Toward a Unified Framework for RGB and RGB-D Visual Navigation
ADVANCES IN ARTIFICIAL INTELLIGENCE, AI 2023, PT II(2024)
摘要
In object-goal navigation, an agent is steered toward a target object based on its observations. The solution pipeline is usually composed of scene representation learning and navigation policy learning: the former reflects the agent observation, and the latter determines the navigation action. To this end, this article proposes a unified visual navigation framework, dubbed VTP, which can employ either RGB or RGBD observations for object-goal visual navigation. Using a unified Visual Transformer Navigation network (VTN), the agent analyzes image areas in relation to specific objects, producing visual representations that capture both instance-to-instance relationships and instance-to-region relationships. Meanwhile, we utilize depth maps to explore the spatial relationship between instances and the agent. Additionally, we develop a pre-training scheme to associate visual representations with navigation signals. Furthermore, we adopt Tentative Policy Learning (TPL) to guide an agent to escape from deadlocks. When an agent is detected as being in deadlock states in training, we utilize Tentative Imitation learning (TIL) to provide the agent expert demonstrations for deadlock escape and such demonstrations are learned in a separate Tentative Policy Network (TPN). In testing, under deadlocks, estimated expert demonstrations are given to the policy network to find an escape action. Our system outperforms other methods in both iTHOR and RoboTHOR.
更多查看译文
关键词
Visual Navigation,Reinforcement Learning,Vision Transformer
AI 理解论文
溯源树
样例
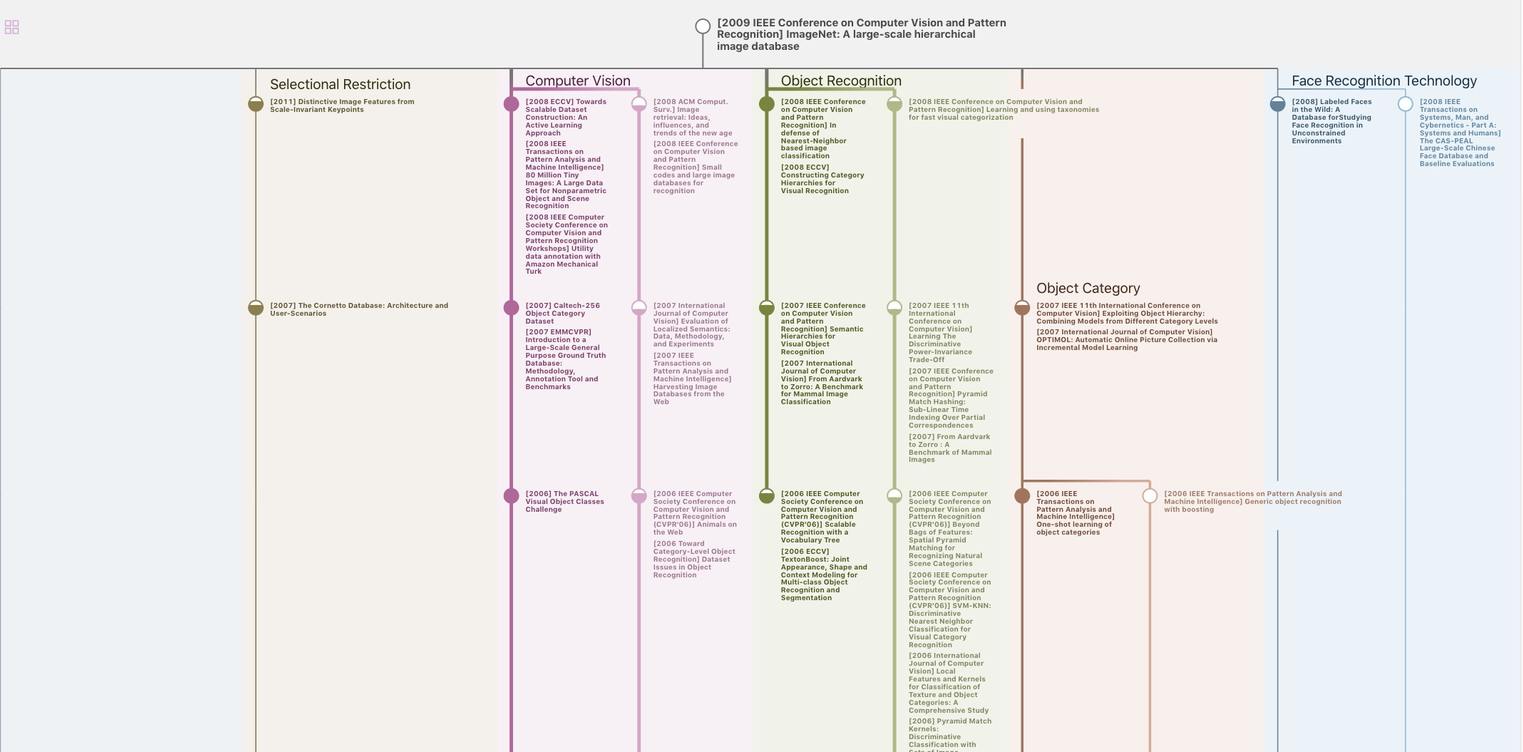
生成溯源树,研究论文发展脉络
Chat Paper
正在生成论文摘要