Trustworthy adaptive adversarial perturbations in social networks
JOURNAL OF INFORMATION SECURITY AND APPLICATIONS(2024)
摘要
Deep neural networks have achieved excellent performance in various research and applications, but they have proven to be susceptible to adversarial examples. Generating adversarial examples can help identify the vulnerability of the deep neural networks and further enhance the robustness and reliability of these models. However, the existing adversarial attacks can hardly achieve the balance between robustness and imperceptibility, which is not trustworthy in social networks. To solve these problems, we propose adaptive adversarial perturbation (AAP) to improve the universal robustness of the adversarial examples while ensuring imperceptibility. To optimize the imperceptibility of the perturbation, we design a noise visibility function (NVF) to reflect the features of the original images based on the human visual system (HVS). By further calculating a coefficient matrix based on the NVF, the perturbation intensity of different pixels can be adjusted dynamically to improve the robustness. The experimental results prove that the proposed method alleviates the trade-off between robustness and imperceptibility, and outperforms existing attack methods in both one-step and iterative ways. Our method makes the adversarial attack more reliable and applicable in social networks.
更多查看译文
关键词
Social networks,Neural networks,Adversarial examples,Human visual system
AI 理解论文
溯源树
样例
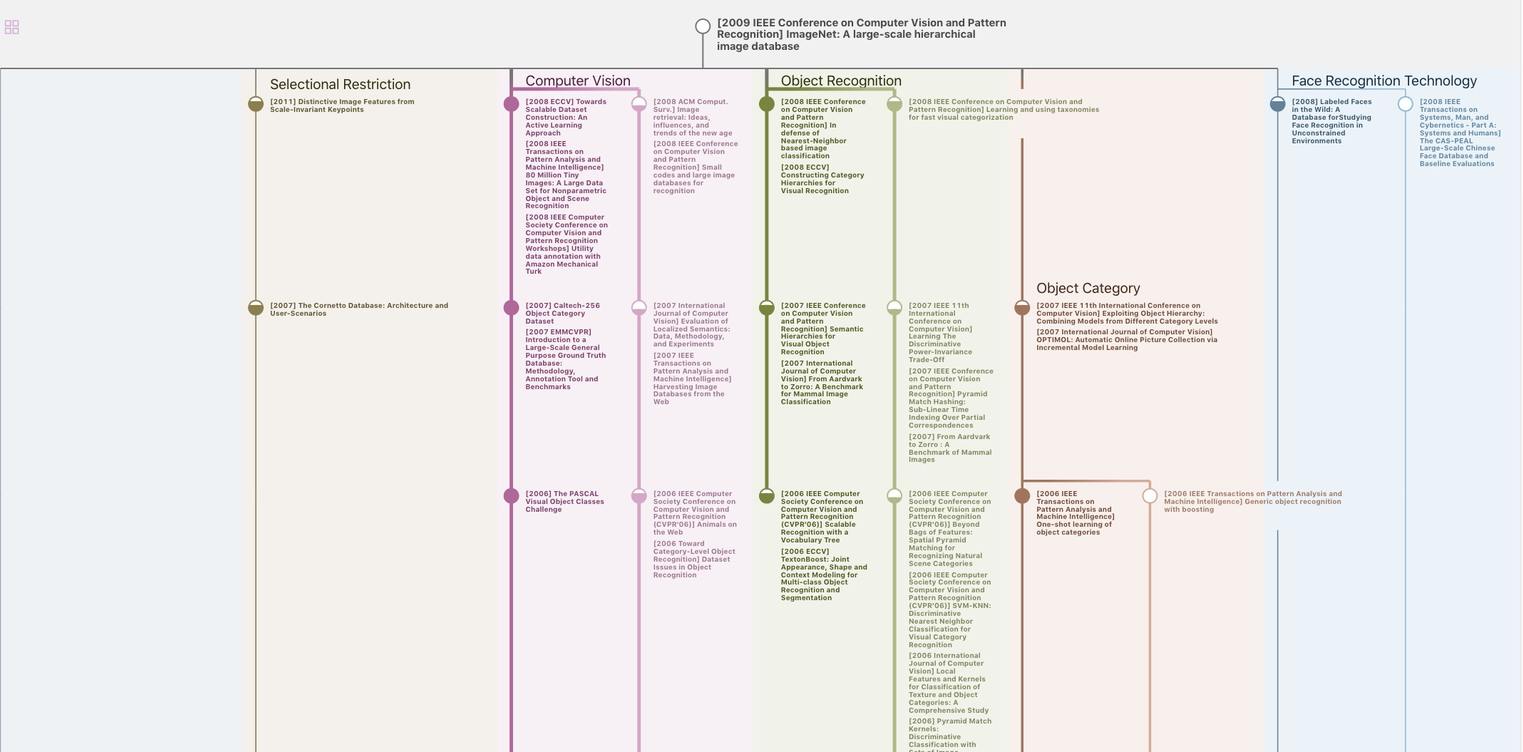
生成溯源树,研究论文发展脉络
Chat Paper
正在生成论文摘要