Two-step affinity matrix learning for multi-view subspace clustering
EXPERT SYSTEMS WITH APPLICATIONS(2024)
摘要
Multi-view subspace clustering aims to learn an appropriate affinity matrix to investigate the relationship between data. However, the learned affinity matrix always has limited representation ability and unclear intrinsic structure. To address the problem, we propose a novel two-step learning strategy to enhance the quality of the affinity matrix. First, we design a generalized framework to calculate the affinity matrix for each view. Unlike most subspace clustering methods that focus on constructing the coefficient matrices, the proposed framework combines the subspace-based and graph-based models to simultaneously learn the coefficient and similarity matrices under a consistency constraint. Thus, the data structure information and multiple views correlation can be captured and propagated between these matrices, which is conducive to generating an informative affinity matrix. Further, we present a post-processing technology called unified good neighbors learning to make the obtained affinity matrix have good sparsity and connectivity. Specifically, we find the good neighbors of each sample by all the views and reassign their coefficients in the final affinity matrix to reflect the essential correlation between samples. Finally, we develop an efficient optimization algorithm and provide the theoretical analyses for the proposed problem. Extensive experiments conducted on six popular multi-view datasets demonstrate the effectiveness of our method. The source code is available at https://github.com/taozhang1223/TAML.
更多查看译文
关键词
Multi-view clustering,Affinity matrix,Subspace-based model,Graph-based model,Good neighbors
AI 理解论文
溯源树
样例
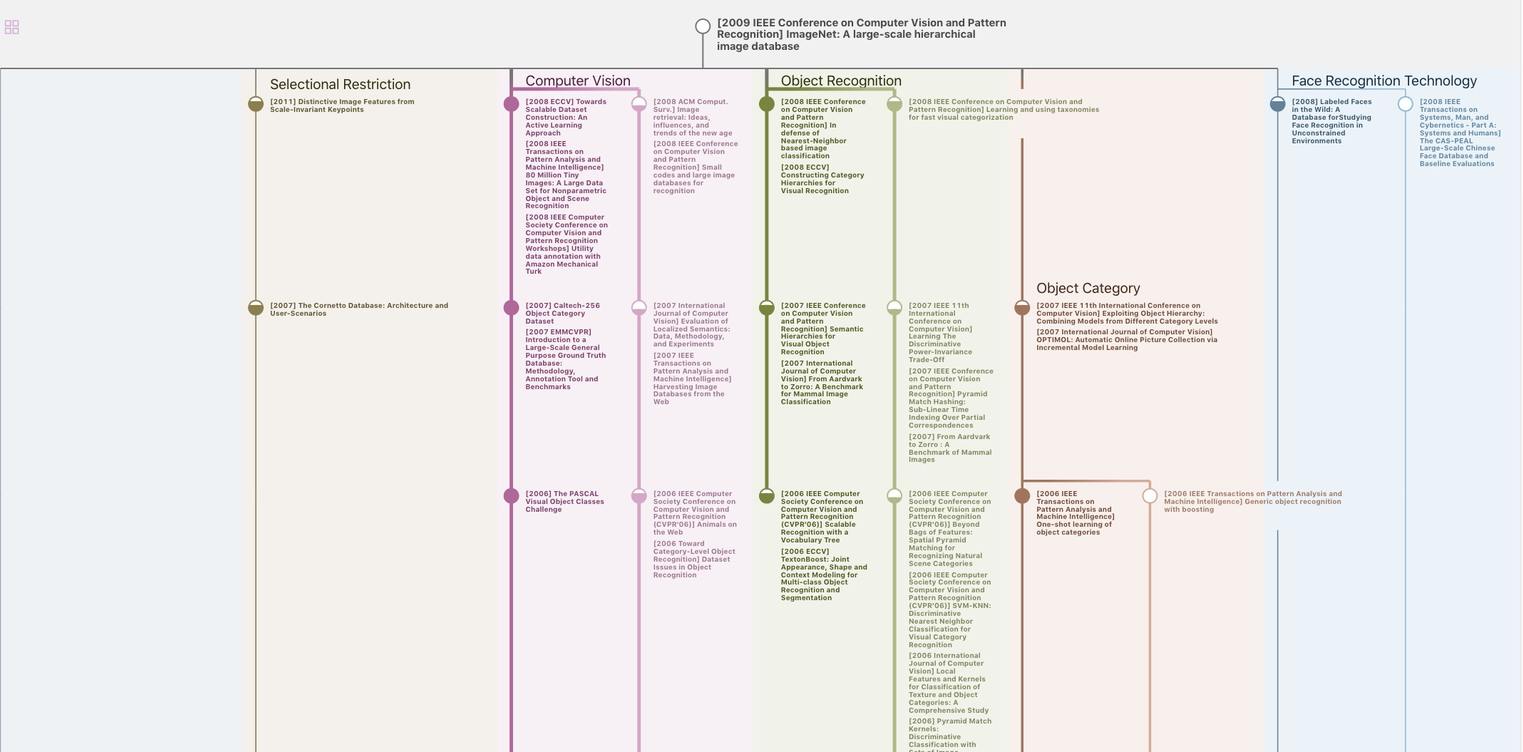
生成溯源树,研究论文发展脉络
Chat Paper
正在生成论文摘要