FastInject: Injecting Unpaired Text Data into CTC-based ASR training
CoRR(2023)
摘要
Recently, connectionist temporal classification (CTC)-based end-to-end (E2E)
automatic speech recognition (ASR) models have achieved impressive results,
especially with the development of self-supervised learning. However, E2E ASR
models trained on paired speech-text data often suffer from domain shifts from
training to testing. To alleviate this issue, this paper proposes a flat-start
joint training method, named FastInject, which efficiently injects multi-domain
unpaired text data into CTC-based ASR training. To maintain training
efficiency, text units are pre-upsampled, and their representations are fed
into the CTC model along with speech features. To bridge the modality gap
between speech and text, an attention-based modality matching mechanism (AM3)
is proposed, which retains the E2E flat-start training. Experiments show that
the proposed FastInject gave a 22\% relative WER reduction (WERR) for
intra-domain Librispeech-100h data and 20\% relative WERR on out-of-domain test
sets.
更多查看译文
关键词
ASR,CTC,text data,domain shifts
AI 理解论文
溯源树
样例
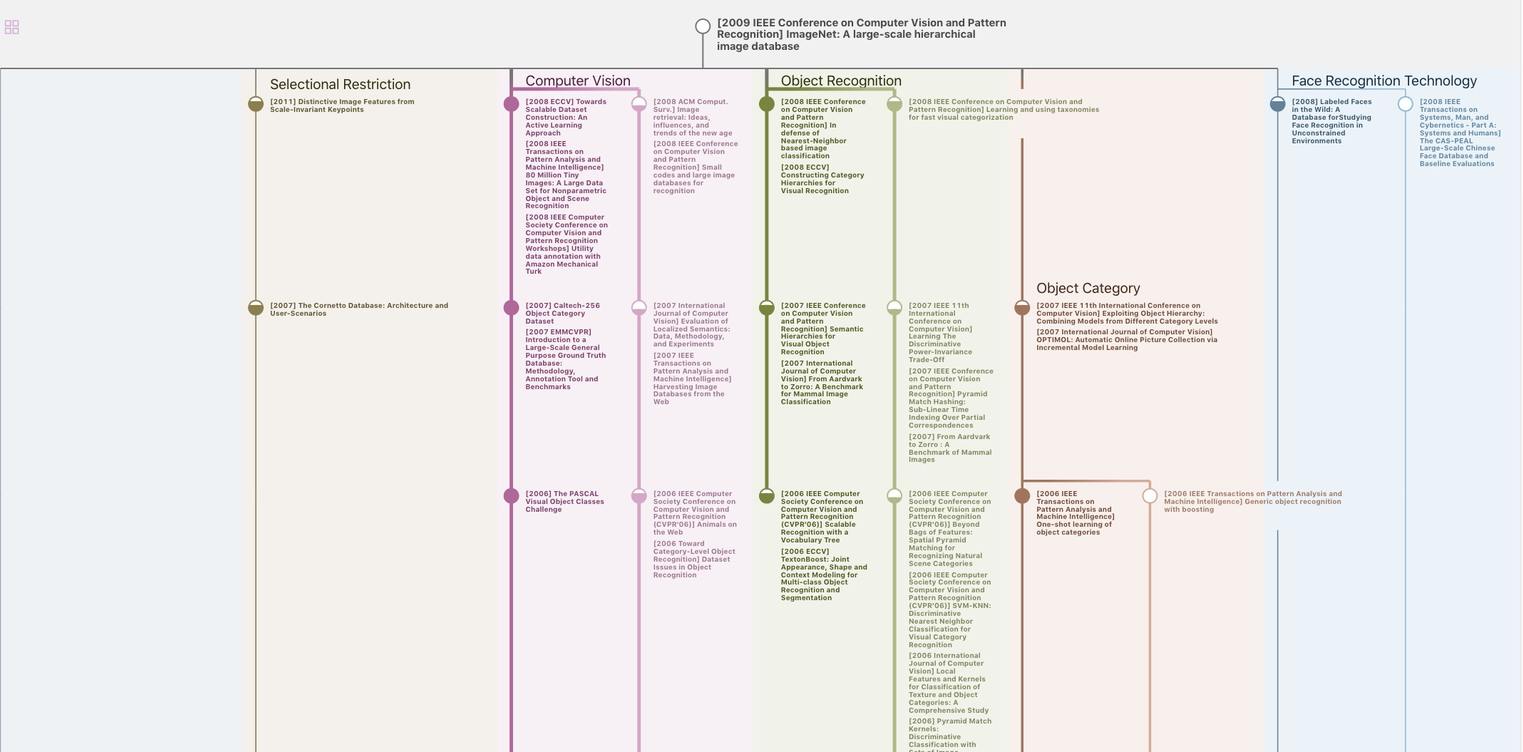
生成溯源树,研究论文发展脉络
Chat Paper
正在生成论文摘要