An efficient model for predicting human diseases through miRNA based on multiple-types of contrastive learning
FRONTIERS IN MICROBIOLOGY(2023)
摘要
Multiple studies have demonstrated that microRNA (miRNA) can be deeply involved in the regulatory mechanism of human microbiota, thereby inducing disease. Developing effective methods to infer potential associations between microRNAs (miRNAs) and diseases can aid early diagnosis and treatment. Recent methods utilize machine learning or deep learning to predict miRNA-disease associations (MDAs), achieving state-of-the-art performance. However, the problem of sparse neighborhoods of nodes due to lack of data has not been well solved. To this end, we propose a new model named MTCL-MDA, which integrates multiple-types of contrastive learning strategies into a graph collaborative filtering model to predict potential MDAs. The model adopts a contrastive learning strategy based on topology, which alleviates the damage to model performance caused by sparse neighborhoods. In addition, the model also adopts a semantic-based contrastive learning strategy, which not only reduces the impact of noise introduced by topology-based contrastive learning, but also enhances the semantic information of nodes. Experimental results show that our model outperforms existing models on all evaluation metrics. Case analysis shows that our model can more accurately identify potential MDA, which is of great significance for the screening and diagnosis of real-life diseases. Our data and code are publicly available at: https://github.com/Lqingquan/MTCL-MDA.
更多查看译文
关键词
contrastive learning,diagnosis and treatment,graph collaborative filtering,human microbiota,miRNA-disease associations,sparse neighborhoods
AI 理解论文
溯源树
样例
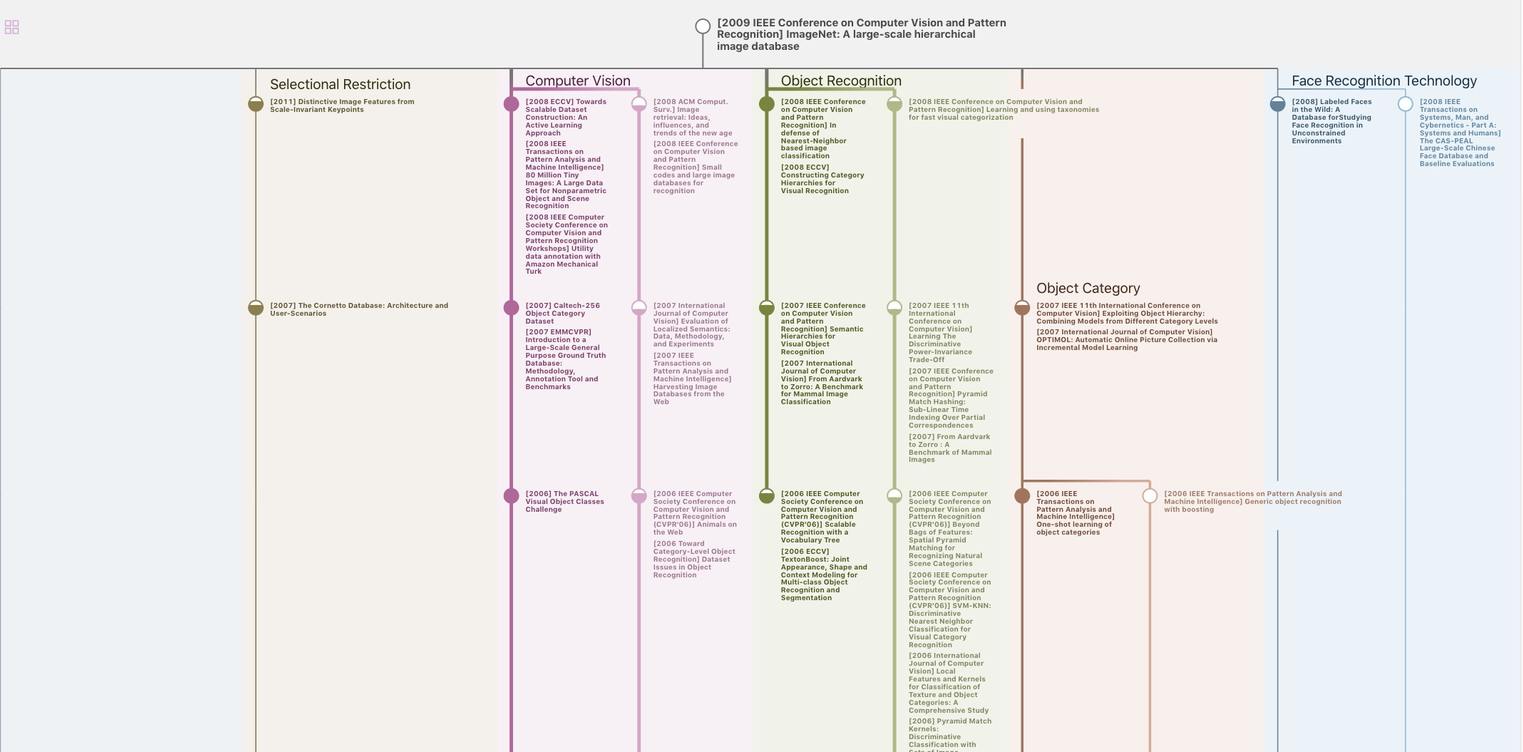
生成溯源树,研究论文发展脉络
Chat Paper
正在生成论文摘要