Fine-tuning protein language models boosts predictions across diverse tasks
biorxiv(2023)
摘要
Prediction methods inputting embeddings from protein Language Models (pLMs) have reached or even surpassed state-of-theart (SOTA) performance on many protein prediction tasks. In natural language processing (NLP) fine-tuning Language Models has become the de facto standard. In contrast, most pLM-based protein predictions do not back-propagate to the pLM. Here, we compared the fine-tuning of three SOTA pLMs (ESM2, ProtT5, Ankh) on eight different tasks. Two results stood out. Firstly, taskspecific supervised fine-tuning almost always improved downstream predictions. Secondly, parameter-efficient fine-tuning could reach similar improvements consuming substantially fewer resources. Put simply: always fine-tune pLMs and you will mostly gain. To help you, we provided easy-to-use notebooks for parameter efficient fine-tuning of ProtT5 for per-protein (pooling) and per-residue prediction tasks at https://github.com/agemagician/ProtTrans/tree/master/Fine-Tuning.
### Competing Interest Statement
The authors have declared no competing interest.
更多查看译文
AI 理解论文
溯源树
样例
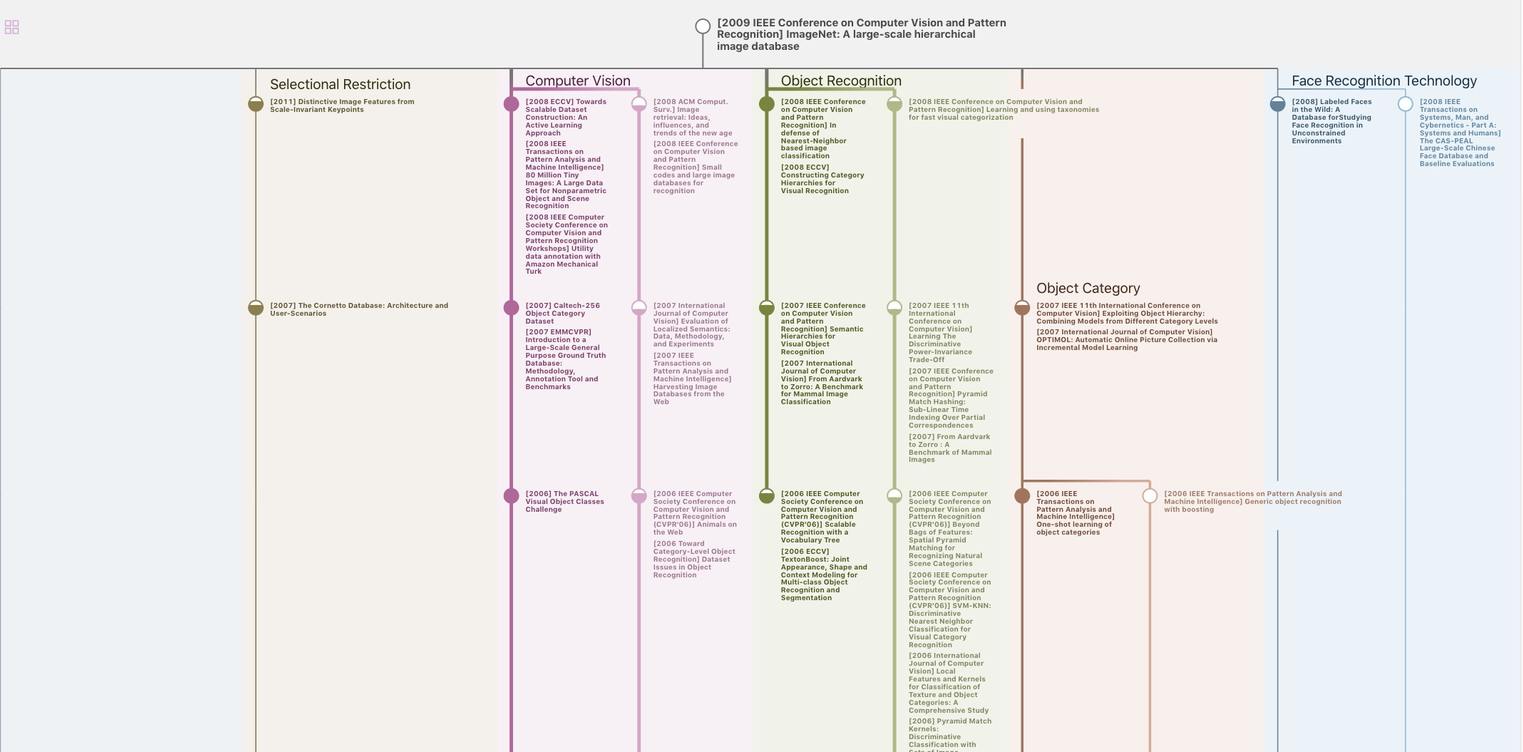
生成溯源树,研究论文发展脉络
Chat Paper
正在生成论文摘要