Evaluating data-driven and hybrid modeling of terrestrial actual evapotranspiration based on an automatic machine learning approach
JOURNAL OF HYDROLOGY(2024)
摘要
The performances of physics-based, data-driven, and hybrid models for estimating terrestrial actual evapo-transpiration (ETa) is currently under debate, which requires thorough evaluations of those models particularly with recent developments in automatic machine learning (AML) techniques. In this study, six AML-based models were first constructed using the H2O-AML platform, from which an optimal (AML-OP) model was selected for estimating daily ETa at ecosystem scales. In addition, hybrid models were developed by combining the six AML models with surface conductance (Gs) inverted from the Penman-Monteith equation and an optimal (PM-OP) model was also selected. With 15 predictor variables as model inputs that were compiled from various data sources, the performances of those models for estimating daily ETa were evaluated using observed data from the FLUXNET2015 dataset. The results revealed that no models showed consistently low noise levels across different ecosystem types, making it necessary to use AML techniques for selecting ecosystem-specific models. Interest-ingly, the AML-OP models (root mean square error (RMSE) and symmetric mean absolute percentage error (SMAPE) were 0.16-0.31 mm d-1 and 9 %-36 % respectively) showed slightly better predictive results than the PM-OP models (RMSE and SMAPE were 0.23-0.36 mm d-1 and 15 %-68 % respectively), likely owing to model parameter uncertainties and tight constraints of physical models on application condition. Secondly, as ETa nonlinearly responds to environmental variables, model predictability under extreme weather (drought and heatwave) conditions was examined. The results showed that the prediction of the AML-OP and PM-OP models expectedly worsened (RMSE and SMAPE increased by 0.06-0.77 mm d-1 and-19 % to 79 %, respectively); however, the AML-OP model still outperformed the PM-OP model in most ecosystems, further underscoring the need to understand ETa regulation mechanisms under varying climatic conditions. Finally, the PM-OP models developed here provided better daily ETa estimates compared to other recently proposed hybrid models (RMSE reduced by 0.98-1.80 mm d-1). Both models can be better applied to wetlands that have been less frequently evaluated previously (RMSE reduction of 0.22 mm d-1 and 0.18 mm d-1 for the AML-OP and PM-OP models).
更多查看译文
关键词
Actual evapotranspiration,Terrestrial ecosystem,Automatic machine learning,Data-driven,Hybrid modeling,FLUXNET
AI 理解论文
溯源树
样例
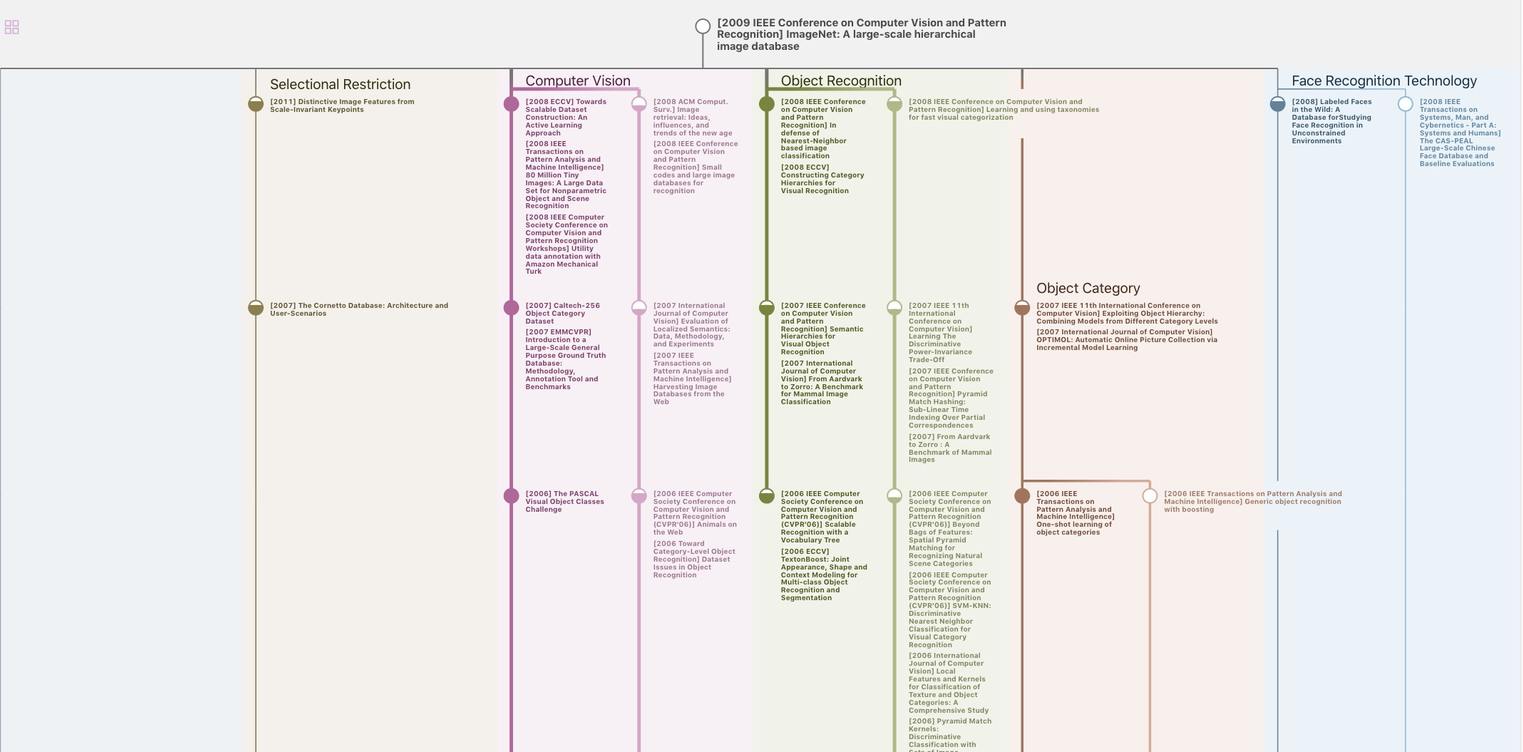
生成溯源树,研究论文发展脉络
Chat Paper
正在生成论文摘要