BCT-OFD: bridging CNN and transformer via online feature distillation for COVID-19 image recognition
International Journal of Machine Learning and Cybernetics(2023)
摘要
Computer-aided systems can assist radiologists in improving the efficiency of COVID-19 diagnosis. Current work adopts complex structures and the correlations between convolutional neural network (CNN) and Transformer haven’t been explored. We propose a novel model named bridging CNN and Transformer via online feature distillation (BCT-OFD). First, lightweight Mpvit-tiny and MobileNetV3-small are chosen as the teacher and student networks, respectively. Sufficient pathological knowledge is smoothly transferred from the teacher to the student using OFD. Then, a adaptive feature fusion module is designed to efficiently fuse the heterogeneous CNN and Transformer features. The implicit correlations between the two networks are fully mined to generate more discriminative fused features. And coordinate attention is adopted to make further feature refinement. The accuracy on three public avaliable datasets reach 97.76%, 98.12% and 96.96%, respectively. It validates that BCT-OFD outperforms state-of-the-art baselines in terms of effectiveness and generalization ability. Notably, BCT-OFD is relatively more lightweight and easier to deploy on those resource-constrained devices, making it the bridge that links theory to application as well as narrowing the gap between them. This study provides an innovative approach in the field of COVID-19 image recognition, offering valuable insights for further improvements in performance.
更多查看译文
关键词
COVID-19 image recognition,Online feature distillation,Feature refinement,Adaptive feature fusion,Coordinate attention
AI 理解论文
溯源树
样例
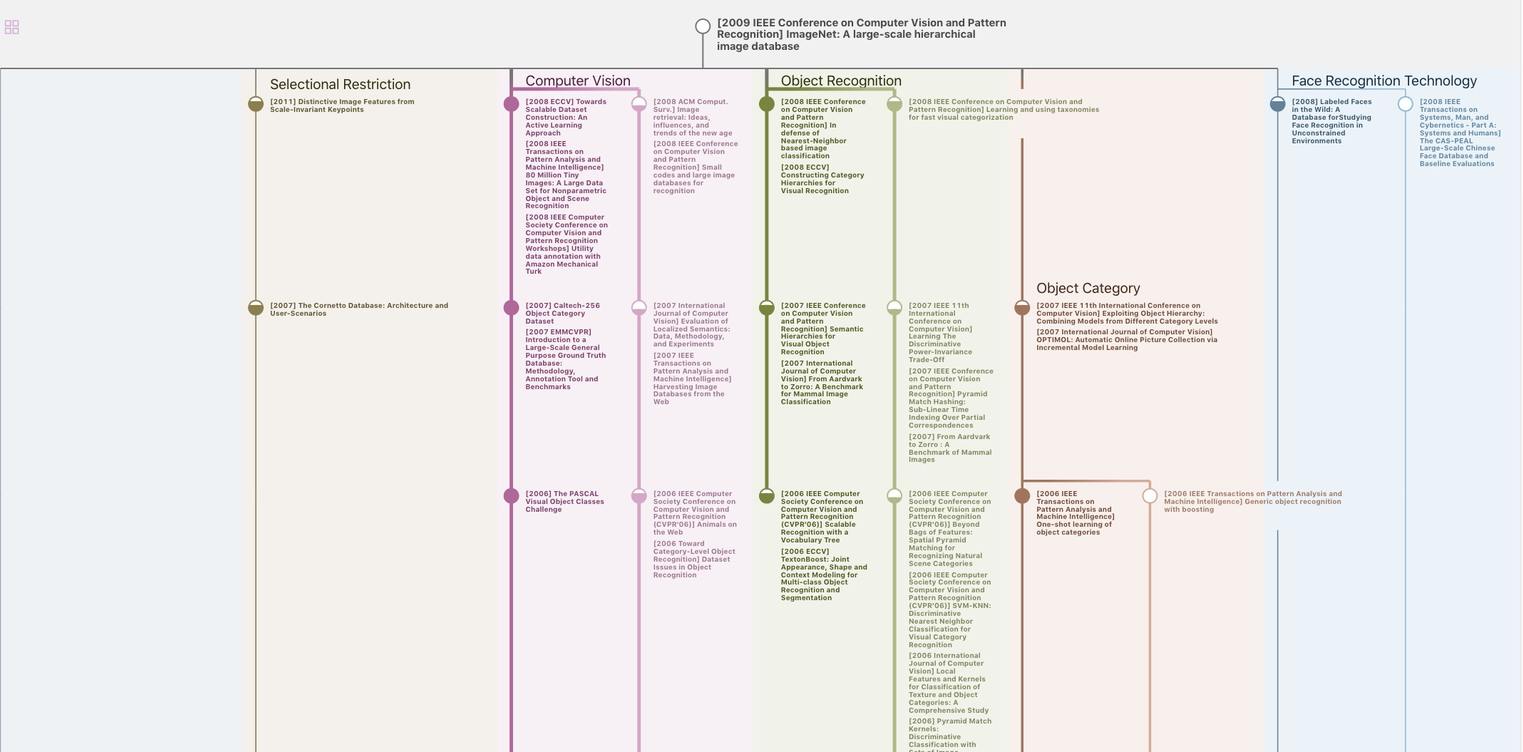
生成溯源树,研究论文发展脉络
Chat Paper
正在生成论文摘要