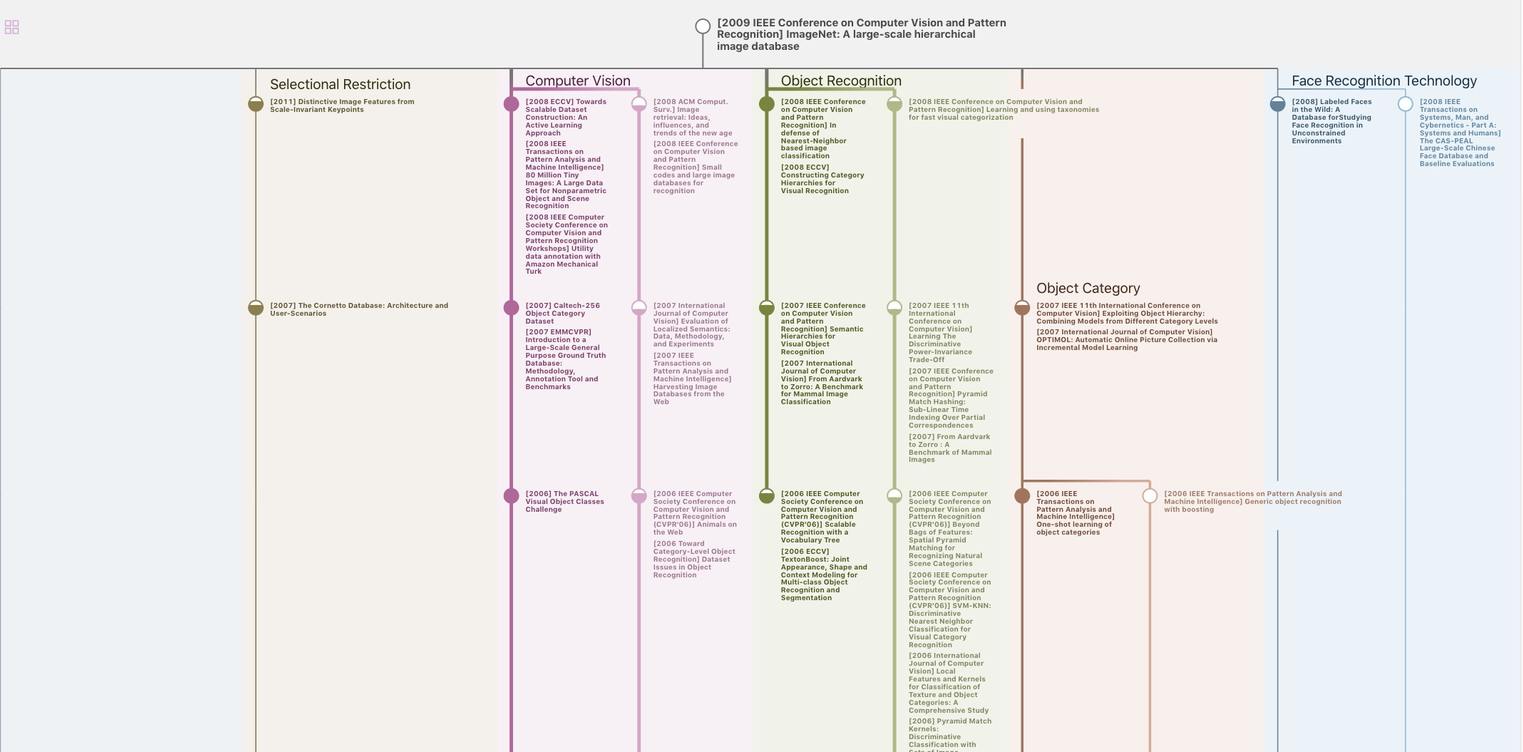
生成溯源树,研究论文发展脉络
Chat Paper
正在生成论文摘要