An Effective and Lightweight Hybrid Network for Object Detection in Remote Sensing Images
IEEE TRANSACTIONS ON GEOSCIENCE AND REMOTE SENSING(2024)
摘要
In recent years, general object detection on nature images leverages convolutional neural networks (CNNs) and vision transformers (ViT) to achieve great progress and high accuracy. However, unlike nature scenarios, remote sensing systems usually deploy a large number of edge devices. Therefore, this condition encourages detectors to have lower parameters than high-complexity neural networks. To this end, we propose an effective and lightweight detection framework of hybrid network, which enhances representation learning to balance efficiency and accuracy of model. Specifically, to compensate low precision caused by lightweight neural networks, we design a boundary-aware context (BAC) module and a frequency self-attention refinement (FSAR) module to improve detector performance in a hybrid structure. The BAC module enhances the local features of the object by fusing the spatial context information with the original image, which not only improves the model accuracy but also effectively solves the problem of multiscale objects. To alleviate the interference of complex background, the FSAR module adopts an adaptive technology to filter out redundant information at different frequencies to improve overall detection performance. The comprehensive experiments on remote sensing datasets, i.e., NWPU VHR-10, LEVIR, and RSOD, indicate that the proposed method achieves state-of-the-art performance and balances between model size and accuracy.
更多查看译文
关键词
Efficient and lightweight,hybrid network,object detection,remote sensing images
AI 理解论文
溯源树
样例
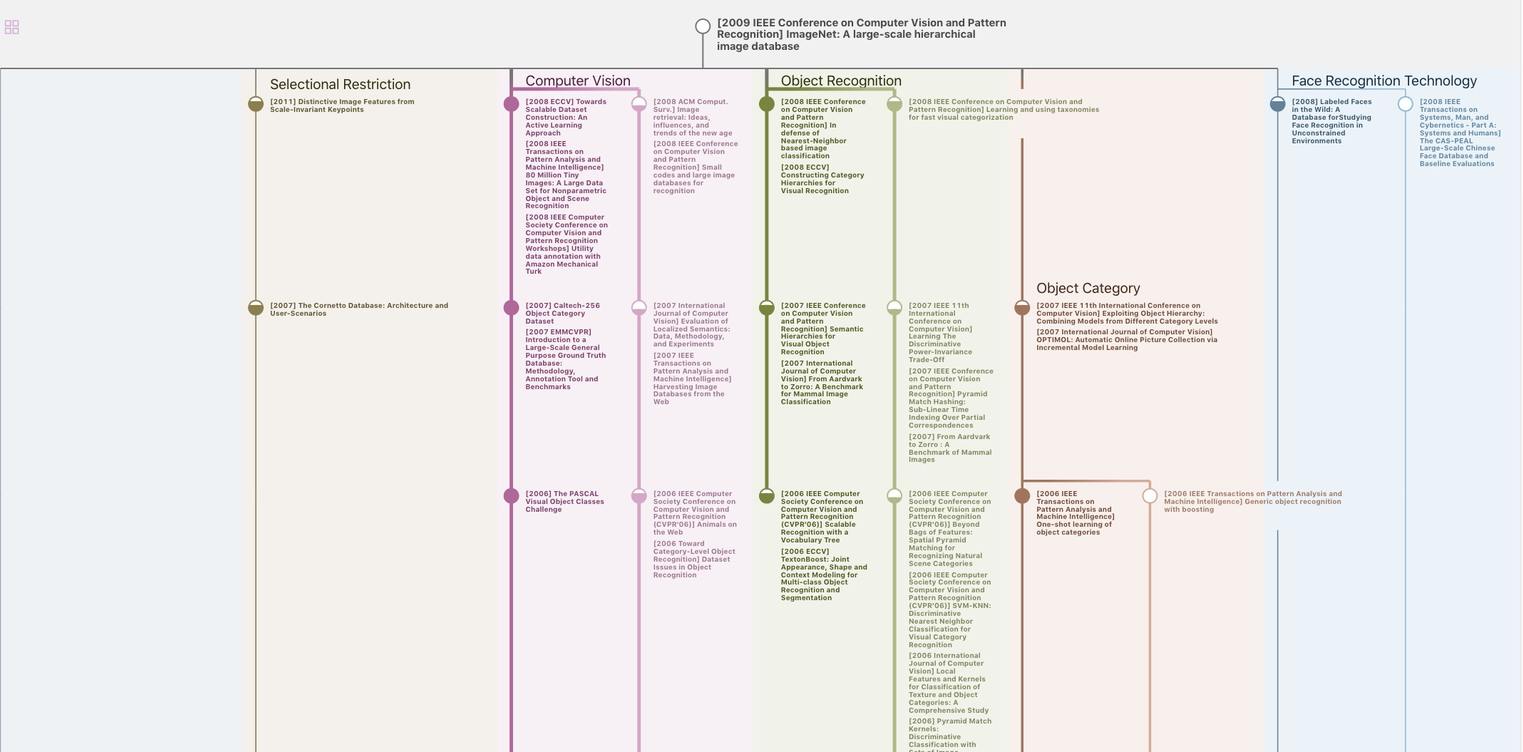
生成溯源树,研究论文发展脉络
Chat Paper
正在生成论文摘要