Event-Triggered Adaptive Neural Control for Full State-Constrained Nonlinear Systems with Unknown Disturbances
Cognitive Computation(2024)
摘要
This paper focuses on the adaptive control issue for a class of uncertain nonlinear systems subject to full state constraints and external disturbance. A novel adaptive nonlinear observer is proposed to compensate for disturbance variables in the transformed system. Combining with radial basis function neural networks (RBFNNs) and nonlinear mapping (NM) mechanism, the constrained system is transformed into an unconstrained form and the system uncertainties are effectively handled. Besides that, an adaptive tracking control approach is formulated by invoking backstepping techniques and the event-sampled scheme is utilized to address the sparsity of resources. The adaptive control problem can be addressed with the proposed algorithm, applying the Lyapunov functions, RBF NNs theory, and inequality techniques. Based on the Lyapunov stability theory, it is proved that the system can never violate the specified state constraints and all the closed-loop signals are semiglobally uniformly ultimately bounded (SGUUB). The validity of the proposed approach is well illustrated by a developed numerical example.
更多查看译文
关键词
Adaptive backstepping control,Disturbance observer,Full state constrains,First-order differentiator,Event-triggered control
AI 理解论文
溯源树
样例
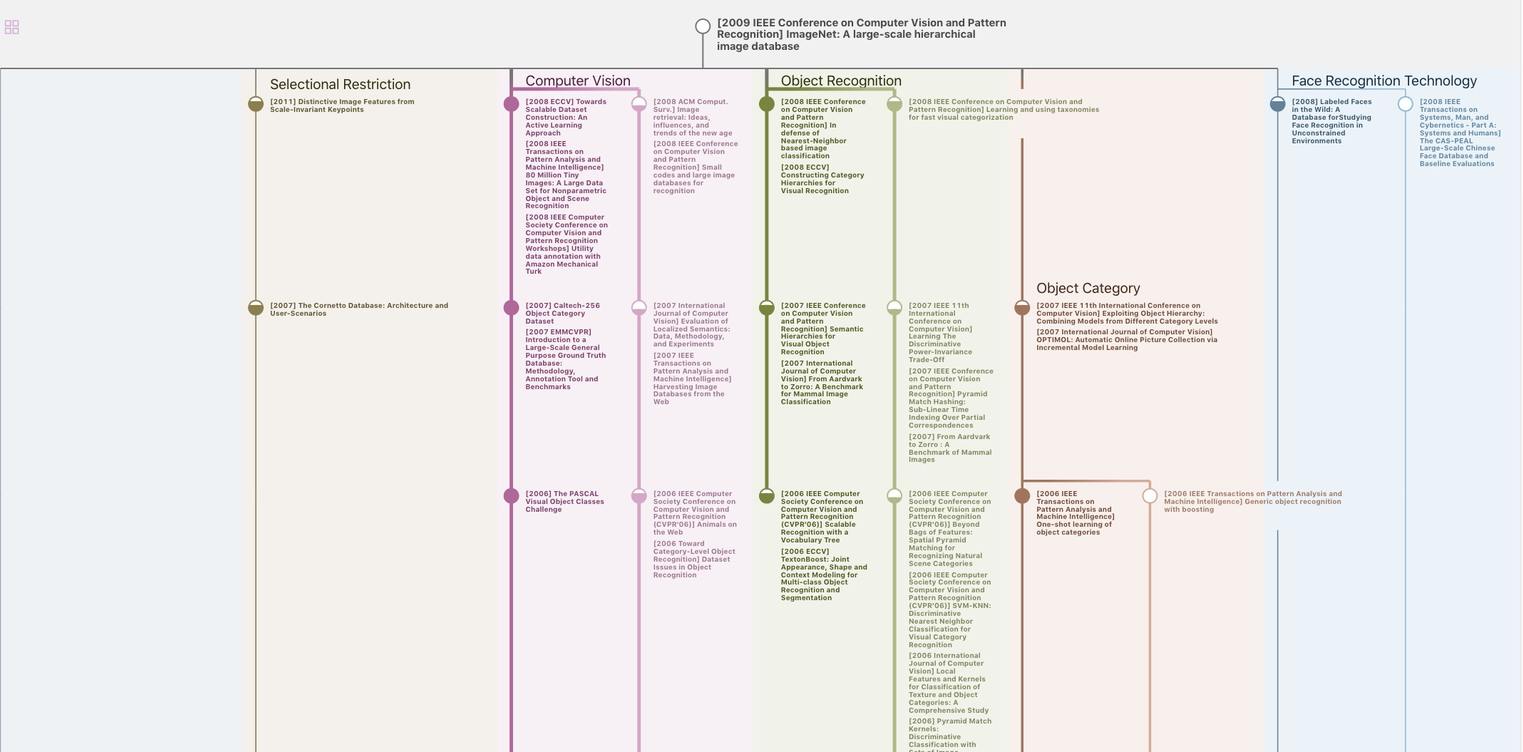
生成溯源树,研究论文发展脉络
Chat Paper
正在生成论文摘要