Multi-instance Randomness Extraction and Security Against Bounded-Storage Mass Surveillance
THEORY OF CRYPTOGRAPHY, TCC 2023, PT III(2023)
摘要
Consider a state-level adversary who observes and stores large amounts of encrypted data from all users on the Internet, but does not have the capacity to store it all. Later, it may target certain "persons of interest" in order to obtain their decryption keys. We would like to guarantee that, if the adversary's storage capacity is only (say) 1% of the total encrypted data size, then even if it can later obtain the decryption keys of arbitrary users, it can only learn something about the contents of (roughly) 1% of the ciphertexts, while the rest will maintain full security. This can be seen as an extension of incompressible cryptography (Dziembowski CRYPTO'06, Guan, Wichs and Zhandry EUROCRYPT'22) to the multi-user setting. We provide solutions in both the symmetric key and public key setting with various trade-offs in terms of computational assumptions and efficiency. As the core technical tool, we study an information-theoretic problem which we refer to as "multi-instance randomness extraction". Suppose X-1,..., X-t are correlated random variables whose total joint min-entropy rate is alpha, but we know nothing else about their individual entropies. We choose t random and independent seeds S-1,..., S-t and attempt to individually extract some small amount of randomness Y-i = Ext(X-i; S-i) from each X-i. We'd like to say that roughly an alpha-fraction of the extracted outputs Y-i should be indistinguishable from uniform even given all the remaining extracted outputs and all the seeds. We show that this indeed holds for specific extractors based on Hadamard and Reed-Muller codes.
更多查看译文
AI 理解论文
溯源树
样例
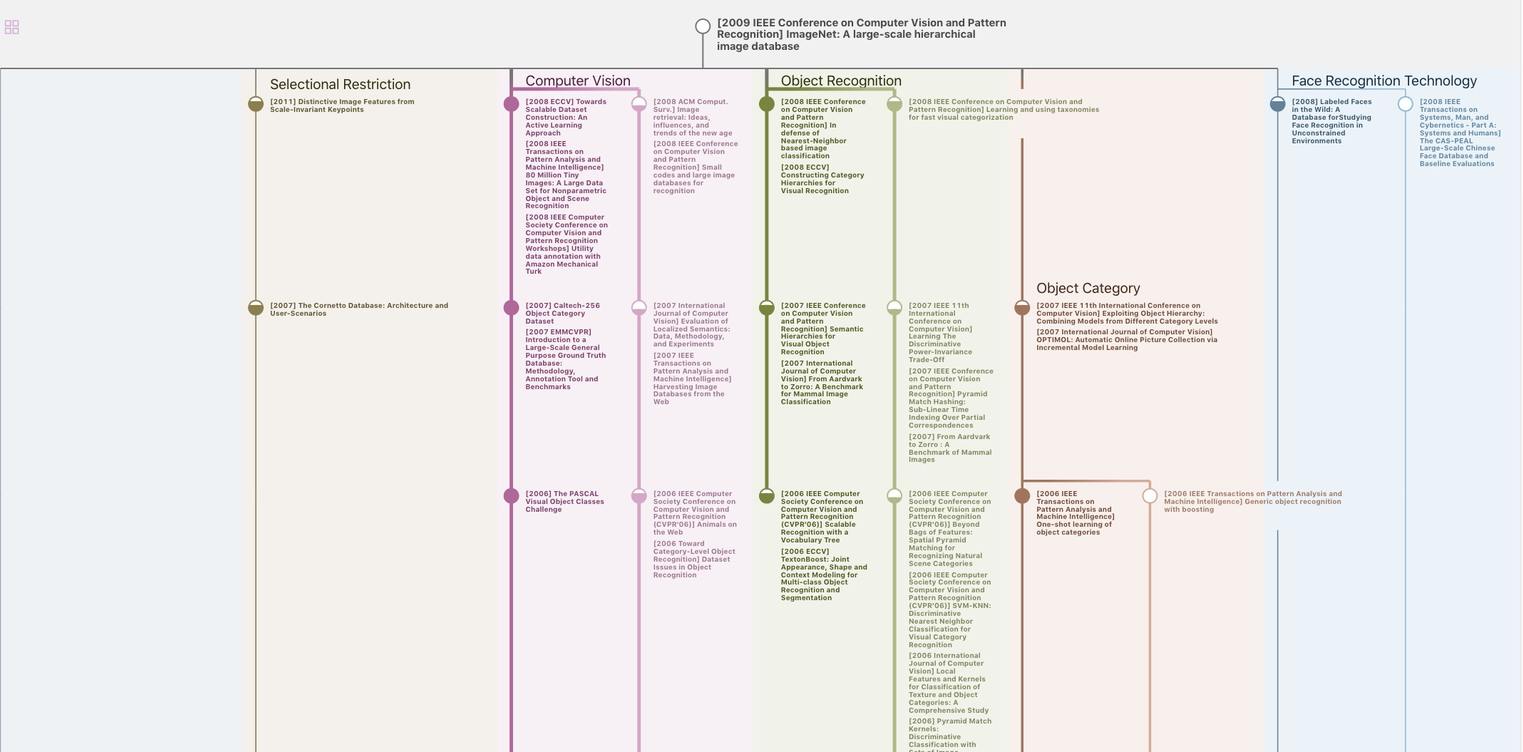
生成溯源树,研究论文发展脉络
Chat Paper
正在生成论文摘要