Mean-field reinforcement learning for decentralized task offloading in vehicular edge computing
JOURNAL OF SYSTEMS ARCHITECTURE(2024)
摘要
Vehicular Edge Computing (VEC) is a promising paradigm for providing low-latency and high-reliability services in the Internet of Vehicles (IoV). The increasing number of mobile devices and the diverse resource requirements of the growing IoV have resulted in a shift from centralized resource management to a decentralized approach. This shift offers improved fault tolerance, scalability, and privacy preservation. However, constructing collaborative awareness and coordination mechanisms between multiple vehicles and edge nodes in a decentralized manner is a challenge. To address this issue, we propose a decentralized many to-many task offloading method that aims to minimize the average task completion latency of vehicles. In this study, we propose a data-sharing mechanism between vehicles and edge servers using the digital twin service, which provides global environmental perceptions to the vehicles by a low-cost approach. Additionally, we develop a mean-field multi-agent reinforcement learning algorithm to generate coordinated task offloading schemes. Instead of interacting with multiple agents, the vehicle only needs to respond to the mean action of the environment. Based on this transition, the agent generates coordinated task offloading decisions in complex scenarios. We evaluate the performance of our method using real urban traffic data. Experiment results verify the efficiency of our proposed method.
更多查看译文
关键词
Vehicular edge computing,Mean-field reinforcement learning,Digital twin,Task offloading
AI 理解论文
溯源树
样例
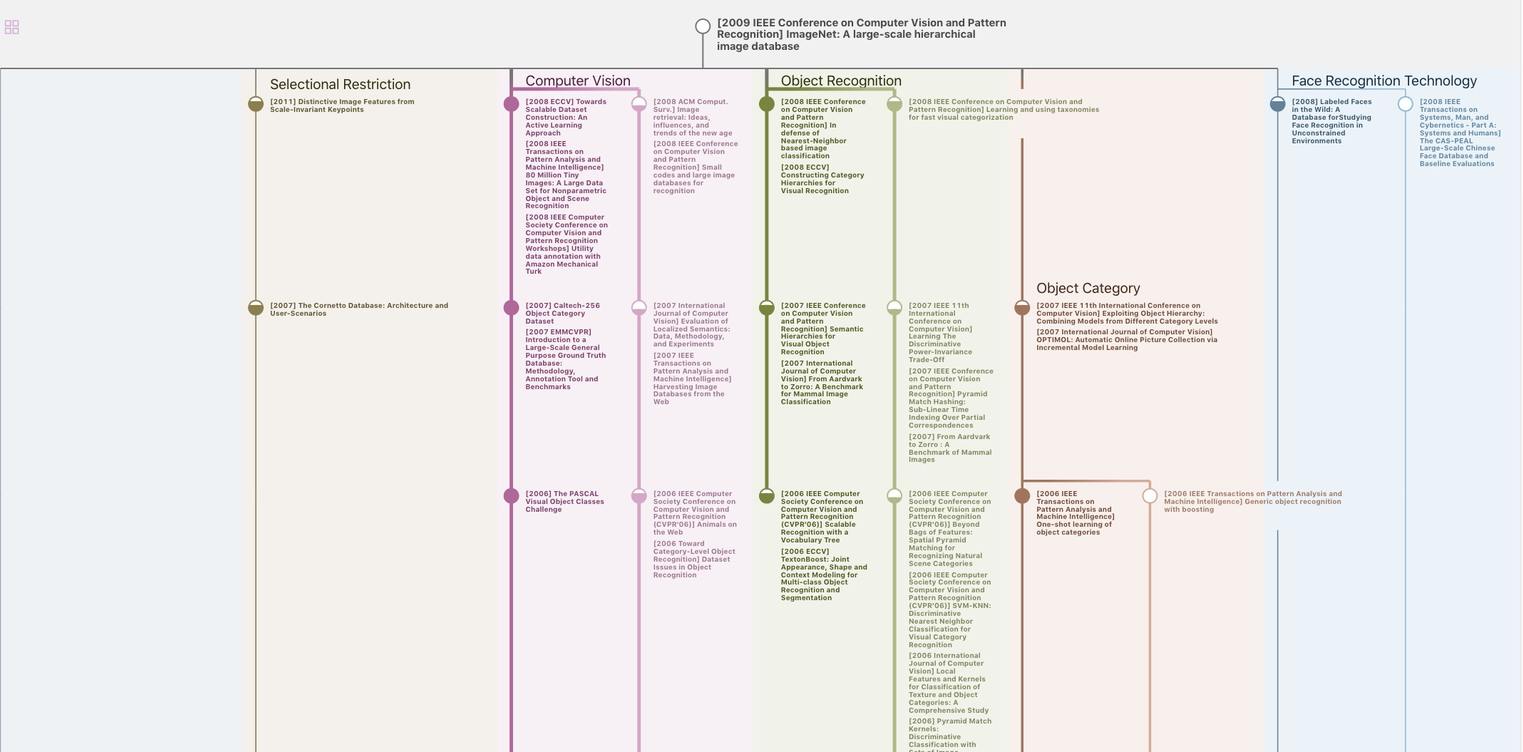
生成溯源树,研究论文发展脉络
Chat Paper
正在生成论文摘要