Improving the prediction of extreme wind speed events with generative data augmentation techniques
RENEWABLE ENERGY(2024)
摘要
Extreme Wind Speed events (EWS) are responsible for the worst damages caused by wind in wind farms. An accurate estimation of the frequency and intensity of EWS is essential to avoid wind turbine damage and to minimize cut-out events in these facilities. In this paper we discuss how generative Data Augmentation (DA) techniques improve the performance of Machine Learning (ML) and Deep Learning (DL) algorithms in EWS prediction problems. These problems are usually tackled as classification tasks, which are highly unbalanced due to the small number of EWS events in wind farms. Different versions of Variational AutoEncoders (VAE) are proposed and analysed in this work (VAEs, Conditional VAEs (CVAEs) and Class-Informed VAEs (CI-VAE)) as generative DA techniques to balance the data in EWS problems, leading to better performance of the prediction systems. The proposed generative DA techniques have been compared against traditional DA algorithms in a real problem of EWS prediction in Spain, considering ERA5 reanalysis data as predictive variables. The results showed that the CI-VAE with a Convolutional Neural Network approach obtained the best results, with values of Precision 0.62, Recall 0.74 and F1 score 0.67, improving up to 4% the results of the method without data augmentation techniques.
更多查看译文
关键词
Extreme wind speed,Prediction systems,Machine learning,Variational autoencoders,Data augmentation techniques
AI 理解论文
溯源树
样例
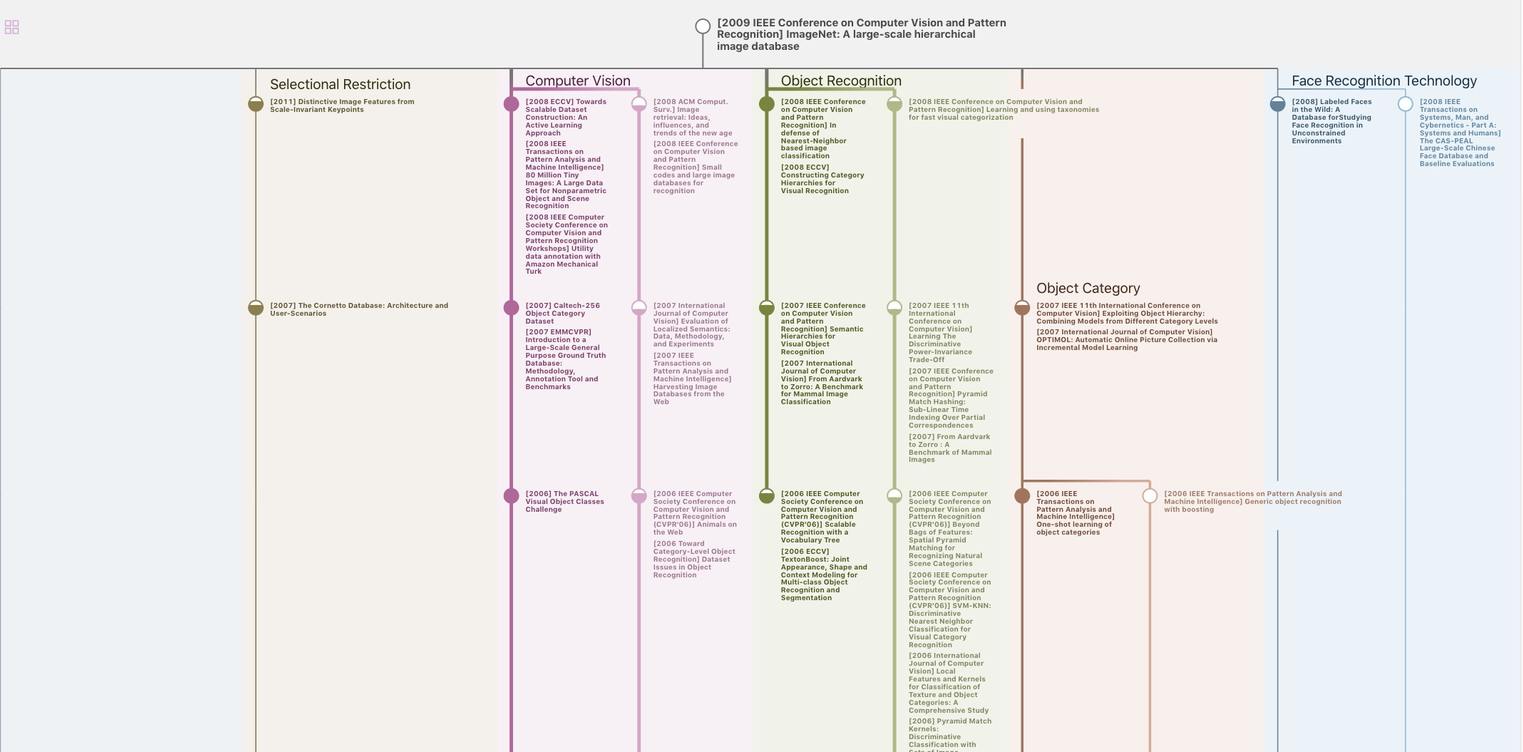
生成溯源树,研究论文发展脉络
Chat Paper
正在生成论文摘要