Global semantic-guided network for saliency prediction
KNOWLEDGE-BASED SYSTEMS(2024)
摘要
The human visual system effectively analyzes scenes based on local, global and semantic properties. Deep learning-based saliency prediction models adopted two-stream networks, leveraged prior knowledge of global semantics, or added long-range dependency modeling structures like transformers to incorporate global saliency information. However, they either brought high complexity to learning local and global features or neglected the design for enhancing local features. In this paper, we propose a Global Semantic-Guided Network (GSGNet), which first enriches global semantics through a modified transformer block and then incorporates semantic information into visual features from local and global perspectives in an efficient way. Multi-head self-attention in transformers captures global features, but lacks information communication within and between feature subspaces (heads) when computing the similarity matrix. To learn global representations and enhance interactions of the subspaces, we propose a Channel-Squeeze Spatial Attention (CSSA) module to emphasize channel-relevant information in a compression manner and learn global spatial relationships. To better fuse local and global contextual information, we propose a hybrid CNN-Transformer block called local-global fusion block (LGFB) for aggregating semantic features simply and efficiently. Experimental results on four public datasets demonstrate that our model achieves compelling performance compared with the state-of-the-art saliency prediction models on various evaluation metrics.
更多查看译文
关键词
Saliency prediction,Channel interaction,Semantic information,Feature fusion,Transformer
AI 理解论文
溯源树
样例
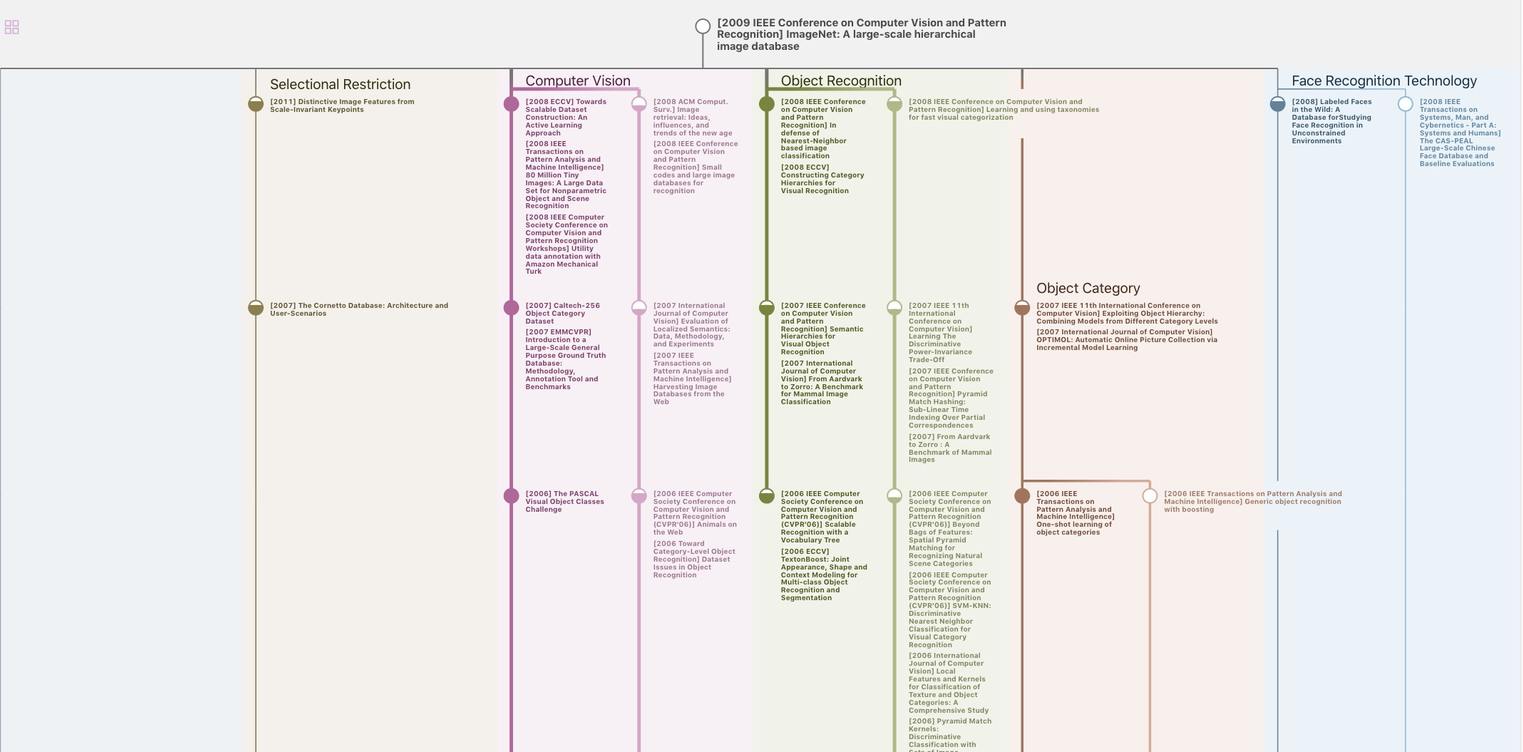
生成溯源树,研究论文发展脉络
Chat Paper
正在生成论文摘要