RUL prediction of rolling bearings across working conditions based on multi-scale convolutional parallel memory domain adaptation network
RELIABILITY ENGINEERING & SYSTEM SAFETY(2024)
摘要
Rolling bearings are widely used in mechanical equipment, effectively determining the failure time of rolling bearings is particularly significant to ensure the safe performance of mechanical equipment. However, in industrial scenarios, the machine mainly works in the normal state for a long time, it is difficult to accumulate the same distribution of the whole life data, but the use of different distribution of data for forecasting will reduce the performance of deep learning-based prediction methods. Therefore, in order to tackle this problem, a multi-scale convolutional parallel memory domain adaptation network is investigated to forecast the residual useful life (RUL) of bearings across working conditions. Firstly, a new characteristic extractor-multi-scale convolutional parallel memory network is designed to extract spatial and temporal characteristics of bearing degradation data. At the same time, in order to minimize the distribution difference between source domain and target domain, a temporal-spatial feature alignment strategy is proposed to obtain domain invariable characteristics by combining maximum mean difference and domain adversarial learning. Finally, the availability of the proposed approach is verified using two rolling bearing data sets. The results reveal that it can efficiently forecast the RUL of rolling bearings across working conditions.
更多查看译文
关键词
Rolling bearing RUL prediction,Multi-scale convolution,Parallel memory network,Across working conditions,Domain adaptation
AI 理解论文
溯源树
样例
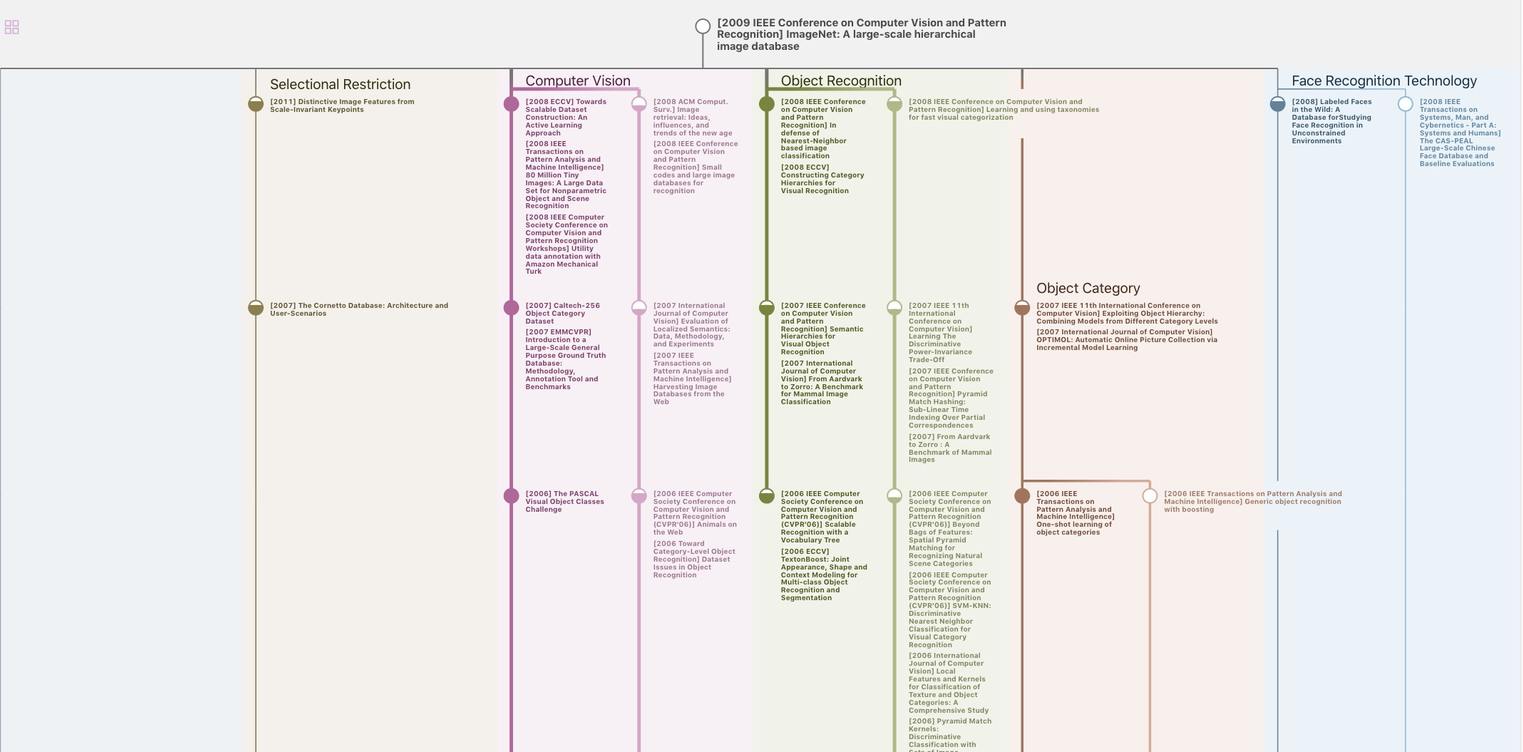
生成溯源树,研究论文发展脉络
Chat Paper
正在生成论文摘要