Leveraging Longitudinal Data for Cardiomegaly and Change Detection in Chest Radiography
PROGRESS IN PATTERN RECOGNITION, IMAGE ANALYSIS, COMPUTER VISION, AND APPLICATIONS, CIARP 2023, PT I(2024)
摘要
Chest radiography has been widely used for automatic analysis through deep learning (DL) techniques. However, in the manual analysis of these scans, comparison with images at previous time points is commonly done, in order to establish a longitudinal reference. The usage of longitudinal information in automatic analysis is not a common practice, but it might provide relevant information for desired output. In this work, the application of longitudinal information for the detection of cardiomegaly and change in pairs of CXR images was studied. Multiple experiments were performed, where the inclusion of longitudinal information was done at the features level and at the input level. The impact of the alignment of the image pairs (through a developed method) was also studied. The usage of aligned images was revealed to improve the final mcs for both the detection of pathology and change, in comparison to a standard multi-label classifier baseline. The model that uses concatenated image features outperformed the remaining, with an Area Under the Receiver Operating Characteristics Curve (AUC) of 0.858 for change detection, and presenting an AUC of 0.897 for the detection of pathology, showing that pathology features can be used to predict more efficiently the comparison between images. In order to further improve the developed methods, data augmentation techniques were studied. These proved that increasing the representation of minority classes leads to higher noise in the dataset. It also showed that neglecting the temporal order of the images can be an advantageous augmentation technique in longitudinal change studies.
更多查看译文
关键词
Classification,Deep Learning,Longitudinal Image Pairs,Pathology,Thorax
AI 理解论文
溯源树
样例
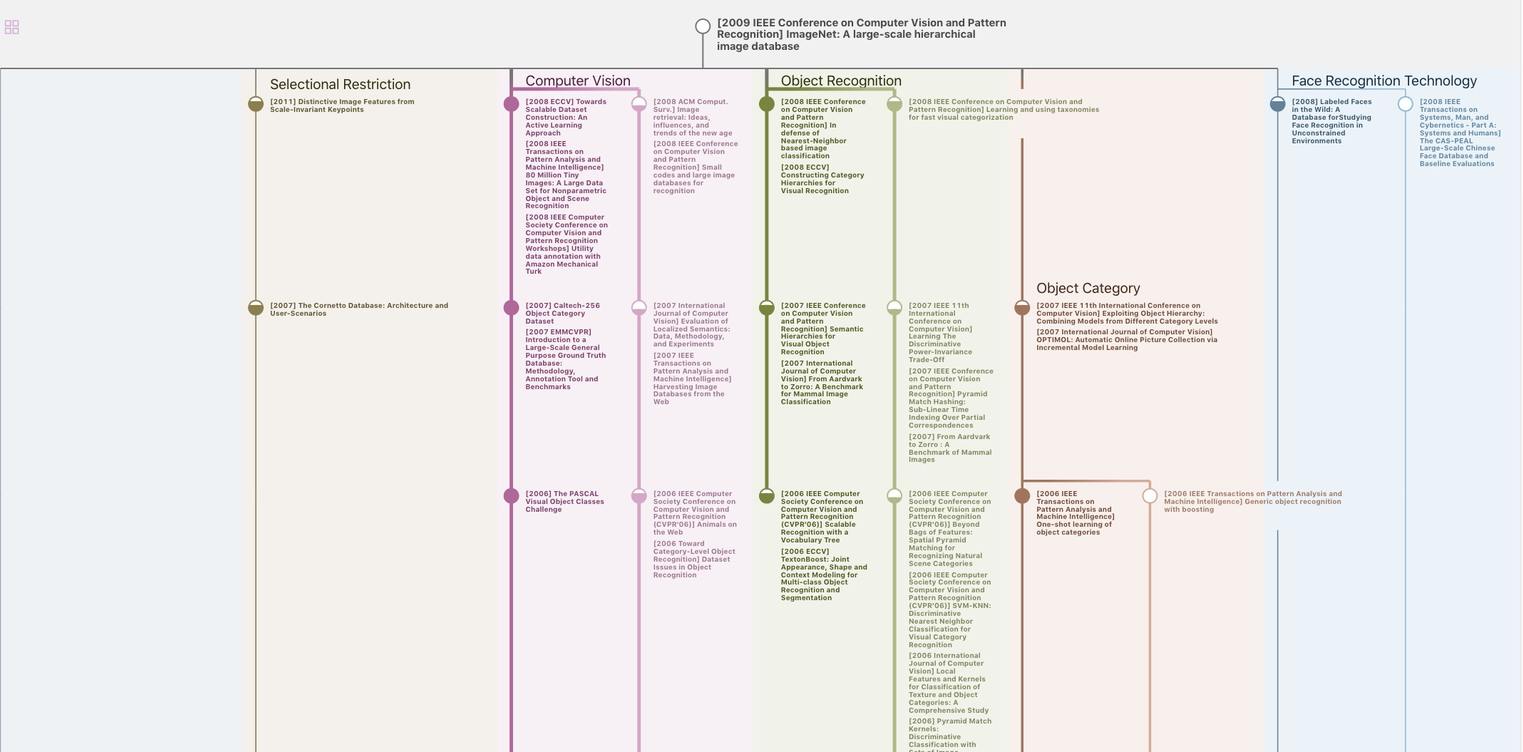
生成溯源树,研究论文发展脉络
Chat Paper
正在生成论文摘要