Vehicle Re-Identification Based on Unsupervised Domain Adaptation by Incremental Generation of Pseudo-Labels
PROGRESS IN PATTERN RECOGNITION, IMAGE ANALYSIS, COMPUTER VISION, AND APPLICATIONS, CIARP 2023, PT I(2024)
摘要
The main goal of vehicle re-identification (ReID) is to associate the same vehicle identity in different cameras. This is a challenging task due to variations in light, viewpoints or occlusions; in particular, vehicles present a large intra-class variability and a small inter-class variability. In ReID, the samples in the test sets belong to identities that have not been seen during training. To reduce the domain gap between train and test sets, this work explores unsupervised domain adaptation (UDA) generating automatically pseudo-labels from the testing data, which are used to fine-tune the ReID models. Specifically, the pseudo-labels are obtained by clustering using different hyperparameters and incrementally due to retraining the model a number of times per hyperparameter with the generated pseudo-labels. The ReID system is evaluated in CityFlow-ReID-v2 dataset.
更多查看译文
关键词
Vehicle re-identification,Unsupervised Domain Adaptation,Pseudo-labels,Deep learning,Image processing,Surveillance videos
AI 理解论文
溯源树
样例
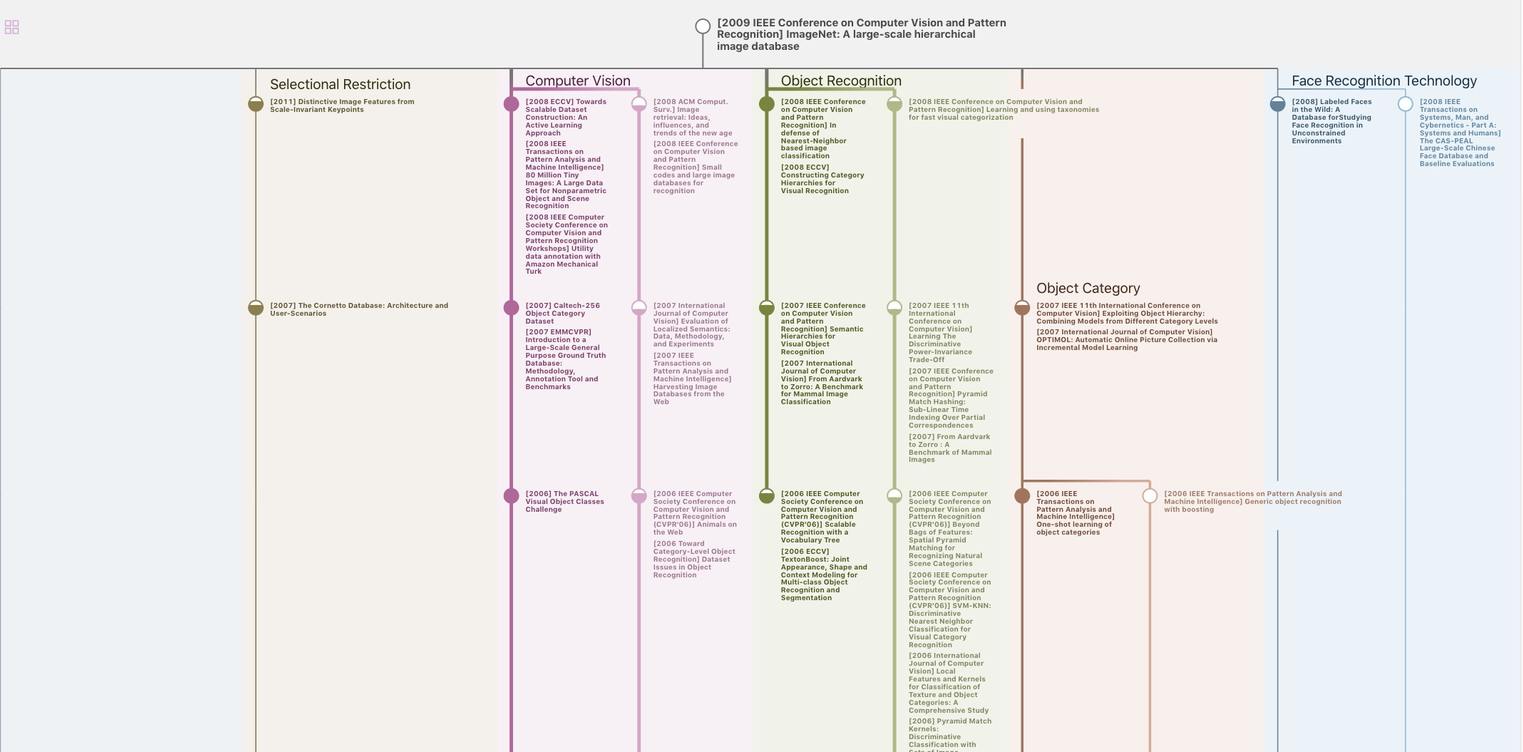
生成溯源树,研究论文发展脉络
Chat Paper
正在生成论文摘要