Causal Disentanglement for Adversarial Defense
ADVANCES IN ARTIFICIAL INTELLIGENCE, AI 2023, PT I(2024)
摘要
Representation learning that seeks the high accuracy of a classifier is a key contribute to the success of state-of-the-art DNNs. However, DNNs face the threat of adversarial attacks and their robustness is in peril. While the adversarial defense has been widely studied, much of the research is based on a statistical association and causality based defense approach is a relatively open area. We present CDAD (Causal Disentanglement for Adversarial Defense), a novel defense method that learns and utilizes causal representations for robust prediction. We take inspiration from a recent study that takes a causal perspective on the adversarial problem and considers the susceptibility of DNNs to adversarial examples come from their reliance on spurious associations between non-causal features and labels, such that an adversary exploits the associations to succeed in the attack. Causal representations are robust as the causal relationship between a cause of the label and the label is invariant under different environments. However, discovering causal representations is a challenging task, especially in the context of image data. Harnessing the recent advancement in representation learning with VAE (Variational AutoEncoder), we design CDAD as a VAE based causal disentanglement representation learning method to decouple causal and non-causal representations. CADA uses the invariance property of causal features as a constraint in the disentanglement of causal features and non-causal features. Experimental results show CDAD's highly competitive performance compared to the state-of-the-art defense methods, while possessing a causal foundation.
更多查看译文
关键词
Causality,Adversarial machine learning,Representations
AI 理解论文
溯源树
样例
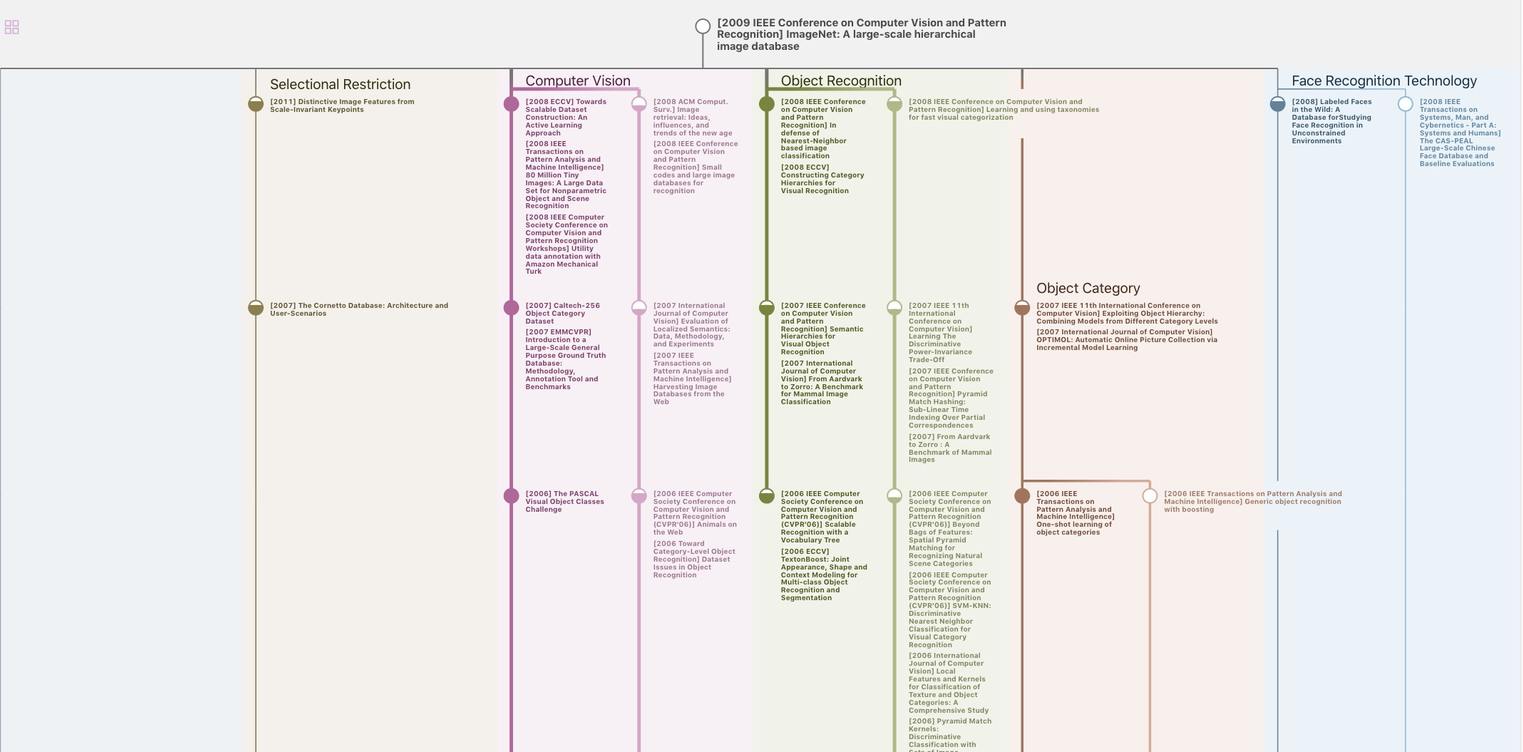
生成溯源树,研究论文发展脉络
Chat Paper
正在生成论文摘要