A Deep Learning Strategy for Multipath Ghosts Filtering via Microwave Tomography
IEEE TRANSACTIONS ON GEOSCIENCE AND REMOTE SENSING(2024)
摘要
Radar imaging algorithms generally exploit linear models of the electromagnetic scattering phenomenon. This assumption leads to qualitative and computationally effective data inversion schemes, which only account for direct scattering from targets, whereas multipath signal contributions are neglected. As a result, multipath ghosts, i.e., false targets reconstructed at positions where no real target exists, affect the radar images, thus preventing a reliable interpretation of the observed scene. This article proposes a fully data-driven deep learning (DL) approach based on a convolutional neural network (CNN) and microwave tomography to face this challenge. The approach achieves multipath ghost suppression for the case of small targets in terms of probing wavelength. In the proposed training scheme, the tomographic image affected by ghosts represents the input of the network while a ghost-free reconstruction is the output. Numerical simulations addressing the detection of metallic rebars via ground penetrating radar (GPR) are presented. As shown, the proposed ghost removal strategy is effective and robust to variations of the scenario parameters on which the network is trained. Finally, an experimental validation shows the effectiveness of the proposed strategy even in operative conditions.
更多查看译文
关键词
Convolutional neural network (CNN),inverse scattering problems,microwave tomography,multipath ghosts,radar imaging
AI 理解论文
溯源树
样例
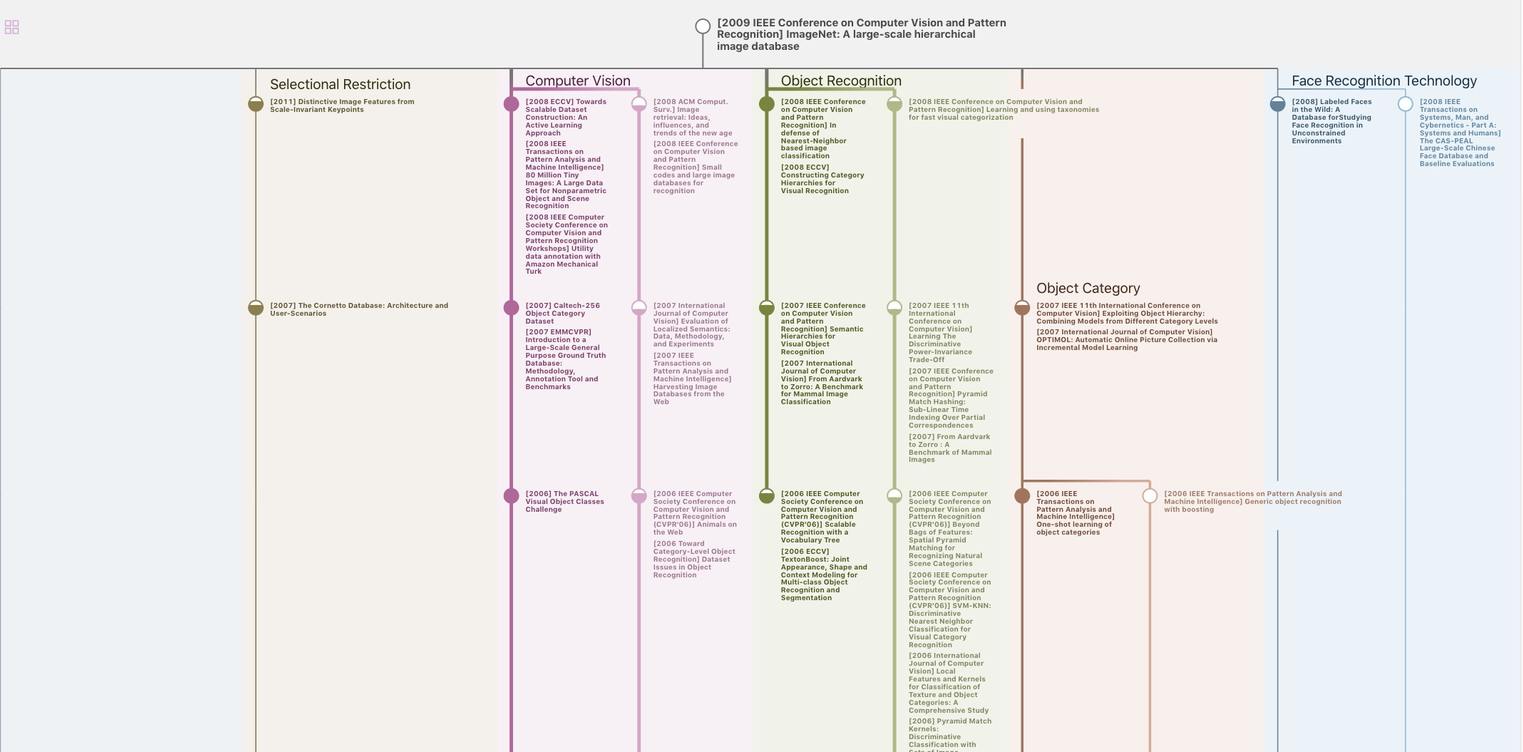
生成溯源树,研究论文发展脉络
Chat Paper
正在生成论文摘要