Training-free Zero-shot Composed Image Retrieval with Local Concept Reranking
CoRR(2023)
摘要
Composed image retrieval attempts to retrieve an image of interest from
gallery images through a composed query of a reference image and its
corresponding modified text. It has recently attracted attention due to the
collaboration of information-rich images and concise language to precisely
express the requirements of target images. Most of the existing composed image
retrieval methods follow a supervised learning paradigm to perform training on
a costly triplet dataset composed of a reference image, modified text, and a
corresponding target image. To alleviate the demand for difficult-to-obtain
labeled triplet data, recent methods have introduced zero-shot composed image
retrieval (ZS-CIR), which aims to retrieve the target image without the
supervision of human-labeled triplets but instead relies on image-text pairs or
self-generated triplets. However, these methods are less computationally
efficient due to the requirement of training and also less understandable,
assuming that the interaction between image and text is conducted with implicit
query embedding. In this work, we present a new Training-Free zero-shot
Composed Image Retrieval (TFCIR) method which translates the query into
explicit human-understandable text. This helps improve computation efficiency
while maintaining the generalization of foundation models. Further, we
introduce a Local Concept Reranking (LCR) mechanism to focus on discriminative
local information extracted from the modified instruction. Extensive
experiments on three ZS-CIR benchmarks show that the proposed approach can
achieve comparable performances with state-of-the-art methods and significantly
outperforms other training-free methods on the open domain datasets, CIRR and
CIRCO, as well as the fashion domain dataset, FashionIQ.
更多查看译文
AI 理解论文
溯源树
样例
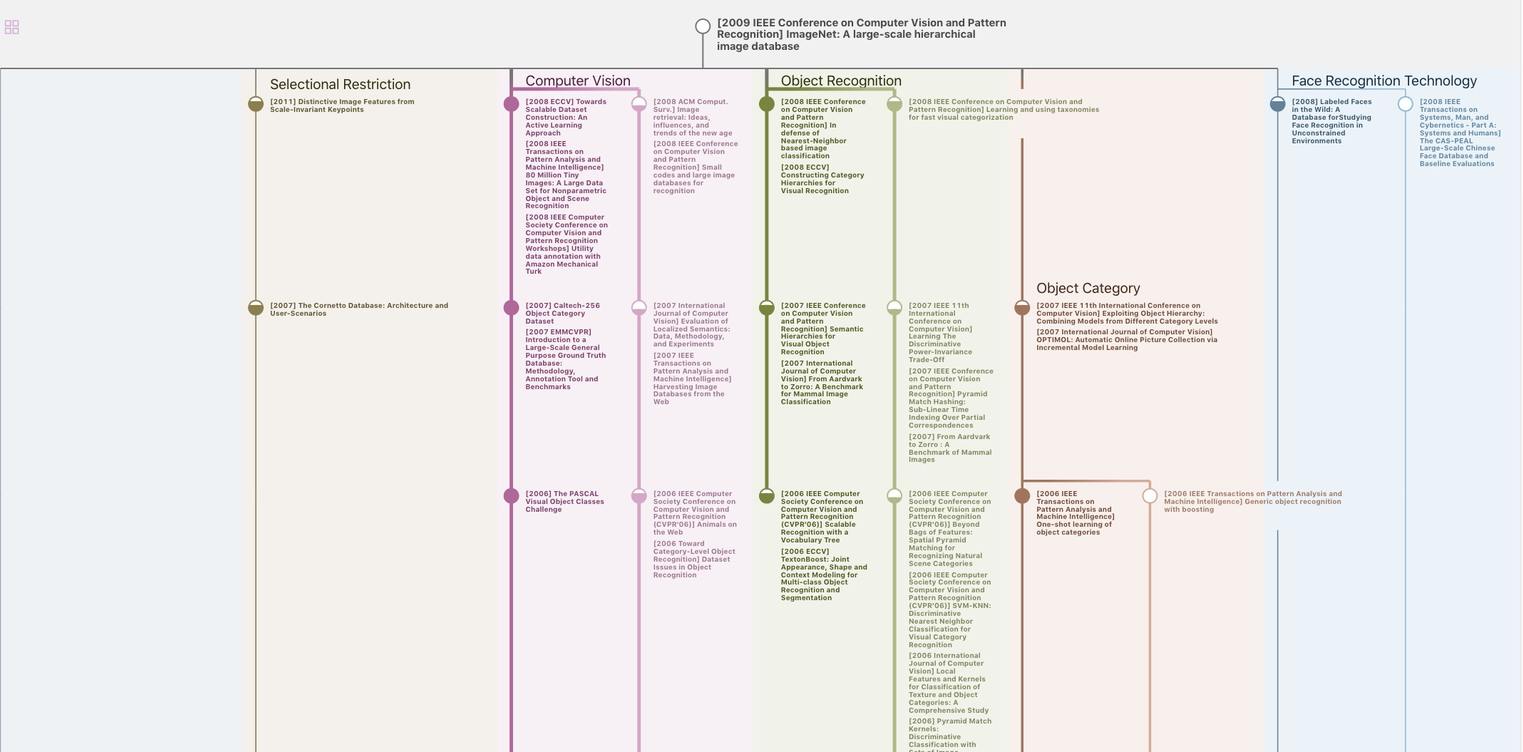
生成溯源树,研究论文发展脉络
Chat Paper
正在生成论文摘要