Integrating Particle Flavor into Deep Learning Models for Hadronization
arxiv(2023)
摘要
Hadronization models used in event generators are physics-inspired functions
with many tunable parameters. Since we do not understand hadronization from
first principles, there have been multiple proposals to improve the accuracy of
hadronization models by utilizing more flexible parameterizations based on
neural networks. These recent proposals have focused on the kinematic
properties of hadrons, but a full model must also include particle flavor. In
this paper, we show how to build a deep learning-based hadronization model that
includes both kinematic (continuous) and flavor (discrete) degrees of freedom.
Our approach is based on Generative Adversarial Networks and we show the
performance within the context of the cluster hadronization model within the
Herwig event generator.
更多查看译文
AI 理解论文
溯源树
样例
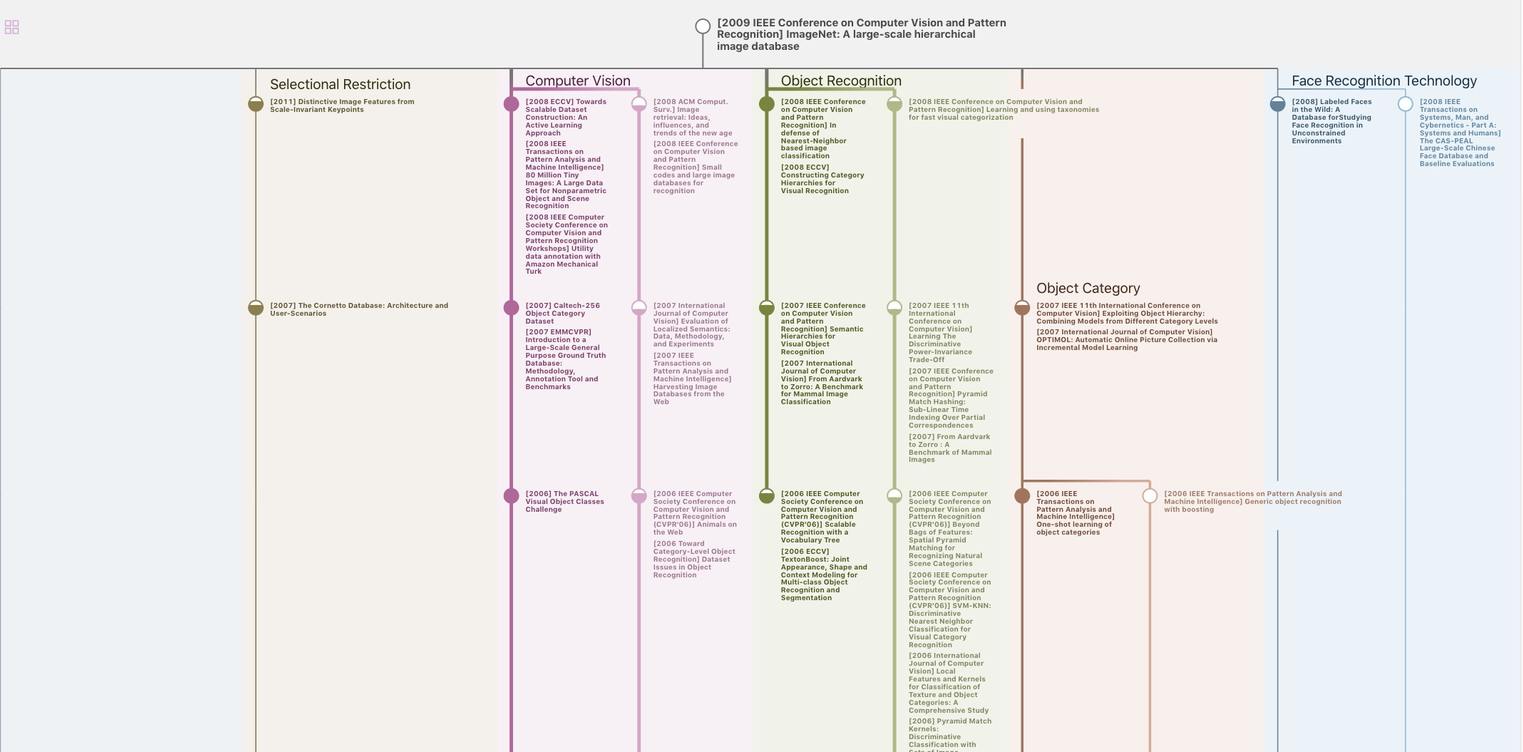
生成溯源树,研究论文发展脉络
Chat Paper
正在生成论文摘要