R-IoM: Enhance Biometric Security with Redundancy-Reduced Hashcode Reliability
BIOMETRIC RECOGNITION, CCBR 2023(2023)
摘要
This research focuses on analyzing vulnerabilities in the IoM hashing technique concerning its susceptibility to preimage attacks, which poses a significant security concern in biometric template protection (BTP) systems. To address these vulnerabilities and achieve a balanced trade-off between performance and security, we propose a novel approach called R-IoM hashing. This method introduces innovative mitigation strategies to minimize information leakage and enhance the resistance to preimage attacks. By employing a dimensionality reduction technique during the IoM hashing process, R-IoM hashing effectively eliminates extraneous data, ensuring improved security without compromising computational efficiency. Through comprehensive experiments, we demonstrate the effectiveness of R-IoM hashing. Notably, this approach maintains remarkably low error rates while showcasing robust resilience against preimage attacks compared to conventional IoM hashing. This substantial improvement in security positions R-IoM hashing as a promising solution for real-world BTP applications. Our research underscores the importance of accurate distance measurement in feature comparison, identifies vulnerabilities in IoM hashing, and introduces a practical and efficient solution through R-IoM hashing to enhance the reliability and security of biometric-based systems. This paper contributes valuable insights into biometric template protection and offers a potential avenue for future research and implementation in security-critical environments.
更多查看译文
AI 理解论文
溯源树
样例
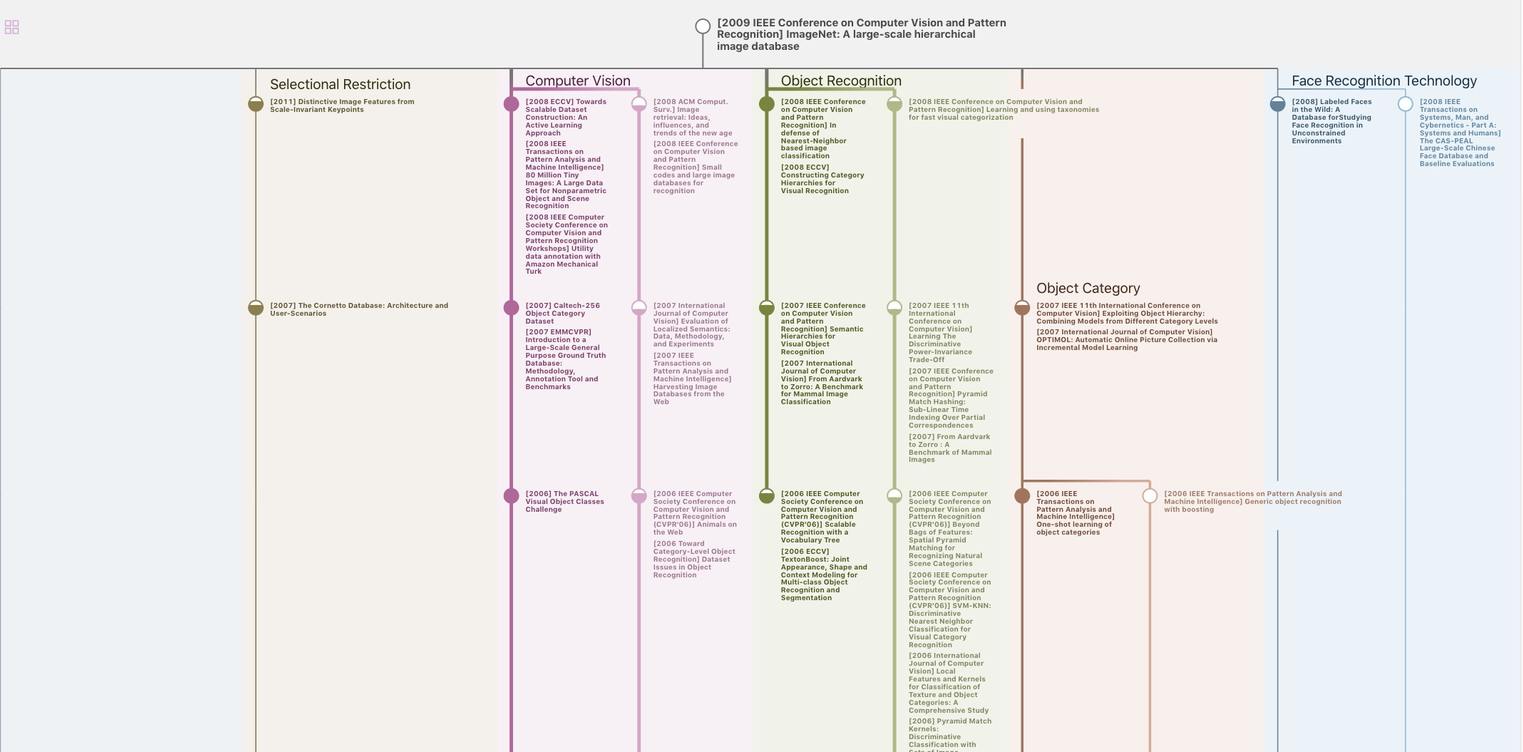
生成溯源树,研究论文发展脉络
Chat Paper
正在生成论文摘要