Revolutionizing Cancer Diagnosis Through Hybrid Self-supervised Deep Learning: EfficientNet with Denoising Autoencoder for Semantic Segmentation of Histopathological Images
MEDICAL IMAGE UNDERSTANDING AND ANALYSIS, MIUA 2023(2024)
摘要
Machine Learning technologies are being developed day after day, especially in the medical field. New approaches, algorithms and architectures are implemented to increase the efficiency and accuracy of diagnosis and segmentation. Deep learning approaches have proven their efficiency; these approaches include architectures like EfficientNet and Denoising Autoencoder. Accurate segmentation of nuclei in histopathological images is essential for the diagnosis and prognosis of diseases like cancer. In this paper, we propose a novel method for semantic segmentation of nuclei using EfficientNet and Denoising Auto-encoder on the PanNuke dataset. The denoising auto-encoder pre-processing step is used to enhance the feature representations of input images, and EfficientNet is the model that has been used as the semantic segmentation model. Our proposed method achieved state-of-the-art results, outperforming most of the previously proposed methods by a significant margin, as our proposed method achieved a higher Dice score of 83.33 compared to the previous related work methods, which achieved scores varying from 69.3 to 80.28. The efficiency of our proposed approach will be demonstrated in this paper by discussing, exploring, and comparing it with previously proposed methods and their results.
更多查看译文
关键词
semantic segmentation,histopathological images,self-supervised deep learning,EfficientNet,Denoising Autoencoder
AI 理解论文
溯源树
样例
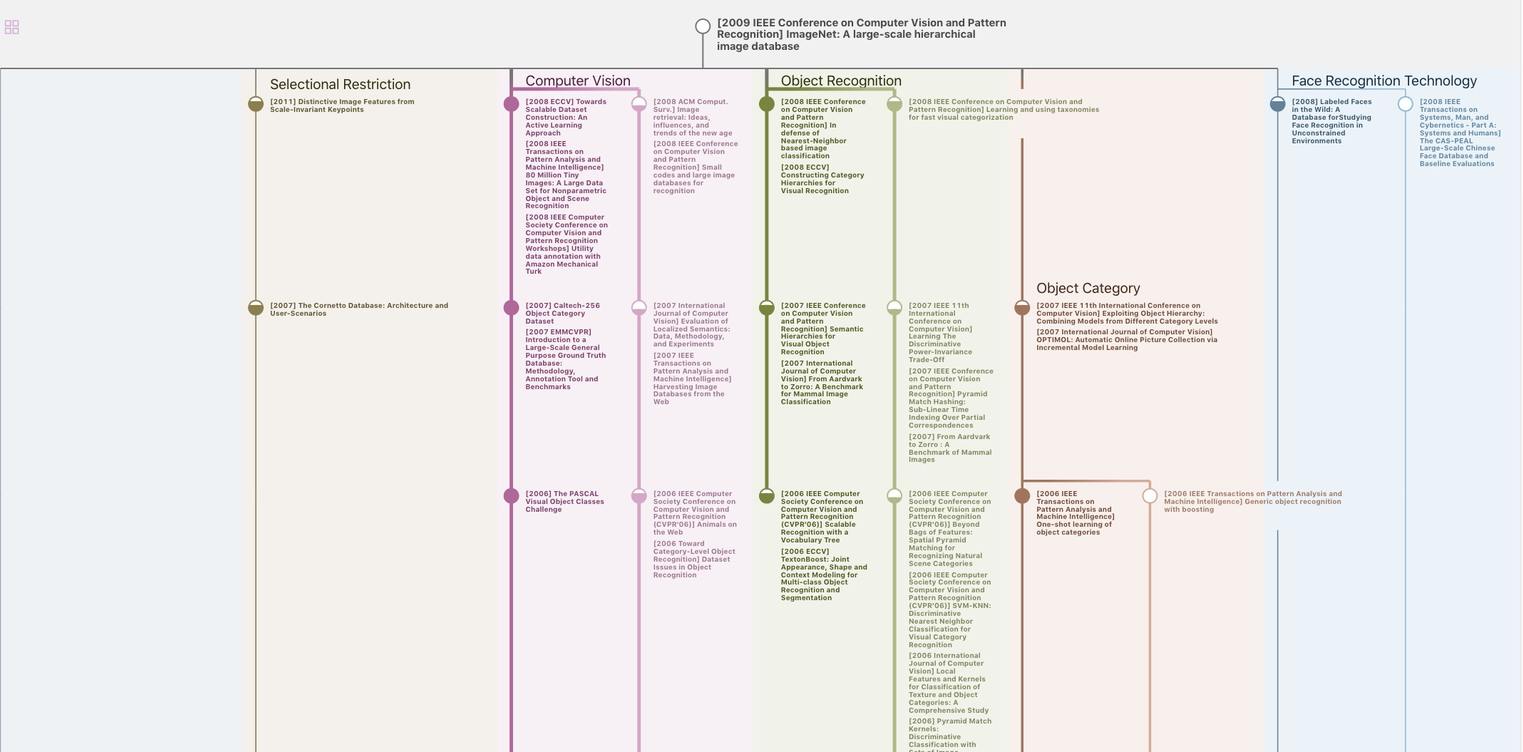
生成溯源树,研究论文发展脉络
Chat Paper
正在生成论文摘要