Advances in Deep Learning Models for Resolving Medical Image Segmentation Data Scarcity Problem: A Topical Review
Archives of Computational Methods in Engineering(2023)
摘要
Deep learning (DL) methods have recently become state-of-the-art in most automated medical image segmentation tasks. Some of the biggest challenges in this field are related to datasets. This paper aims to review the recent developments in deep learning architectures and approaches that aim to resolve dataset-related challenges faced in DL-based medical image segmentation. We have studied architectural developments in deep learning models and their recent applications in medical image segmentation tasks. Popular U-Net-based models are tested for segmentation performance comparison on a Coronavirus disease 2019 (Covid-19) lung infection Computed Tomography segmentation dataset. The comparison results prove the effectiveness of the original U-Net architecture, even in present-day medical image segmentation tasks. To overcome major dataset-related challenges such as labeled data scarcity, high annotation time and cost, distribution shifts, low-quality of images, and generalizability issues; we have studied recent developments in deep learning approaches like active learning, data augmentation, domain adaptation, and self- and semi-supervised learning, that aim to provide innovative solutions for those challenges. With rapid developments in the field, approaches like data augmentation, domain adaptation, and semi-supervised learning have become some of the hot areas of research, aiming for more efficient use of datasets, better segmentation prediction, and model generalizability.
更多查看译文
AI 理解论文
溯源树
样例
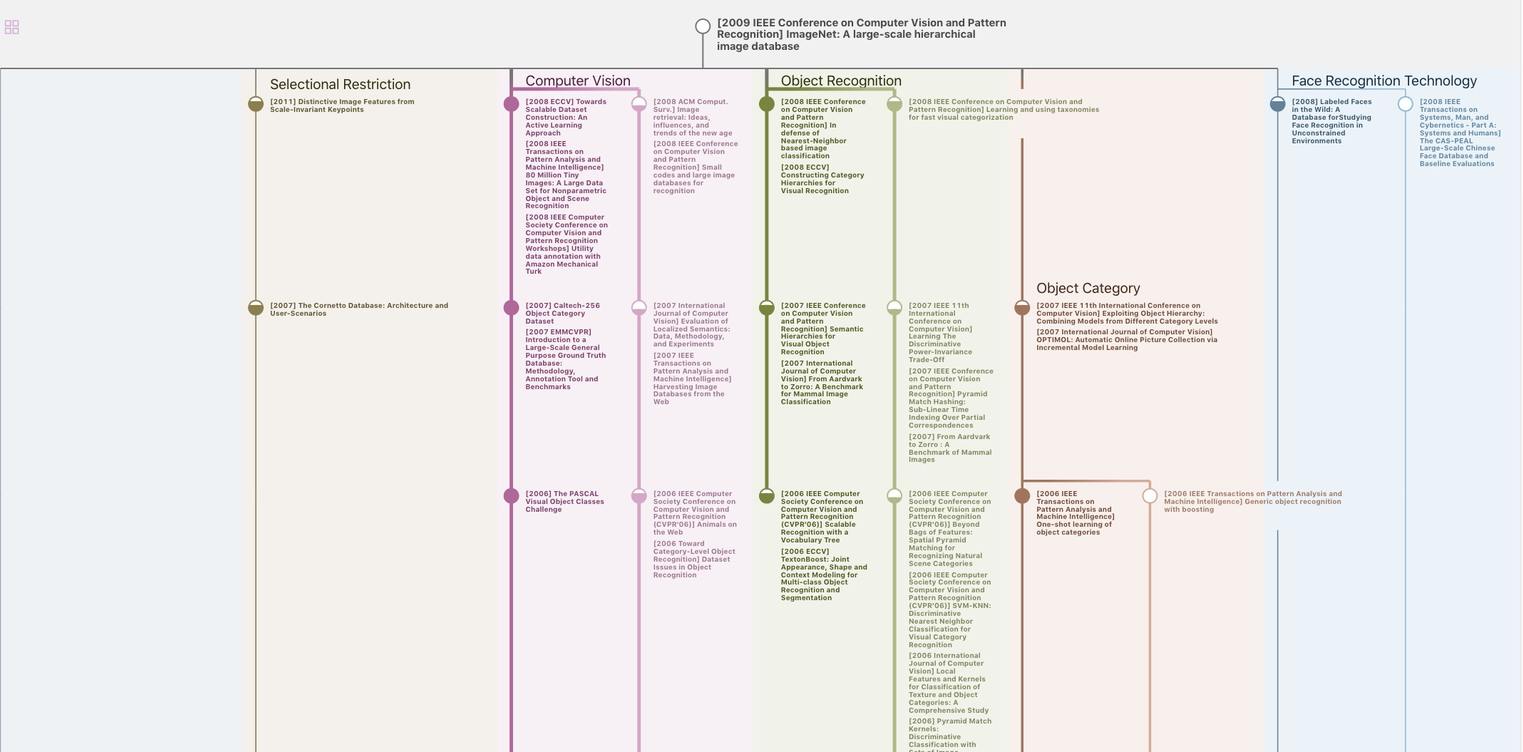
生成溯源树,研究论文发展脉络
Chat Paper
正在生成论文摘要