Interpretable multidisease diagnosis and label noise detection based on a matching network and self-paced learning
PATTERN RECOGNITION(2024)
摘要
With the extensive use of information systems in hospitals, a large quantity of electronic medical record data has been accumulated, which makes it possible to train clinical decision support systems based on the data. However, electronic medical records are written by doctors of different levels, which easily introduces label noise into the datasets. The lack of interpretability of current auxiliary diagnosis methods is another problem. To address these challenges, we introduce a matching network based on medical guidelines and build an auxiliary diagnosis model based on self-paced learning. The matching network based on guidelines can provide medical knowledge beyond medical records and a certain degree of interpretability. Additionally, self-paced learning can help the model identify the label noise and prevent the model from being misled. The experiments show that our method outperforms the baselines in a Chinese medical multi-disease diagnosis dataset and the MIMIC-III dataset and has good performance in the label noise detection task.
更多查看译文
关键词
Multidisease diagnosis,Electronic medical record,Label noise detection,Self-paced learning,Interpretability of deep learning
AI 理解论文
溯源树
样例
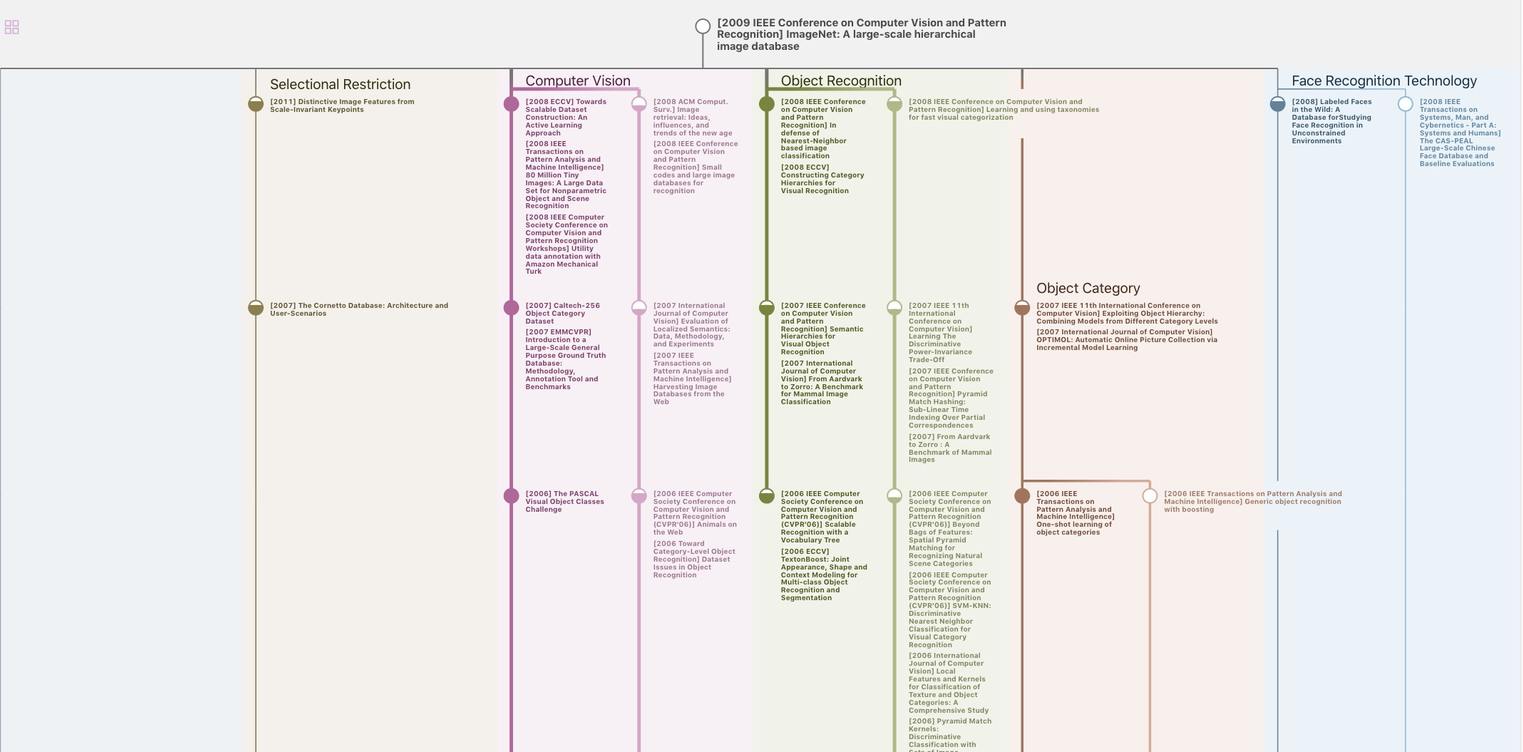
生成溯源树,研究论文发展脉络
Chat Paper
正在生成论文摘要