Soft imitation reinforcement learning with value decomposition for portfolio management
APPLIED SOFT COMPUTING(2024)
摘要
Imitation learning has been recognized as a method to accelerate the training process of deep reinforcement learning agents in search of optimal strategies. Nevertheless, existing imitation learning algorithms have limitations in effectively leveraging expert demonstrations when confronted with dynamic environments, as the behavior cloning loss weight cannot be adaptively updated. To overcome this challenge, we propose a novel approach called Soft Imitation Reinforcement Learning (SIRL), which combines imitation learning and reinforcement learning to guide the training of reinforcement learning agents in an adaptive manner. Additionally, we addressed the challenge of high-dimensional action spaces for reinforcement learning in portfolio management with value decomposition, and provide theoretical proof of convergence for this method. To validate the effectiveness of the SIRL algorithm, we conduct extensive experiments using stock market data from emerging (China) and developed (the US) countries. Our experiments indicate the versatility of the proposed SIRL across different types of trading data, encompassing both high-frequency (5-minute interval) and low-frequency (daily and weekly) data.
更多查看译文
关键词
Imitation learning,Deep reinforcement learning,Soft Imitation Reinforcement Learning,Portfolio management
AI 理解论文
溯源树
样例
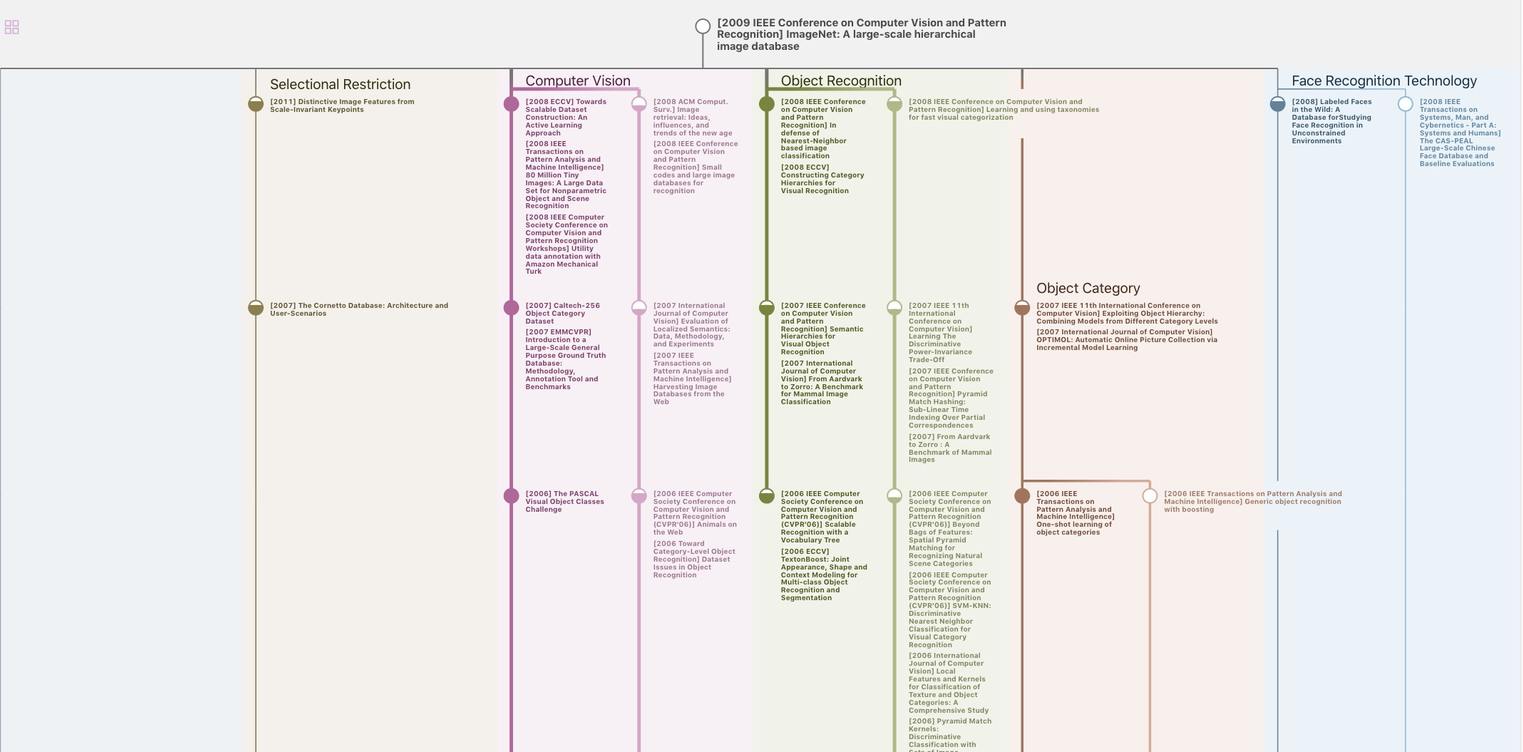
生成溯源树,研究论文发展脉络
Chat Paper
正在生成论文摘要