Optimized injection of noise in activation functions to improve generalization of neural networks
CHAOS SOLITONS & FRACTALS(2024)
摘要
This paper proposes a flexible probabilistic activation function that enhances the training and operation of artificial neural networks by intentionally injecting noise to gain additional control over the response of each neuron. During the learning phase, the level of injected noise is iteratively optimized by gradient-descent, realizing a form of adaptive stochastic resonance. From simple hard-threshold non-differentiable neuronal responses, controlled injection of noise gives access to a wide range of useful activation functions, with sufficient differentiability to enable gradient-descent learning for both the neuron and the injected-noise levels. Experimental results on function approximation demonstrate injected noise generally converging to non vanishing optimal levels associated with improved generalization abilities in the neural networks. A theoretical explanation of the generalization improvement based on the path norm bound is presented. With injected noise in the deep neural network, experimental results on classifying images also obtain non-vanishing optimal noise levels to achieve better testing accuracies. The proposed probabilistic activation functions show the potential of adaptive stochastic resonance for useful applications in machine learning.
更多查看译文
关键词
Probabilistic activation function,Adaptive stochastic resonance,Injected noise,Rademacher complexity,Generalization
AI 理解论文
溯源树
样例
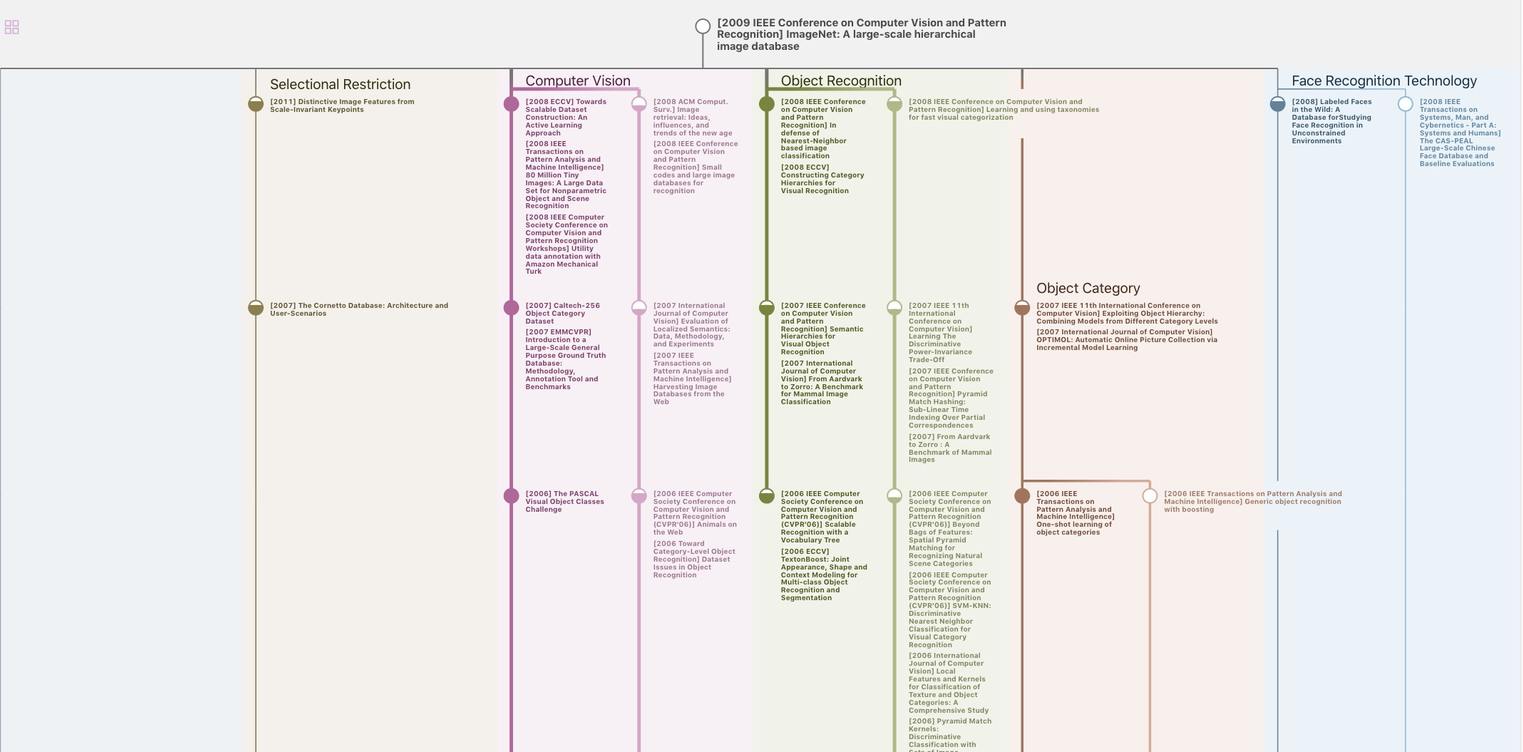
生成溯源树,研究论文发展脉络
Chat Paper
正在生成论文摘要