Development of machine learning interatomic potential for zinc
COMPUTATIONAL MATERIALS SCIENCE(2024)
摘要
The HCP structure of zinc (Zn) exhibits an anomalous c/a ratio, a factor that traditional empirical potentials find challenging to reproduce accurately, thus impeding thorough investigations of its property and behavior via realistic atomistic simulations. The advent of machine learning interatomic potentials in recent years has ushered in new opportunities for the development of reliable Zn potentials. In this study, we construct a large set of atomic configurations composed of Zn atoms and calculate their energy, atomic forces, and stress tensor through high-throughput density functional theory calculations. Based on the calculated data, a moment tensor potential for Zn is developed, whose fidelity is then thoroughly examined by comprehensive comparison to existing potentials from the literature, density function theory calculations, and/or experimental measurements. It is found that the constructed potential can accurately reproduce the c/a ratio of HCP Zn, the relative stability of different crystal phases, the elastic properties, and defect properties of Zn. It can also describe reliably the structure, thermodynamic, and kinetic properties of Zn melt. This potential therefore offers a reliable tool for further exploration of the property and behavior of Zn at the atomic scale.
更多查看译文
关键词
Machine learning interatomic potential,Zinc,Density functional theory,Molecular dynamics simulation
AI 理解论文
溯源树
样例
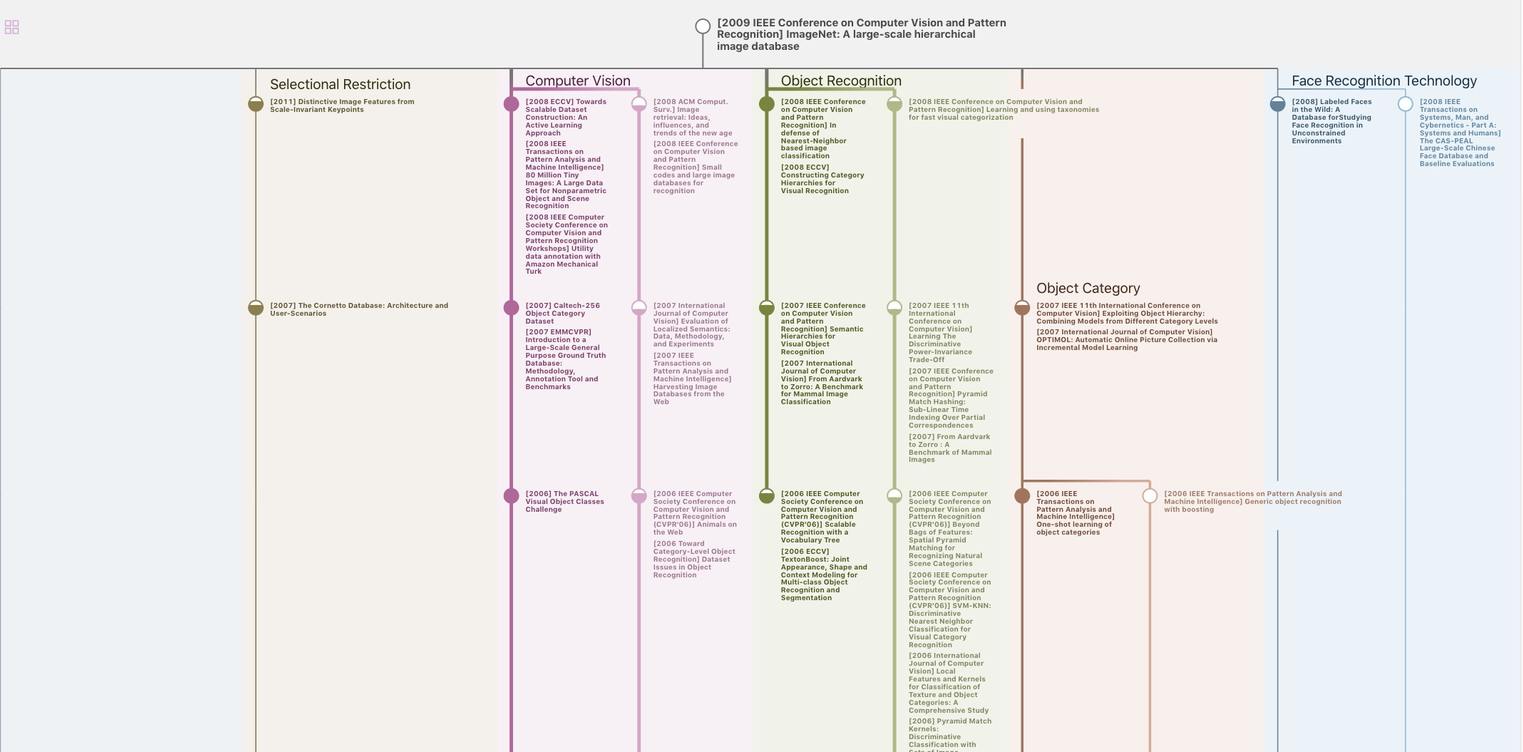
生成溯源树,研究论文发展脉络
Chat Paper
正在生成论文摘要