Context Matters: Cross-Domain Cell Detection in Histopathology Images via Contextual Regularization
MEDICAL IMAGE UNDERSTANDING AND ANALYSIS, MIUA 2023(2024)
摘要
Deep learning-based cell detectors have shown promise in automating cell detection in histopathology images, which can aid in cancer diagnosis and prognosis. Nevertheless, the color variation in stain appearance across histopathology images obtained from diverse locations might degrade the accuracy of cell detection. The limitations of a basic cell detector on a dissimilar target dataset are attributed to its emphasis on domain-specific features while overlooking domain-invariant features. Thus, a cell detector that is trained on a particular source dataset may not perform optimally on a different target dataset. In this work, we propose a domain generalization method contextual regularization (CR) for cell detection in histopathology images, which is derived from the basic object detector and focuses on domain-invariant features to enhance detection performance across varying datasets. Specifically, we involves a reconstruction task that involves masking the high-level semantic features either stochastically or adaptively. Then, a transformer-based reconstruction head is designed to recover the original features based on partial observations. Additionally, CR can be seamlessly integrated with various deep learning-based cell detectors without further modifications, and it does not request additional cost in the inference time. The proposed method was validated on a publicly available dataset that comprises histopathology images acquired at different sites, and the results show that our method can effectively improve the generalization of cell detectors to unseen domains.
更多查看译文
关键词
Cell detection,Domain Generalization,Contextual regularization
AI 理解论文
溯源树
样例
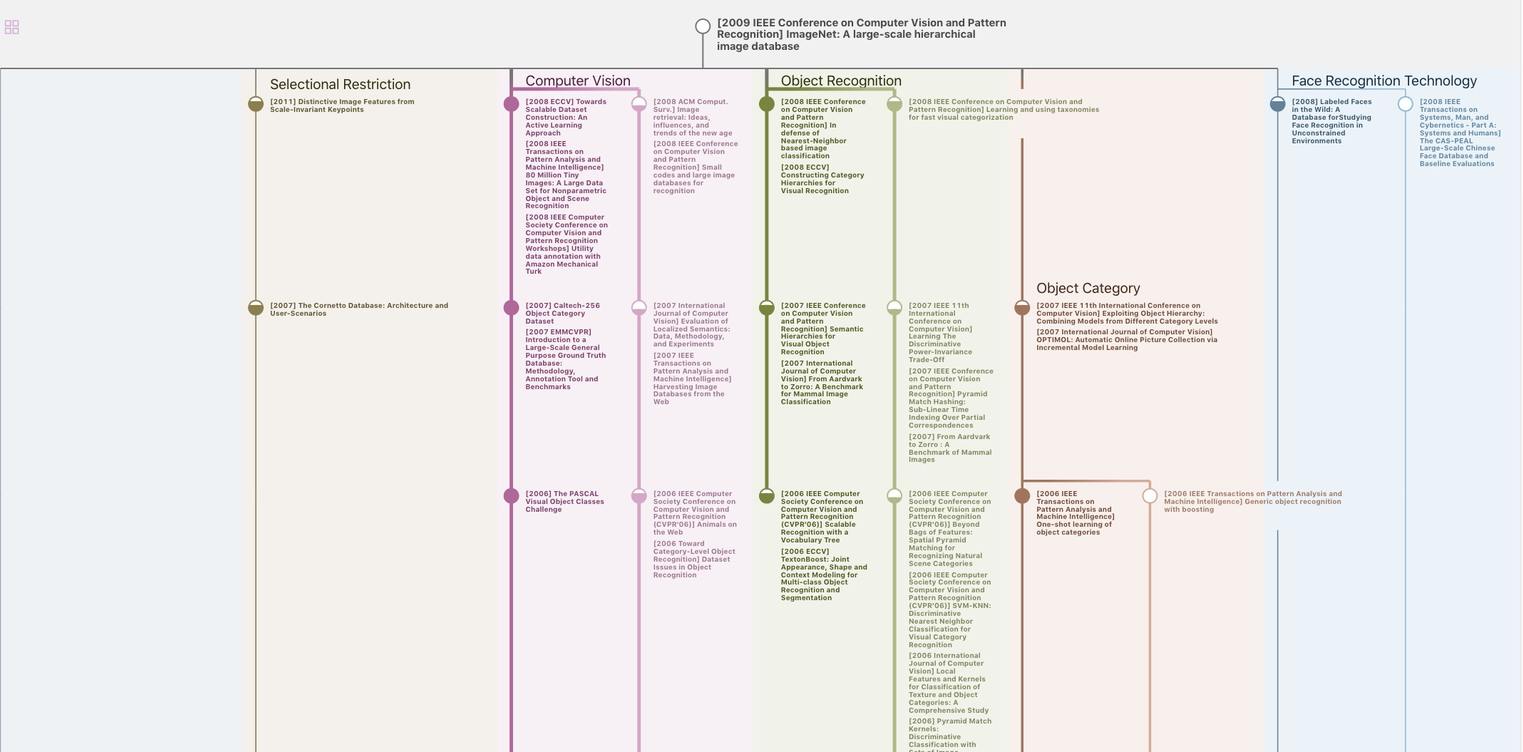
生成溯源树,研究论文发展脉络
Chat Paper
正在生成论文摘要