A two-stage model extraction attack on GANs with a small collected dataset
COMPUTERS & SECURITY(2024)
摘要
Due to their capacity for image generation, GAN models may be considered as a solution for the use of private data, which enhances their commercial value. However, unlike discriminative models such as CNNs, extraction attacks on GANs have not received significant attention, with only a few relevant works available. This paper proposes a novel two-stage extraction attack on GANs that does not require access to the victim GAN model. In the first stage, data augmentation is performed using GAN inversion techniques and vector arithmetic, tailored to scenarios with small query budgets. The second stage comprises two types of training: the innocent transfer training on unprocessed collected samples and the additional training on the augmented set. We provide three options for additional training: training of the discriminator alone (D), training of the generator alone (G), and training of both (D+G). We then conduct a quantity and quality evaluation of our extraction attacks compared to the baseline attack. Experiment results demonstrate that our proposed approach can successfully extract a GAN model, where the distribution of the extracted GAN closely resembles the distribution of the target GAN, even with a hundred generated images of the target GAN. Specifically, we achieve stable performance with better extraction, a more disentangled latent space for the extracted GAN, and significant semantic attribute editing performance using the two-stage extraction with additional training of the discriminator (D). By demonstrating that even a hundred leaked images may result in severe extraction, this study raises serious concerns regarding GAN model leakage.
更多查看译文
关键词
Model extraction attack,Generative adversarial networks,Data augmentation,Privacy leakage,Defense method
AI 理解论文
溯源树
样例
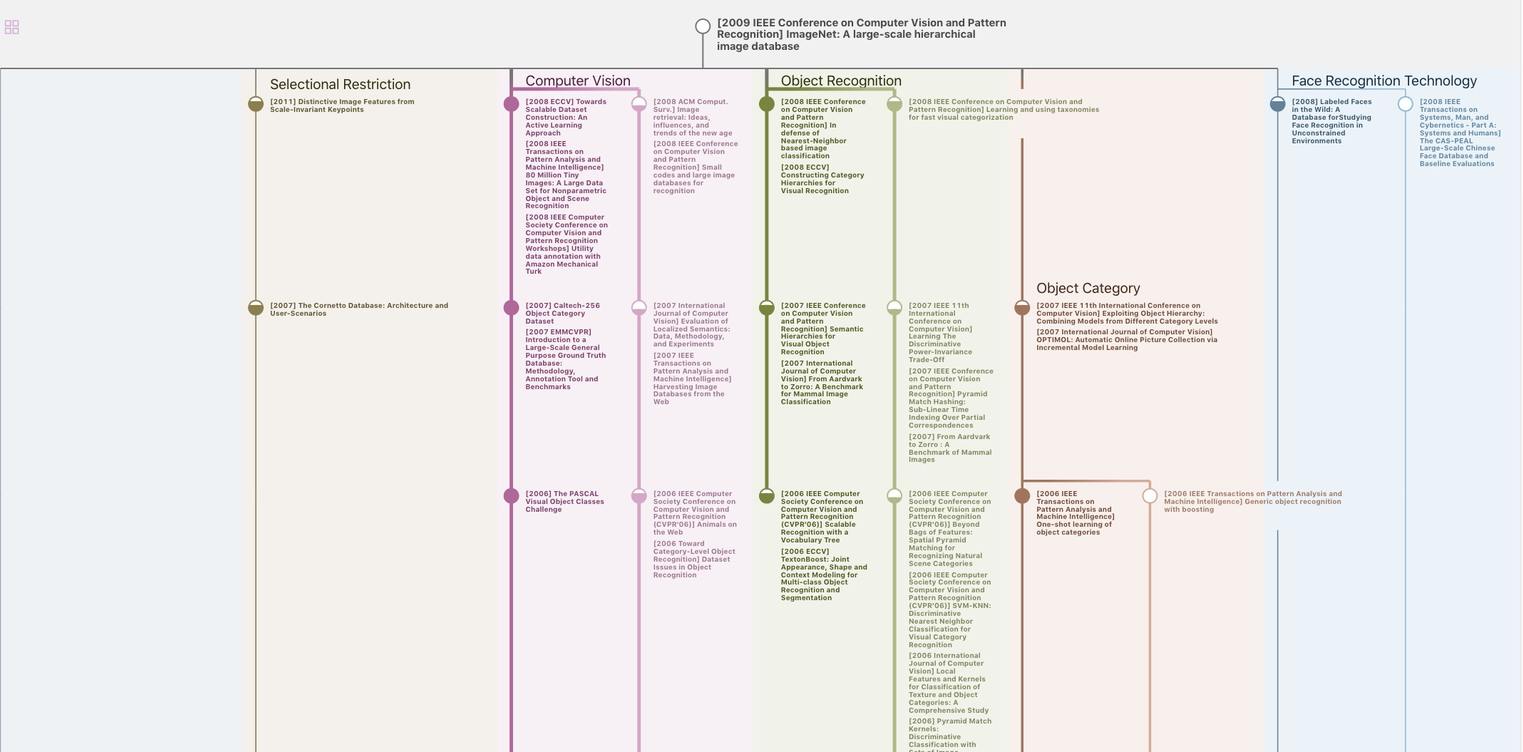
生成溯源树,研究论文发展脉络
Chat Paper
正在生成论文摘要