Self-supervised Monocular Depth Estimation on Unseen Synthetic Cameras
PROGRESS IN PATTERN RECOGNITION, IMAGE ANALYSIS, COMPUTER VISION, AND APPLICATIONS, CIARP 2023, PT I(2024)
摘要
Monocular depth estimation is a critical task in computer vision, and self-supervised deep learning methods have achieved remarkable results in recent years. However, these models often struggle on camera generalization, i.e. at sequences captured by unseen cameras. To address this challenge, we present a new public custom dataset created using the CARLA simulator [4], consisting of three video sequences recorded by five different cameras with varying focal distances. This dataset has been created due to the absence of public datasets containing identical sequences captured by different cameras. Additionally, it is proposed in this paper the use of adversarial training to improve the models' robustness to intrinsic camera parameter changes, enabling accurate depth estimation regardless of the recording camera. The results of our proposed architecture are compared with a baseline model, hence being evaluated the effectiveness of adversarial training and demonstrating its potential benefits both on our synthetic dataset and on the KITTI benchmark [8] as the reference dataset to evaluate depth estimation.
更多查看译文
关键词
Monocular Depth Estimation,Computer Vision,Self-Supervised Learning,Camera Generalization,Custom Synthetic Dataset,Adversarial Training
AI 理解论文
溯源树
样例
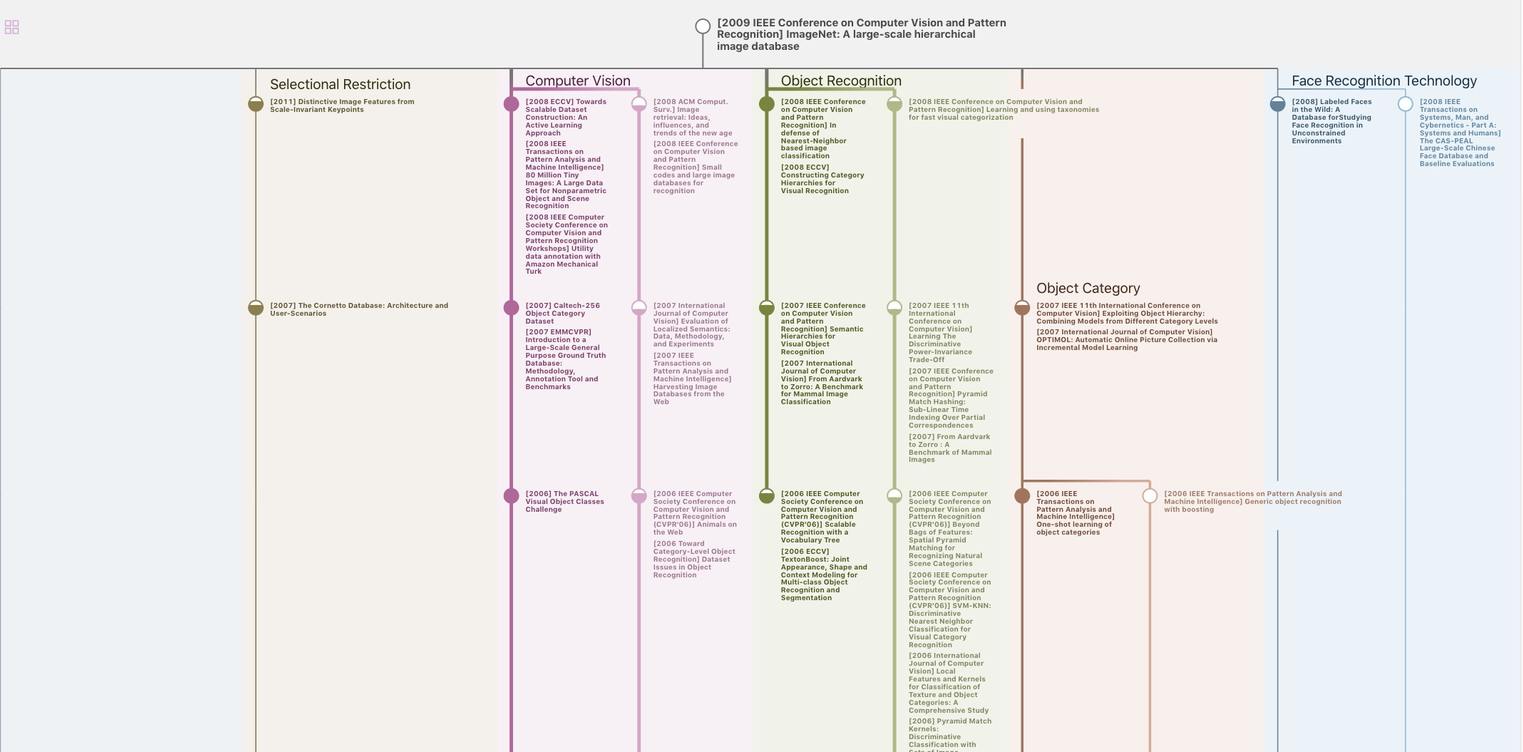
生成溯源树,研究论文发展脉络
Chat Paper
正在生成论文摘要