Uncertainty in GNN Learning Evaluations: A Comparison between Measures for Quantifying Randomness in GNN Community Detection
ENTROPY(2024)
摘要
(1) The enhanced capability of graph neural networks (GNNs) in unsupervised community detection of clustered nodes is attributed to their capacity to encode both the connectivity and feature information spaces of graphs. The identification of latent communities holds practical significance in various domains, from social networks to genomics. Current real-world performance benchmarks are perplexing due to the multitude of decisions influencing GNN evaluations for this task. (2) Three metrics are compared to assess the consistency of algorithm rankings in the presence of randomness. The consistency and quality of performance between the results under a hyperparameter optimisation with the default hyperparameters is evaluated. (3) The results compare hyperparameter optimisation with default hyperparameters, revealing a significant performance loss when neglecting hyperparameter investigation. A comparison of metrics indicates that ties in ranks can substantially alter the quantification of randomness. (4) Ensuring adherence to the same evaluation criteria may result in notable differences in the reported performance of methods for this task. The W randomness coefficient, based on the Wasserstein distance, is identified as providing the most robust assessment of randomness.
更多查看译文
关键词
graph neural networks,community detection,hyperparameter optimisation,node clustering,representation learning,benchmarks
AI 理解论文
溯源树
样例
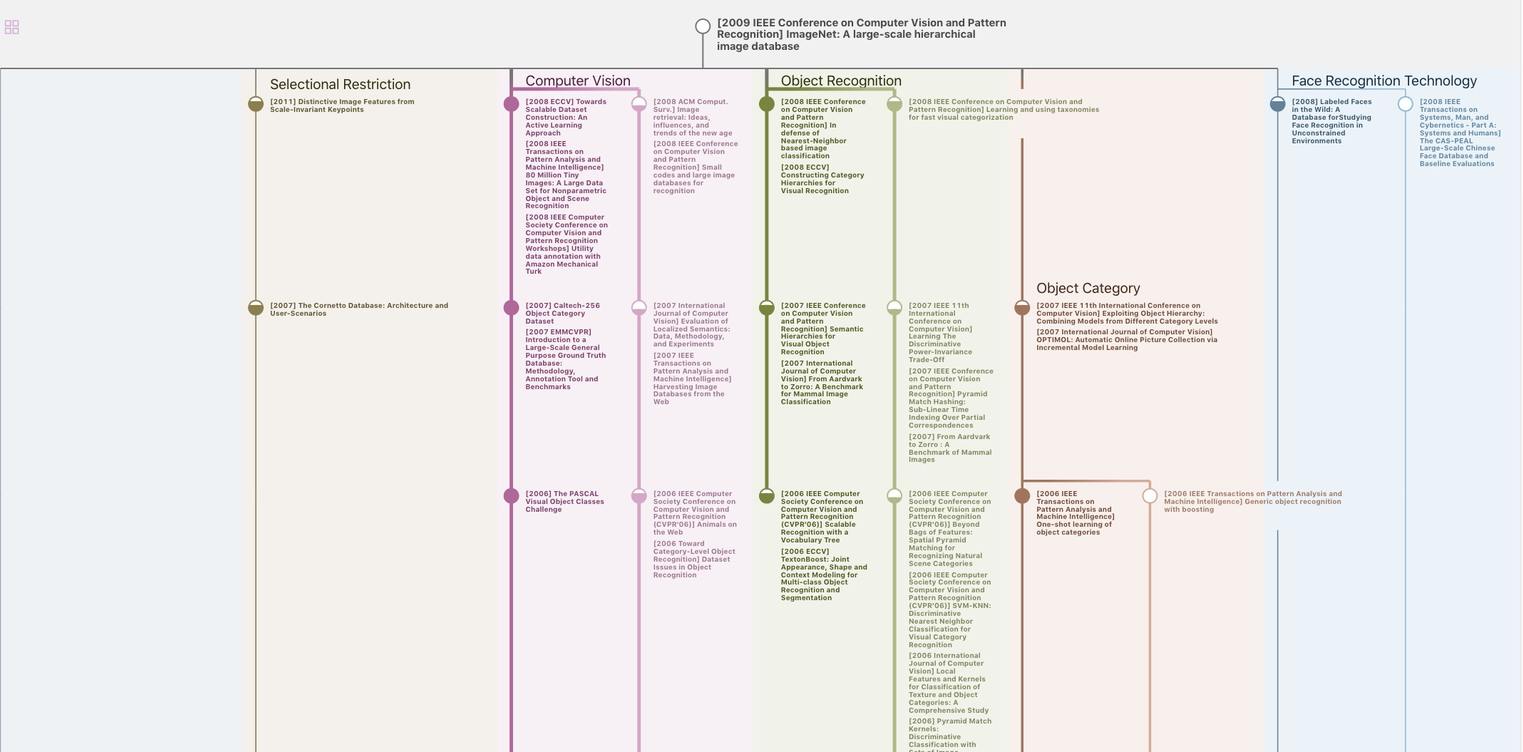
生成溯源树,研究论文发展脉络
Chat Paper
正在生成论文摘要